Deep Learning-Based Analysis of Social Media Sentiment Impact on Cryptocurrency Market Microstructure
DOI:
https://doi.org/10.70393/616a736d.323730ARK:
https://n2t.net/ark:/40704/AJSM.v3n2a02Disciplines:
BusinessSubjects:
FinanceReferences:
27Keywords:
Cryptocurrency Market Microstructure, Deep Learning, Sentiment Analysis, Market PredictionAbstract
This paper presents an advanced framework for analyzing cryptocurrency market microstructure through the integration of deep learning techniques and social media sentiment analysis. The proposed approach combines BERT-based sentiment analysis with market microstructure indicators to capture complex market dynamics. The framework processes multi-source data streams, including social media content and order book information, to generate comprehensive market insights. Experimental evaluation conducted on cryptocurrency market data from January 2022 to December 2023 demonstrates superior performance compared to traditional approaches. The model achieves 91.2% prediction accuracy and maintains a Sharpe ratio of 2.34 in trading simulations. The attention mechanism effectively identifies relevant market signals with 92.3% precision, while the temporal feature extraction module captures multi-scale market patterns. The applications have been successful with the capability of the ability to below 100 milliseconds, fit for high applications. The studies made for fields by creating the processing system for market microstructure focuses for commercial and investigators. The framework's performance stability across different market conditions validates its practical applicability in cryptocurrency trading and market analysis.
Downloads
Metrics
References
[1] Liu, X. Y., Xia, Z., Rui, J., Gao, J., Yang, H., Zhu, M., ... & Guo, J. (2022). FinRL-Meta: Market environments and benchmarks for data-driven financial reinforcement learning. Advances in Neural Information Processing Systems, 35, 1835-1849.
[2] Yang, H., Liu, X. Y., Zhong, S., & Walid, A. (2020, October). Deep reinforcement learning for automated stock trading: An ensemble strategy. In Proceedings of the first ACM international conference on AI in finance (pp. 1-8).
[3] Riva, A., Bisi, L., Liotet, P., Sabbioni, L., Vittori, E., Pinciroli, M., ... & Restelli, M. (2022, November). Addressing non-stationarity in fx trading with online model selection of offline rl experts. In Proceedings of the Third ACM International Conference on AI in Finance (pp. 394-402).
[4] Gort, B. J. D., Liu, X. Y., Sun, X., Gao, J., Chen, S., & Wang, C. D. (2022). Deep reinforcement learning for cryptocurrency trading: Practical approach to address backtest overfitting. arXiv preprint arXiv:2209.05559.
[5] Jiang, Z., & Liang, J. (2017, September). Cryptocurrency portfolio management with deep reinforcement learning. In 2017 Intelligent systems conference (IntelliSys) (pp. 905-913). IEEE.
[6] Rodríguez-Ibánez, M., Casánez-Ventura, A., Castejón-Mateos, F., & Cuenca-Jiménez, P. M. (2023). A review on sentiment analysis from social media platforms. Expert Systems with Applications, 223, 119862.
[7] Singh, M., Jakhar, A. K., & Pandey, S. (2021). Sentiment analysis on the impact of coronavirus in social life using the BERT model. Social Network Analysis and Mining, 11(1), 33.
[8] Mehta, P., Pandya, S., & Kotecha, K. (2021). Harvesting social media sentiment analysis to enhance stock market prediction using deep learning. PeerJ Computer Science, 7, e476.
[9] Nemes, L., & Kiss, A. (2021). Social media sentiment analysis based on COVID-19. Journal of Information and Telecommunication, 5(1), 1-15.
[10] Heidari, M., James Jr, H., & Uzuner, O. (2021, April). An empirical study of machine learning algorithms for social media bot detection. In 2021 IEEE International IOT, Electronics and Mechatronics Conference (IEMTRONICS) (pp. 1-5). IEEE.
[11] Xia, S., Zhu, Y., Zheng, S., Lu, T., & Ke, X. (2024). A Deep Learning-based Model for P2P Microloan Default Risk Prediction. International Journal of Innovative Research in Engineering and Management, 11(5), 110-120.
[12] Li, S., Xu, H., Lu, T., Cao, G., & Zhang, X. (2024). Emerging Technologies in Finance: Revolutionizing Investment Strategies and Tax Management in the Digital Era. Management Journal for Advanced Research, 4(4), 35-49.
[13] Liu, Y., Xu, Y., & Zhou, S. (2024). Enhancing User Experience through Machine Learning-Based Personalized Recommendation Systems: Behavior Data-Driven UI Design. Authorea Preprints.
[14] Xu, Y., Liu, Y., Wu, J., & Zhan, X. (2024). Privacy by Design in Machine Learning Data Collection: An Experiment on Enhancing User Experience. Applied and Computational Engineering, 97, 64-68.
[15] Xu, X., Xu, Z., Yu, P., & Wang, J. (2025). Enhancing User Intent for Recommendation Systems via Large Language Models. Preprints.
[16] Li, L., Xiong, K., Wang, G., & Shi, J. (2024). AI-Enhanced Security for Large-Scale Kubernetes Clusters: Advanced Defense and Authentication for National Cloud Infrastructure. Journal of Theory and Practice of Engineering Science, 4(12), 33-47.
[17] Yu, P., Xu, X., & Wang, J. (2024). Applications of Large Language Models in Multimodal Learning. Journal of Computer Technology and Applied Mathematics, 1(4), 108-116.
[18] Huang, D., Yang, M., & Zheng, W. (2024). Using Deep Reinforcement Learning for Optimizing Process Parameters in CHO Cell Cultures for Monoclonal Antibody Production. Artificial Intelligence and Machine Learning Review, 5(3), 12-27.
[19] Huang, T., Xu, Z., Yu, P., Yi, J., & Xu, X. (2025). A Hybrid Transformer Model for Fake News Detection: Leveraging Bayesian Optimization and Bidirectional Recurrent Unit. arXiv preprint arXiv:2502.09097.
[20] Weng, J., Jiang, X., & Chen, Y. (2024). Real-time Squat Pose Assessment and Injury Risk Prediction Based on Enhanced Temporal Convolutional Neural Networks.
[21] Xu, X., Yu, P., Xu, Z., & Wang, J. (2025). A hybrid attention framework for fake news detection with large language models. arXiv preprint arXiv:2501.11967.
[22] Ma, X., & Fan, S. (2024). Research on Cross-national Customer Churn Prediction Model for Biopharmaceutical Products Based on LSTM-Attention Mechanism. Academia Nexus Journal, 3(3).
[23] Chen, Y., Feng, E., & Ling, Z. (2024). Secure Resource Allocation Optimization in Cloud Computing Using Deep Reinforcement Learning. Journal of Advanced Computing Systems, 4(11), 15-29.
[24] Shen, Q., Zhang, Y., & Xi, Y. (2024). Deep Learning-Based Investment Risk Assessment Model for Distributed Photovoltaic Projects. Journal of Advanced Computing Systems, 4(3), 31-46.
[25] Chen, J., Zhang, Y., & Wang, S. (2024). Deep Reinforcement Learning-Based Optimization for IC Layout Design Rule Verification. Journal of Advanced Computing Systems, 4(3), 16-30.
[26] Bi, W., Trinh, T. K., & Fan, S. (2024). Machine Learning-Based Pattern Recognition for Anti-Money Laundering in Banking Systems. Journal of Advanced Computing Systems, 4(11), 30-41.
[27] Jiang, C., Zhang, H., & Xi, Y. (2024). Automated Game Localization Quality Assessment Using Deep Learning: A Case Study in Error Pattern Recognition. Journal of Advanced Computing Systems, 4(10), 25-37.
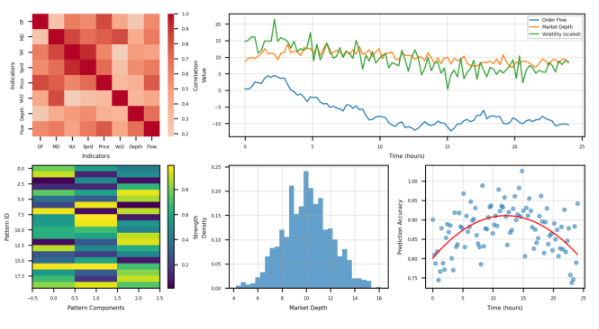
Downloads
Published
How to Cite
Issue
Section
ARK
License
Copyright (c) 2025 The author retains copyright and grants the journal the right of first publication.

This work is licensed under a Creative Commons Attribution 4.0 International License.