Cross-modal Contrastive Learning for Robust Visual Representation in Dynamic Environmental Conditions
DOI:
https://doi.org/10.70393/616a6e73.323833ARK:
https://n2t.net/ark:/40704/AJNS.v2n2a04Disciplines:
Information ScienceSubjects:
Information RetrievalReferences:
33Keywords:
Cross-modal Contrastive Learning, Environmental Robustness, Feature Alignment, Visual RepresentationAbstract
This paper proposes a novel cross-modal contrastive learning framework for robust visual representation under dynamic environmental conditions. We address the challenge of maintaining consistent performance across varying environments by introducing a dual-stream architecture that leverages complementary information from visual and contextual modalities. Our framework incorporates three key components: (1) a cross-modal contrastive learning mechanism that establishes correspondences between modalities while preserving their semantic structure, (2) a feature alignment module with cross-modal attention that dynamically aligns features across modalities, and (3) an environmental adaptation strategy with adaptive normalization and memory-augmented learning to enhance robustness against environmental variations. Extensive experiments on three datasets (DynamicVQA, MultiEnv-ImageText, and RobustSceneX) demonstrate that our approach consistently outperforms existing methods, achieving an average improvement of 8.1% in mean Average Precision over state-of-the-art baselines. Ablation studies confirm the contribution of each component, with the full model exhibiting superior performance in cross-condition scenarios. Zero-shot transfer experiments further validate the generalizability of our learned representations to downstream tasks. Our work provides a comprehensive solution for robust visual representation learning in real-world applications where environmental conditions frequently change.
Downloads
Metrics
References
[1] Song G, Zhang W, Wang B. Deep cross-modal hashing with contrast learning and feature fusion. In2023 International Conference on Image Processing, Computer Vision and Machine Learning (ICICML) 2023 Nov 3 (pp. 638-642). IEEE.
[2] Chhipa PC, Chopra M, Mengi G, Gupta V, Upadhyay R, Chippa MS, De K, Saini R, Uchida S, Liwicki M. Functional knowledge transfer with self-supervised representation learning. In2023 IEEE International Conference on Image Processing (ICIP) 2023 Oct 8 (pp. 3339-3343). IEEE.
[3] Higa K, Yamaguchi M, Hosoi T. ICCL: Self-Supervised Intra-and Cross-Modal Contrastive Learning with 2D-3D Pairs for 3D Scene Understanding. In2023 IEEE International Conference on Image Processing (ICIP) 2023 Oct 8 (pp. 1085-1089). IEEE.
[4] Li R, Weng Z, Chen Y, Zhuang H, Tan YP, Lin Z. Joint-Neighborhood Product Quantization for Unsupervised Cross-Modal Retrieval. In2024 IEEE International Conference on Visual Communications and Image Processing (VCIP) 2024 Dec 8 (pp. 1-5). IEEE.
[5] Ohtomo K, Kitahara Y, Harakawa R, Nakamura A, Shida Y, Ogasawara W, Iwahashi M. Micro-spatial attention with sparse constraint for self-supervised learning for oleaginous yeast image representation. In2023 IEEE International Conference on Visual Communications and Image Processing (VCIP) 2023 Dec 4 (pp. 1-5). IEEE.
[6] Chen, C., Zhang, Z., & Lian, H. (2025). A Low-Complexity Joint Angle Estimation Algorithm for Weather Radar Echo Signals Based on Modified ESPRIT. Journal of Industrial Engineering and Applied Science, 3(2), 33-43.
[7] Xu, K., & Purkayastha, B. (2024). Integrating Artificial Intelligence with KMV Models for Comprehensive Credit Risk Assessment. Academic Journal of Sociology and Management, 2(6), 19-24.
[8] Xu, K., & Purkayastha, B. (2024). Enhancing Stock Price Prediction through Attention-BiLSTM and Investor Sentiment Analysis. Academic Journal of Sociology and Management, 2(6), 14-18.
[9] Shu, M., Liang, J., & Zhu, C. (2024). Automated Risk Factor Extraction from Unstructured Loan Documents: An NLP Approach to Credit Default Prediction. Artificial Intelligence and Machine Learning Review, 5(2), 10-24.
[10] Shu, M., Wang, Z., & Liang, J. (2024). Early Warning Indicators for Financial Market Anomalies: A Multi-Signal Integration Approach. Journal of Advanced Computing Systems, 4(9), 68-84.
[11] Liu, Y., Bi, W., & Fan, J. (2025). Semantic Network Analysis of Financial Regulatory Documents: Extracting Early Risk Warning Signals. Academic Journal of Sociology and Management, 3(2), 22-32.
[12] Zhang, Y., Fan, J., & Dong, B. (2025). Deep Learning-Based Analysis of Social Media Sentiment Impact on Cryptocurrency Market Microstructure. Academic Journal of Sociology and Management, 3(2), 13-21.
[13] Zhou, Z., Xi, Y., Xing, S., & Chen, Y. (2024). Cultural Bias Mitigation in Vision-Language Models for Digital Heritage Documentation: A Comparative Analysis of Debiasing Techniques. Artificial Intelligence and Machine Learning Review, 5(3), 28-40.
[14] Zhang, Y., Zhang, H., & Feng, E. (2024). Cost-Effective Data Lifecycle Management Strategies for Big Data in Hybrid Cloud Environments. Academia Nexus Journal, 3(2).
[15] Wu, Z., Feng, E., & Zhang, Z. (2024). Temporal-Contextual Behavioral Analytics for Proactive Cloud Security Threat Detection. Academia Nexus Journal, 3(2).
[16] Ji, Z., Hu, C., Jia, X., & Chen, Y. (2024). Research on Dynamic Optimization Strategy for Cross-platform Video Transmission Quality Based on Deep Learning. Artificial Intelligence and Machine Learning Review, 5(4), 69-82.
[17] Zhang, K., Xing, S., & Chen, Y. (2024). Research on Cross-Platform Digital Advertising User Behavior Analysis Framework Based on Federated Learning. Artificial Intelligence and Machine Learning Review, 5(3), 41-54.
[18] Xiao, X., Zhang, Y., Chen, H., Ren, W., Zhang, J., & Xu, J. (2025). A Differential Privacy-Based Mechanism for Preventing Data Leakage in Large Language Model Training. Academic Journal of Sociology and Management, 3(2), 33-42.
[19] Xiao, X., Chen, H., Zhang, Y., Ren, W., Xu, J., & Zhang, J. (2025). Anomalous Payment Behavior Detection and Risk Prediction for SMEs Based on LSTM-Attention Mechanism. Academic Journal of Sociology and Management, 3(2), 43-51.
[20] Liu, Y., Feng, E., & Xing, S. (2024). Dark Pool Information Leakage Detection through Natural Language Processing of Trader Communications. Journal of Advanced Computing Systems, 4(11), 42-55.
[21] Chen, Y., Zhang, Y., & Jia, X. (2024). Efficient Visual Content Analysis for Social Media Advertising Performance Assessment. Spectrum of Research, 4(2).
[22] Wu, Z., Wang, S., Ni, C., & Wu, J. (2024). Adaptive Traffic Signal Timing Optimization Using Deep Reinforcement Learning in Urban Networks. Artificial Intelligence and Machine Learning Review, 5(4), 55-68.
[23] Chen, J., & Zhang, Y. (2024). Deep Learning-Based Automated Bug Localization and Analysis in Chip Functional Verification. Annals of Applied Sciences, 5(1).
[24] Zhang, Y., Jia, G., & Fan, J. (2024). Transformer-Based Anomaly Detection in High-Frequency Trading Data: A Time-Sensitive Feature Extraction Approach. Annals of Applied Sciences, 5(1).
[25] Zhang, H., Koh, J. Y., Baldridge, J., Lee, H., & Yang, Y. (2021). Cross-modal contrastive learning for text-to-image generation. In Proceedings of the IEEE/CVF conference on computer vision and pattern recognition (pp. 833-842).
[26] Zolfaghari, M., Zhu, Y., Gehler, P., & Brox, T. (2021). Crossclr: Cross-modal contrastive learning for multi-modal video representations. In Proceedings of the IEEE/CVF international conference on computer vision (pp. 1450-1459).
[27] Li, W., Gao, C., Niu, G., Xiao, X., Liu, H., Liu, J., ... & Wang, H. (2020). Unimo: Towards unified-modal understanding and generation via cross-modal contrastive learning. arXiv preprint arXiv:2012.15409.
[28] Afham, M., Dissanayake, I., Dissanayake, D., Dharmasiri, A., Thilakarathna, K., & Rodrigo, R. (2022). Crosspoint: Self-supervised cross-modal contrastive learning for 3d point cloud understanding. In Proceedings of the IEEE/CVF conference on computer vision and pattern recognition (pp. 9902-9912).
[29] Wu, Y., Liu, J., Gong, M., Gong, P., Fan, X., Qin, A. K., ... & Ma, W. (2023). Self-supervised intra-modal and cross-modal contrastive learning for point cloud understanding. IEEE Transactions on Multimedia, 26, 1626-1638.
[30] Kim, D., Tsai, Y. H., Zhuang, B., Yu, X., Sclaroff, S., Saenko, K., & Chandraker, M. (2021). Learning cross-modal contrastive features for video domain adaptation. In Proceedings of the IEEE/CVF International Conference on Computer Vision (pp. 13618-13627).
[31] Wang, L., Zhang, C., Xu, H., Xu, Y., Xu, X., & Wang, S. (2023, October). Cross-modal contrastive learning for multimodal fake news detection. In Proceedings of the 31st ACM international conference on multimedia (pp. 5696-5704).
[32] Yan, L., Weng, J., & Ma, D. (2025). Enhanced TransFormer-Based Algorithm for Key-Frame Action Recognition in Basketball Shooting.
[33] Wang, Y., Wan, W., Zhang, H., Chen, C., & Jia, G. (2025). Pedestrian Trajectory Intention Prediction in Autonomous Driving Scenarios Based on Spatio-temporal Attention Mechanism.
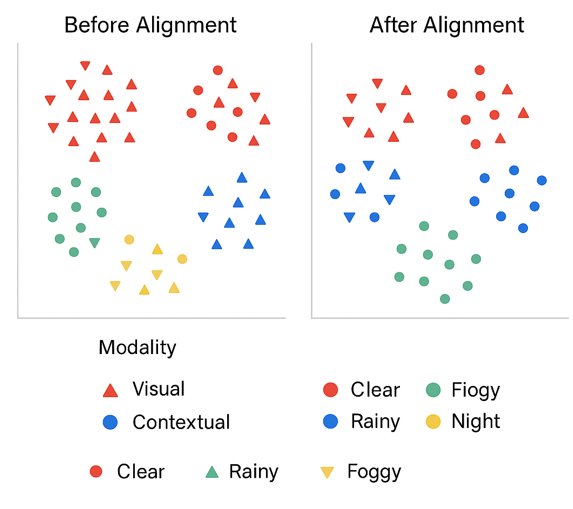
Downloads
Published
How to Cite
Issue
Section
ARK
License
Copyright (c) 2025 The author retains copyright and grants the journal the right of first publication.

This work is licensed under a Creative Commons Attribution 4.0 International License.