Enhancing Stock Price Prediction through Attention-BiLSTM and Investor Sentiment Analysis
DOI:
https://doi.org/10.5281/zenodo.14065931ARK:
https://n2t.net/ark:/40704/AJSM.v2n6a03Disciplines:
FinanceSubjects:
Financial ManagementReferences:
15Keywords:
Financial Management Methods, Smart Finance, Attention Mechanism, Stock Trend Prediction, LSTMAbstract
The change of stock price is the focus of investors in the stock market, so stock price trend prediction has always been a hot topic in quantitative investment research. Traditional machine learning prediction model is difficult to deal with nonlinear, high frequency and high noise stock price time series, which makes the prediction accuracy of stock price trend low. In order to improve the forecasting accuracy, the temporal characteristics of stock price data are studied. A bidirectional long short-term memory neural network combining empirical mode decomposition (EMD), investor sentiment and attention mechanism is proposed to predict the rise and fall of stock prices. First, the empirical mode decomposition algorithm is used to extract the characteristics of stock price time series on different time scales, and the investor complex index of the text from the close of the last trading day to the opening of the next trading day is extracted by constructing the all-inclusive sentiment dictionary.The realization of a stock price trend prediction model based on Attention-BiLSTM involves combining the Bidirectional Long Short-Term Memory (BiLSTM) network with an attention mechanism. The BiLSTM processes data points from both past and future for better context understanding, while the attention mechanism selectively focuses on crucial information, improving the model's predictive accuracy in capturing and utilizing patterns in stock price movements. This sophisticated approach enhances the model's ability to forecast stock trends effectively.
Downloads
Metrics
References
Ma, X., Wang, J., Ni, X., & Shi, J. (2024). Machine Learning Approaches for Enhancing Customer Retention and Sales Forecasting in the Biopharmaceutical Industry: A Case Study. International Journal of Engineering and Management Research, 14(5), 58-75.
Li, H., Sun, J., & Ke, X. (2024). AI-Driven Optimization System for Large-Scale Kubernetes Clusters: Enhancing Cloud Infrastructure Availability, Security, and Disaster Recovery. Journal of Artificial Intelligence General science (JAIGS) ISSN: 3006-4023, 2(1), 281-306.
Xia, S., Wei, M., Zhu, Y., & Pu, Y. (2024). AI-Driven Intelligent Financial Analysis: Enhancing Accuracy and Efficiency in Financial Decision-Making. Journal of Economic Theory and Business Management, 1(5), 1-11.
Zhang, H., Lu, T., Wang, J., & Li, L. (2024). Enhancing Facial Micro-Expression Recognition in Low-Light Conditions Using Attention-guided Deep Learning. Journal of Economic Theory and Business Management, 1(5), 12-22.
Wang, J., Lu, T., Li, L., & Huang, D. (2024). Enhancing Personalized Search with AI: A Hybrid Approach Integrating Deep Learning and Cloud Computing. International Journal of Innovative Research in Computer Science & Technology, 12(5), 127-138.
Che, C., Huang, Z., Li, C., Zheng, H., & Tian, X. (2024). Integrating generative ai into financial market prediction for improved decision making. arXiv preprint arXiv:2404.03523.
Li, L., Zhang, Y., Wang, J., & Ke, X. (2024). Deep Learning-Based Network Traffic Anomaly Detection: A Study in IoT Environments.
Cao, G., Zhang, Y., Lou, Q., & Wang, G. (2024). Optimization of High-Frequency Trading Strategies Using Deep Reinforcement Learning. Journal of Artificial Intelligence General science (JAIGS) ISSN: 3006-4023, 6(1), 230-257.
Wang, G., Ni, X., Shen, Q., & Yang, M. (2024). Leveraging Large Language Models for Context-Aware Product Discovery in E-commerce Search Systems. Journal of Knowledge Learning and Science Technology ISSN: 2959-6386 (online), 3(4).
Li, H., Wang, G., Li, L., & Wang, J. (2024). Dynamic Resource Allocation and Energy Optimization in Cloud Data Centers Using Deep Reinforcement Learning. Journal of Artificial Intelligence General science (JAIGS) ISSN: 3006-4023, 1(1), 230-258.
Che, C., Zheng, H., Huang, Z., Jiang, W., & Liu, B. (2024). Intelligent robotic control system based on computer vision technology. arXiv preprint arXiv:2404.01116.
Zheng, H.; Wu, J.; Song, R.; Guo, L.; Xu, Z. Predicting Financial Enterprise Stocks and Economic Data Trends Using Machine Learning Time Series Analysis. Applied and Computational Engineering 2024, 87, 26–32.
Ju, C., & Zhu, Y. (2024). Reinforcement Learning‐Based Model for Enterprise Financial Asset Risk Assessment and Intelligent Decision‐Making.
Huang, D., Yang, M., & Zheng, W. (2024). Integrating AI and Deep Learning for Efficient Drug Discovery and Target Identification.
Yang, M., Huang, D., & Zhan, X. (2024). Federated Learning for Privacy-Preserving Medical Data Sharing in Drug Development.
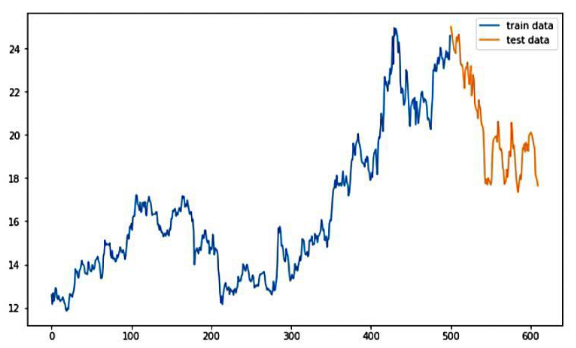
Downloads
Published
How to Cite
Issue
Section
ARK
License
Copyright (c) 2024 The author retains copyright and grants the journal the right of first publication.

This work is licensed under a Creative Commons Attribution 4.0 International License.