A New Paradigm of Personalized Education Driven by Multi-Agent Collaboration
DOI:
https://doi.org/10.70393/616a736d.323931ARK:
https://n2t.net/ark:/40704/AJSM.v3n3a02Disciplines:
EducationSubjects:
Educational TechnologyReferences:
39Keywords:
Multi-agent Collaboration, Fusion of Large Language Model and Small Model, Personalized Learning Experiences, Intelligent Transformation of EducationAbstract
In order to promote the in-depth development of intelligent education, this paper proposes an intelligent collaboration framework that integrates general-purpose large language models and professional small models. By integrating the broad language comprehension ability of the general model and the fine grasp of subject knowledge of the domain-specific model, and combining the teaching mechanism in learning theory, the framework realizes a diversified knowledge construction method and supports a highly personalized and dynamically adaptive learning experience. We further explore the practical application scenarios of the framework in intelligent education, and demonstrate its potential in teaching assistance, learning guidance and knowledge transfer. With the continuous evolution of artificial intelligence technology, the collaborative system is expected to become a key engine to promote the intelligent transformation of education in the future.
Downloads
Metrics
References
[1] Hu, X., Niu, R., & Tang, T. (2021). Pre-processing of metro signaling equipment fault text based on fusion of lexical domain and semantic domain. Journal of the China Railway Society, 43(02), 78-85.
[2] Zhong, Y. (2024). Enhancing the Heat Dissipation Efficiency of Computing Units Within Autonomous Driving Systems and Electric Vehicles.
[3] Yekollu, R. K., Bhimraj Ghuge, T., Sunil Biradar, S., Haldikar, S. V., & Farook Mohideen Abdul Kader, O. (2024, February). AI-driven personalized learning paths: Enhancing education through adaptive systems. In International Conference on Smart data intelligence (pp. 507-517). Singapore: Springer Nature Singapore.
[4] Hanna, M. G., Pantanowitz, L., Dash, R., Harrison, J. H., Deebajah, M., Pantanowitz, J., & Rashidi, H. H. (2025). Future of Artificial Intelligence (AI)-Machine Learning (ML) Trends in Pathology and Medicine. Modern Pathology, 10070
[5] Ma, C., Hou, D., Jiang, J., Fan, Y., Li, X., Li, T., ... & Xiong, H. (2022). Elucidating the Synergic Effect in Nanoscale MoS2/TiO2 Heterointerface for Na‐Ion Storage. Advanced Science, 9(35), 2204837.
[6] Tran, K. T., Dao, D., Nguyen, M. D., Pham, Q. V., O'Sullivan, B., & Nguyen, H. D. (2025). Multi-Agent Collaboration Mechanisms: A Survey of LLMs. arXiv preprint arXiv:2501.06322.
[7] Guo, Z. B., Xu, L. F., Zheng, Y. H., Xie, J. S., & Wang, T. T. (2025). Bearing fault diagnostic framework under unknown working conditions based on condition-guided diffusion model. Measurement, 242, Article 115951. https://doi.org/10.1016/j.measurement.2024.115951
[8] Cen, Z., & Zhao, Y. (2024). Enhancing User Engagement through Adaptive Interfaces: A Study on Real-time Personalization in Web Applications. Journal of Economic Theory and Business Management, 1(6), 1-7.
[9] Zhao, Y., & Cen, Z. (2024). Exploring Multimodal Feedback Mechanisms for Improving User Interaction in Virtual Reality Environments. Journal of Industrial Engineering and Applied Science, 2(6), 35-41.
[10] Xiao, J., Zhang, B., Zhao, Y., Wu, J., & Qu, P. (2024). Application of Large Language Models in Personalized Advertising Recommendation Systems. Journal of Industrial Engineering and Applied Science, 2(4), 132-142.
[11] Zhao, Y., Qu, P., Xiao, J., Wu, J., & Zhang, B. (2024). Optimizing Telehealth Services with LILM-Driven Conversational Agents: An HCI Evaluation. Journal of Industrial Engineering and Applied Science, 2(4), 122-131.
[12] Zhao, Y., Wu, J., Qu, P., Zhang, B., & Yan, H. (2024). Assessing User Trust in LLM-based Mental Health Applications: Perceptions of Reliability and Effectiveness. Journal of Computer Technology and Applied Mathematics, 1(2), 19-26.
[13] Jiang, C., Ding, R., Cui, X., Xie, Y., Zhao, Z., Wang, X., & Qiao, Y. Industry enters the XPU era: from an educational view.
[14] Sheng, Z., Wu, F., Zuo, X., Li, C., Qiao, Y., & Lei, H. (2024, November). Research on the LLM-Driven Vulnerability Detection System Using LProtector. In 2024 IEEE 4th International Conference on Data Science and Computer Application (ICDSCA) (pp. 192-196). IEEE.
[15] Zhang, W., Huang, J., Wang, R., Wei, C., Huang, W., & Qiao, Y. (2024, October). Integration of Mamba and Transformer-MAT for Long-Short Range Time Series Forecasting with Application to Weather Dynamics. In 2024 International Conference on Electrical, Communication and Computer Engineering (ICECCE) (pp. 1-6). IEEE.
[16] Khosla, R., & Ichalkaranje, N. (2013). Design of intelligent multi-agent systems: human-centredness, architectures, learning and adaptation. Springer.
[17] Qiao, Y., Li, K., Lin, J., Wei, R., Jiang, C., Luo, Y., & Yang, H. (2024, June). Robust domain generalization for multi-modal object recognition. In 2024 5th International Conference on Artificial Intelligence and Electromechanical Automation (AIEA) (pp. 392-397). IEEE.
[18] Li, Z., Yazdanpanah, V., Sarkadi, S., He, Y., Shafipour, E., & Stein, S. (2024, September). Towards Citizen-Centric Multiagent Systems Based on Large Language Models. In Proceedings of the 2024 International Conference on Information Technology for Social Good (pp. 26-31).
[19] Wang, Y., Pan, Y., Zhao, Q., Deng, Y., Su, Z., Du, L., & Luan, T. H. (2024). Large Model Agents: State-of-the-Art, Cooperation Paradigms, Security and Privacy, and Future Trends. arXiv preprint arXiv:2409.14457.
[20] Cruz, C. J. X. (2024). Transforming Competition into Collaboration: The Revolutionary Role of Multi-Agent Systems and Language Models in Modern Organizations. arXiv preprint arXiv:2403.07769.
[21] Su, J., Jiang, C., Jin, X., Qiao, Y., Xiao, T., Ma, H., ... & Lin, J. (2024). Large language models for forecasting and anomaly detection: A systematic literature review. arXiv preprint arXiv:2402.10350.
[22] Gao, C., Lan, X., Li, N., Yuan, Y., Ding, J., Zhou, Z., ... & Li, Y. (2024). Large language models empowered agent-based modeling and simulation: A survey and perspectives. Humanities and Social Sciences Communications, 11(1), 1-24.
[23] Herrouz, A., Djoudi, M., Degha, H. E., & Boukanoun, B. (2023). An Autonomous Multi-Agent System for Customized Scientific Literature Recommendation: A Tool for Researchers and Students. Revue des Sciences et Technologies de l'Information-Série ISI: Ingénierie des Systèmes d'Information, 28(4), 799-814.
[24] Spaho, E., Çiço, B., & Shabani, I. (2025). IoT Integration Approaches into Personalized Online Learning: Systematic Review. Computers, 14(2), 63.
[25] Wang, X., Qiao, Y., Xiong, J., Zhao, Z., Zhang, N., Feng, M., & Jiang, C. (2024). Advanced network intrusion detection with tabtransformer. Journal of Theory and Practice of Engineering Science, 4(03), 191-198.
[26] Xu, S., Zhang, X., & Qin, L. (2024). Eduagent: Generative student agents in learning. arXiv preprint arXiv:2404.07963.
[27] Holtz, N., Wittfoth, S., & Gómez, J. M. (2024, August). The New Era of Knowledge Retrieval: Multi-Agent Systems Meet Generative AI. In 2024 Portland International Conference on Management of Engineering and Technology (PICMET) (pp. 1-10). IEEE.
[28] Hoang, K., Huynh, T., Tran, D. H., Pham, T., & Tran, B. Personalized Learning Through Gamification: Multi-Agent and Large Language Model Approaches.
[29] Zhang, N., Xiong, J., Zhao, Z., Feng, M., Wang, X., Qiao, Y., & Jiang, C. (2024). Dose my opinion count? A CNN-LSTM approach for sentiment analysis of Indian general elections. Journal of Theory and Practice of Engineering Science, 4(05), 40-50.
[30] Vuković, I., Kuk, K., Čisar, P., Banđur, M., Banđur, Đ., Milić, N., & Popović, B. (2021). Multi-agent system observer: Intelligent support for engaged e-learning. Electronics, 10(12), 1370.
[31] Yuan, L., Zhang, Z., Li, L., Guan, C., & Yu, Y. (2023). A survey of progress on cooperative multi-agent reinforcement learning in open environment. arXiv preprint arXiv:2312.01058.
[32] Lykov, A., Dronova, M., Naglov, N., Litvinov, M., Satsevich, S., Bazhenov, A., ... & Tsetserukou, D. (2023). Llm-mars: Large language model for behavior tree generation and nlp-enhanced dialogue in multi-agent robot systems. arXiv preprint arXiv:2312.09348.
[33] Lin, C. S. (2025). A hybrid model for the detection of multi-agent written news articles based on linguistic features and BERT. The Journal of Supercomputing, 81(2), 381.
[34] Händler, T. (2023). Balancing autonomy and alignment: a multi-dimensional taxonomy for autonomous LLM-powered multi-agent architectures. arXiv preprint arXiv:2310.03659.
[35] Mansour, A. M. O., Obeidat, M. A. A., & Abdallah, J. M. Y. (2023). A Multi-Agent Systems Approach for Optimized Biomedical Literature Search. Ingenierie des Systemes d'Information, 28(4), 1039.
[36] Aryal, S., Do, T., Heyojoo, B., Chataut, S., Gurung, B. D. S., Gadhamshetty, V., & Gnimpieba, E. (2024). Leveraging multi-AI agents for cross-domain knowledge discovery. arXiv preprint arXiv:2404.08511.
[37] Spaho, E., Çiço, B., & Shabani, I. (2025). IoT Integration Approaches into Personalized Online Learning.
[38] Hanga, K. M., & Kovalchuk, Y. (2019). Machine learning and multi-agent systems in oil and gas industry applications: A survey. Computer Science Review, 34, 10019
[39] Nakashima, H., Aghajan, H., & Augusto, J. C. (Eds.). (2009). Handbook of ambient intelligence and smart environments. Springer Science & Business Media.
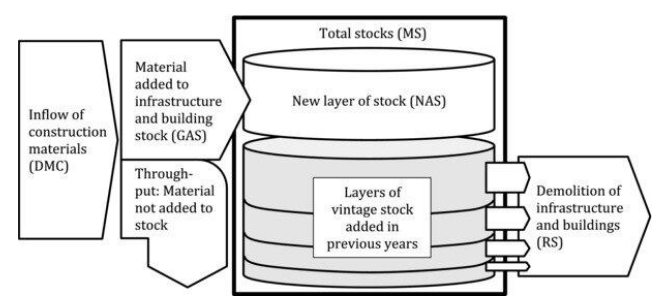
Downloads
Published
How to Cite
Issue
Section
ARK
License
Copyright (c) 2025 The author retains copyright and grants the journal the right of first publication.

This work is licensed under a Creative Commons Attribution 4.0 International License.