Multimodal Sentiment Analysis: A Study on Emotion Understanding and Classification by Integrating Text and Images
DOI:
https://doi.org/10.5281/zenodo.13909963ARK:
https://n2t.net/ark:/40704/AJNS.v1n1a08References:
27Keywords:
Multimodal Sentiment Analysis, Text and Image Fusion, Emotion Classification, Deep Learning, BERTAbstract
The advent of social media and the proliferation of multimodal content have led to the growing importance of understanding sentiment in both text and images. Traditional sentiment analysis relies heavily on textual data, but recent trends indicate that integrating visual information can significantly improve sentiment prediction accuracy. This paper explores multimodal sentiment analysis, specifically focusing on emotion understanding and classification by integrating textual and image-based features. We review existing approaches, develop a hybrid deep learning model utilizing attention mechanisms and transformer architectures for multimodal sentiment classification, and evaluate its performance on benchmark datasets, including Twitter and Instagram data. Our findings suggest that multimodal approaches outperform text-only models, especially in more nuanced sentiment cases such as sarcasm, irony, or mixed emotions. Moreover, we address key challenges like feature fusion, domain adaptation, and the contextual alignment of visual and textual information. The results provide insights into optimizing multimodal fusion techniques to enhance real-world application performance.
Downloads
Metrics
References
Hutto, C. J., & Gilbert, E. (2014). VADER: A Parsimonious Rule-Based Model for Sentiment Analysis.
Social Media Text. In Proceedings of the 8th International Conference on Weblogs and Social Media.
You, Q., Luo, J., Jin, H., & Yang, J. (2015). Robust Image Sentiment Analysis Using Progressively Trained and Domain Transferred Deep Networks. In Proceedings of the AAAI Conference on Artificial Intelligence.
Devlin, J., Chang, M. W., Lee, K., & Toutanova, K. (2019). BERT: Pre-training of Deep Bidirectional Transformers for Language Understanding. In Proceedings of the 2019 Conference of the North American Chapter of the Association for Computational Linguistics.
Zadeh, A., Chen, M., Poria, S., Cambria, E., & Morency, L.-P. (2016). Multimodal Sentiment Analysis with Word-Level Fusion. In Proceedings of the 2016 Conference on Empirical Methods in Natural Language Processing.
Poria, S., Cambria, E., Bajpai, R., & Hussain, A. (2017). A Review of Affective Computing: From Unimodal Analysis to Multimodal Fusion. Information Fusion, 37, 98-125.
Li, W. (2024). The Impact of Apple’s Digital Design on Its Success: An Analysis of Interaction and Interface Design. Academic Journal of Sociology and Management, 2(4), 14–19.
Chen, Q., & Wang, L. (2024). Social Response and Management of Cybersecurity Incidents. Academic Journal of Sociology and Management, 2(4), 49–56.
Song, C. (2024). Optimizing Management Strategies for Enhanced Performance and Energy Efficiency in Modern Computing Systems. Academic Journal of Sociology and Management, 2(4), 57–64.
Chen, Q., Li, D., & Wang, L. (2024). Blockchain Technology for Enhancing Network Security. Journal of Industrial Engineering and Applied Science, 2(4), 22–28.
Chen, Q., Li, D., & Wang, L. (2024). The Role of Artificial Intelligence in Predicting and Preventing Cyber Attacks. Journal of Industrial Engineering and Applied Science, 2(4), 29–35.
Chen, Q., Li, D., & Wang, L. (2024). Network Security in the Internet of Things (IoT) Era. Journal of Industrial Engineering and Applied Science, 2(4), 36–41.
Li, D., Chen, Q., & Wang, L. (2024). Cloud Security: Challenges and Solutions. Journal of Industrial Engineering and Applied Science, 2(4), 42–47.
Baltrusaitis, T., Ahuja, C., & Morency, L.-P. (2018). Multimodal machine learning: A survey and taxonomies. *IEEE Transactions on Pattern Analysis and Machine Intelligence*, 41(2), 423-443. https://doi.org/10.1109/TPAMI.2018.2798607
Chen, X., & Zhang, Z. (2020). A multimodal approach to sentiment analysis based on deep learning. *Journal of Visual Communication and Image Representation*, 66, 102693. https://doi.org/10.1016/j.jvcir.2019.102693
Poria, S., Hu, X., Kumar, A., & Gelbukh, A. (2017). A multimodal approach for sentiment analysis of social media. *Proceedings of the 2017 IEEE International Conference on Data Mining Workshops*, 25-32. https://doi.org/10.1109/ICDMW.2017.18
Li, D., Chen, Q., & Wang, L. (2024). Phishing Attacks: Detection and Prevention Techniques. Journal of Industrial Engineering and Applied Science, 2(4), 48–53.
Song, C., Zhao, G., & Wu, B. (2024). Applications of Low-Power Design in Semiconductor Chips. Journal of Industrial Engineering and Applied Science, 2(4), 54–59.
Zhao, G., Song, C., & Wu, B. (2024). 3D Integrated Circuit (3D IC) Technology and Its Applications. Journal of Industrial Engineering and Applied Science, 2(4), 60–65.
Wu, B., Song, C., & Zhao, G. (2024). Applications of Heterogeneous Integration Technology in Chip Design. Journal of Industrial Engineering and Applied Science, 2(4), 66–72.
Song, C., Wu, B., & Zhao, G. (2024). Optimization of Semiconductor Chip Design Using Artificial Intelligence. Journal of Industrial Engineering and Applied Science, 2(4), 73–80.
Song, C., Wu, B., & Zhao, G. (2024). Applications of Novel Semiconductor Materials in Chip Design. Journal of Industrial Engineering and Applied Science, 2(4), 81–89.
Li, W. (2024). Transforming Logistics with Innovative Interaction Design and Digital UX Solutions. Journal of Computer Technology and Applied Mathematics, 1(3), 91-96.
Li, W. (2024). User-Centered Design for Diversity: Human-Computer Interaction (HCI) Approaches to Serve Vulnerable Communities. Journal of Computer Technology and Applied Mathematics, 1(3), 85-90.
4. You, Q., Jin, H., Yang, Y., & Xu, S. (2015). Image sentiment analysis based on deep learning. *Proceedings of the 23rd ACM International Conference on Multimedia*, 989-992. https://doi.org/10.1145/2733373.2806346
5. Zadeh, A., Chen, M., Poria, S., & Morency, L.-P. (2018). Multimodal language analysis in the wild: CMU-Multimodal Opinion Sentiment and Emotion Intensity (CMU-MOSEI) dataset. *Proceedings of the 2018 Conference on Empirical Methods in Natural Language Processing*, 510-520. https://doi.org/10.18653/v1/D18-1050
6. Zhang, L., & Liu, B. (2018). Sentiment analysis and opinion mining: A survey. *Wiley Interdisciplinary Reviews: Data Mining and Knowledge Discovery*, 8(4), e1263. https://doi.org/10.1002/widm.1263
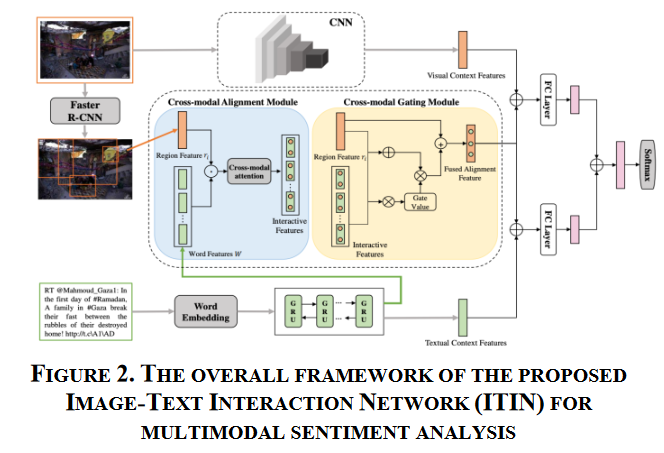
Downloads
Published
How to Cite
Issue
Section
ARK
License
Copyright (c) 2024 The author retains copyright and grants the journal the right of first publication.

This work is licensed under a Creative Commons Attribution 4.0 International License.