AI Driven Digital Transformation in the Architecture Industry with Technical Implementation and Business Process Optimization Review
DOI:
https://doi.org/10.70393/616a736d.323934ARK:
https://n2t.net/ark:/40704/AJSM.v3n3a07Disciplines:
BusinessSubjects:
EntrepreneurshipReferences:
28Keywords:
Artificial Intelligence (AI), Digital Transformation, Architecture Industry, Generative Design, Building Information Modeling (BIM), Computer Vision, Natural Language Processing (NLP), Business Process Optimization, Sustainability, Risk Management, Automation, Design InnovationAbstract
There is a big digital revolution emerging in architecture, driven by ML (Machine Learning) and AI (Artificial Intelligence). These technologies have brought the design–construction–building lifecycle process much closer to modern working methods, and they have complemented, rather than replaced, existing systems. In this article, we present an overview of AI-facilitated digital transformation in architecture, focusing on three key technologies: generative design, computer vision, and natural language processing (NLP), and showing how they improve businesses in both strategic and operational aspects. For a more tangible perspective, we showcase, through specific applications and emerging tools, how AI enhances design quality, efficiency, sustainability, and client experience. The report also highlights major barriers to AI implementation, from lack of data interoperability and skills shortages to company culture. This article demonstrates how AI is disrupting established architectural practices, and the ways in which its fusion with business models and market forces will shape the future of the built environment.
Downloads
Metrics
References
[1] Merschbrock, C., & Munkvold, B. E. (2015). Effective digital collaboration in the construction industry–A case study of BIM deployment in a hospital construction project. Computers in Industry, 73, 1-7.
[2] Lin, W., Xiao, J., & Cen, Z. (2024). Exploring Bias in NLP Models: Analyzing the Impact of Training Data on Fairness and Equity. Journal of Industrial Engineering and Applied Science, 2(5), 24-28.
[3] Chen, Q. Fu, Y. Yuan, Z. Wen, G. Fan, D. Liu, D. Zhang, Z. Li, and Y. Xiao, Hallucination detection: Robustly discerning reliable answers in large language models, in Proceedings of the 32nd ACM International Conference on Information and Knowledge Management, 2023, pp. 245–255.
[4] Wang, J., Cao, S., Zhang, R., Li, S., & Tse, T. K. (2024). Uncertainty of typhoon extreme wind speeds in Hong Kong integrating the effects of climate change. Physics of Fluids, 36, 087126
[5] Lyu, S. (2024). The Application of Generative AI in Virtual Reality and Augmented Reality. Journal of Industrial Engineering and Applied Science, 2(6), 1-9.
[6] Li, K., Chen, X., Song, T., Zhou, C., Liu, Z., Zhang, Z., Guo, J., & Shan, Q. (2025a, March 24). Solving situation puzzles with large language model and external reformulation.
[7] Rane, N. (2023). Integrating leading-edge artificial intelligence (AI), internet of things (IOT), and big data technologies for smart and sustainable architecture, engineering and construction (AEC) industry: Challenges and future directions. Engineering and Construction (AEC) Industry: Challenges and Future Directions (September 24, 2023).
[8] Li, K., Liu, L., Chen, J., Yu, D., Zhou, X., Li, M., ... & Li, Z. (2024, November). Research on reinforcement learning based warehouse robot navigation algorithm in complex warehouse layout. In 2024 6th International Conference on Artificial Intelligence and Computer Applications (ICAICA) (pp. 296-301). IEEE.
[9] Khanzode, A. R. (2011). An integrated virtual design and construction and lean (IVL) method for coordination of mechanical, electrical and plumbing (MEP) systems. Stanford University.
[10] Sun, J., Zhang, S., Lian, J., Fu, L., Zhou, Z., Fan, Y., & Xu, K. (2024, December). Research on Deep Learning of Convolutional Neural Network for Action Recognition of Intelligent Terminals in the Big Data Environment and its Intelligent Software Application. In 2024 IEEE 7th International Conference on Automation, Electronics and Electrical Engineering (AUTEEE) (pp. 996-1004). IEEE.
[11] Luo, M., Du, B., Zhang, W., Song, T., Li, K., Zhu, H., ... & Wen, H. (2023). Fleet rebalancing for expanding shared e-Mobility systems: A multi-agent deep reinforcement learning approach. IEEE Transactions on Intelligent Transportation Systems, 24(4), 3868-3881.
[12] Lin, W. (2025). Enhancing Video Conferencing Experience through Speech Activity Detection and Lip Synchronization with Deep Learning Models. Journal of Computer Technology and Applied Mathematics, 2(2), 16-23.
[13] Lin, W. (2024). A Systematic Review of Computer Vision-Based Virtual Conference Assistants and Gesture Recognition. Journal of Computer Technology and Applied Mathematics, 1(4), 28-35.
[14] Zuo, Q., Tao, D., Qi, T., Xie, J., Zhou, Z., Tian, Z., & Mingyu, Y. (2025). Industrial Internet Robot Collaboration System and Edge Computing Optimization. arXiv preprint arXiv:2504.02492.
[15] Luo, M., Zhang, W., Song, T., Li, K., Zhu, H., Du, B., & Wen, H. (2021, January). Rebalancing expanding EV sharing systems with deep reinforcement learning. In Proceedings of the Twenty-Ninth International Conference on International Joint Conferences on Artificial Intelligence (pp. 1338-1344).
[16] Wu, S., Fu, L., Chang, R., Wei, Y., Zhang, Y., Wang, Z., ... & Li, K. (2025). Warehouse Robot Task Scheduling Based on Reinforcement Learning to Maximize Operational Efficiency. Authorea Preprints.
[17] Rane, N., Choudhary, S., & Rane, J. (2023). Leading-edge technologies for architectural design: a comprehensive review. Available at SSRN 4637891.
[18] Li, K., Chen, X., Song, T., Zhang, H., Zhang, W., & Shan, Q. (2024). GPTDrawer: Enhancing Visual Synthesis through ChatGPT. arXiv preprint arXiv:2412.10429.
[19] Saad, S., Haris, M., Ammad, S., & Rasheed, K. (2024). AI-assisted building design. In AI in material science (pp. 143-168). CRC Press.
[20] Lyu, S. (2024). Machine Vision-Based Automatic Detection for Electromechanical Equipment. Journal of Computer Technology and Applied Mathematics, 1(4), 12-20.
[21] Lin, W. (2024). The Application of Real-time Emotion Recognition in Video Conferencing. Journal of Computer Technology and Applied Mathematics, 1(4), 79-88.
[22] Zhou, Z. (2025). Research on the Application of Intelligent Robots and Software in Multiple Segmentation Scenarios Based on Machine Learning. Available at SSRN 5236930.
[23] Balakrishnan, A. (2024). Leveraging artificial intelligence for enhancing regulatory compliance in the financial sector. International Journal of Computer Trends and Technology.
[24] Y. Chen, J. Zhao, Z. Wen, Z. Li, and Y. Xiao, Temporalmed: Advancing medical dialogues with time-aware responses in large language models, in Proceedings of the 17th ACM International Conference on Web Search and Data Mining, 2024, pp. 116–124.
[25] Li, X., Cao, H., Zhang, Z., Hu, J., Jin, Y., & Zhao, Z. (2024). Artistic Neural Style Transfer Algorithms with Activation Smoothing. arXiv preprint arXiv:2411.08014.
[26] He, Y., Li, S., Li, K., Wang, J., Li, B., Shi, T., ... & Wang, X. (2025). Enhancing Low-Cost Video Editing with Lightweight Adaptors and Temporal-Aware Inversion. arXiv preprint arXiv:2501.04606.
[27] Dezhi Yu, Lipeng Liu, Siye Wu, et al. Machine learning optimizes the efficiency of picking and packing in automated warehouse robot systems. TechRxiv. January 21, 2025. DOI: 10.36227/techrxiv.173750249.93643684/v1.
[28] Mao, Y., Tao, D., Zhang, S., Qi, T., & Li, K. (2025). Research and Design on Intelligent Recognition of Unordered Targets for Robots Based on Reinforcement Learning. arXiv preprint arXiv:2503.07340.
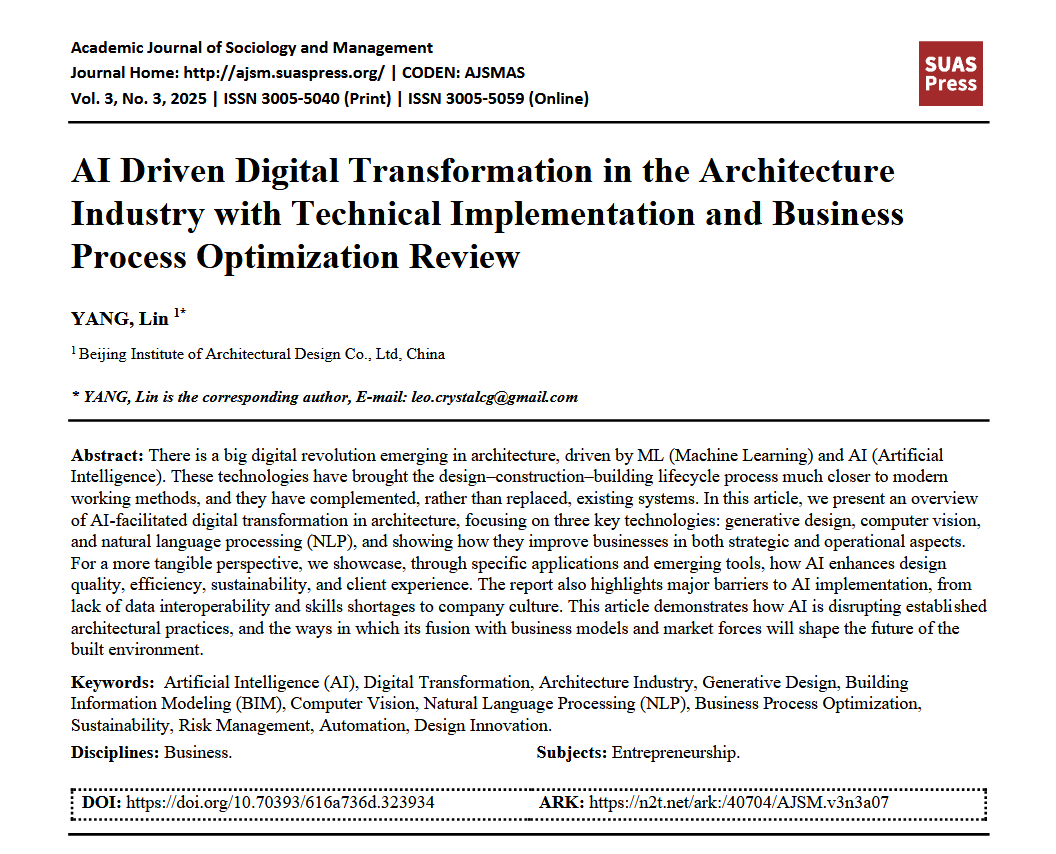
Downloads
Published
How to Cite
Issue
Section
ARK
License
Copyright (c) 2025 The author retains copyright and grants the journal the right of first publication.

This work is licensed under a Creative Commons Attribution 4.0 International License.