Overview of Multimodal Generative Models in Natural Language Processing and Computer Vision
DOI:
https://doi.org/10.5281/zenodo.13988327ARK:
https://n2t.net/ark:/40704/JCTAM.v1n4a09Disciplines:
Artificial IntelligenceSubjects:
Multimodal Generative ModelsReferences:
18Keywords:
Multimodal Generative Models, Natural Language Processing, Computer Vision, Data Fusion, Deep Learning, CLIP, DALL·EAbstract
Multimodal generative models have become essential in the deep learning renaissance, as they provide unparalleled flexibility over a diverse context of applications within Natural Language Processing (NLP) and Computer Vision (CV). In this paper, we systematically review the basic concepts and technical improvements in multimodal generative models by discussing their applications across different modalities such as text, images, audio,and video. These models though augment the strength of AI to comprehend and perform complicated tasks by coalescing data from various modalities. In this paper, we investigate how these principles apply to many of the existing mainstream models (including CLIP, DALL·E, Flamingo), and consider their applications in VQA,text-to-image-synthesis; medical image analysis; edutainment content creation & user research developments. This paper also examines the existing difficulties of such technologies including paucity in data availability, modality fusion effectiveness and constraints on computational resources while suggesting pathways for future research. The paper goes on to state privacy parallels between multi-modal generative models (GGMs) calls for a model of safety over responsibility when it comes to technological innovation.
References
Huang, X., Wu, Y., Zhang, D., Hu, J., & Long, Y. (2024). Improving Academic Skills Assessment with NLP and Ensemble Learning. arXiv preprint arXiv:2409.19013.
Ma, B., Ma, B., Gao, M., Wang, Z., Ban, X., Huang, H., & Wu, W. (2021). Deep learning‐based automatic inpainting for material microscopic images. Journal of Microscopy, 281(3), 177-189.
Liu, W., Cheng, S., Zeng, D., & Qu, H. (2023). Enhancing document-level event argument extraction with contextual clues and role relevance. arXiv preprint arXiv:2310.05991.
Wang, D. (Ed.). (2016). Information Science and Electronic Engineering: Proceedings of the 3rd International Conference of Electronic Engineering and Information Science (ICEEIS 2016), January 4-5, 2016, Harbin, China. CRC Press.
Liu, W., Zhou, L., Zeng, D., Xiao, Y., Cheng, S., Zhang, C., ... & Chen, W. (2024). Beyond Single-Event Extraction: Towards Efficient Document-Level Multi-Event Argument Extraction. arXiv preprint arXiv:2405.01884.
Lu, J. (2024). Optimizing E-Commerce with Multi-Objective Recommendations Using Ensemble Learning.
Yu, P., Cui, V. Y., & Guan, J. (2021, March). Text classification by using natural language processing. In Journal of Physics: Conference Series (Vol. 1802, No. 4, p. 042010). IOP Publishing.
Jiang, L., Yang, X., Yu, C., Wu, Z., & Wang, Y. (2024, July). Advanced AI framework for enhanced detection and assessment of abdominal trauma: Integrating 3D segmentation with 2D CNN and RNN models. In 2024 3rd International Conference on Robotics, Artificial Intelligence and Intelligent Control (RAIIC) (pp. 337-340). IEEE.
Wang, Y., Ban, X., Wang, H., Li, X., Wang, Z., Wu, D., ... & Liu, S. (2019). Particle filter vehicles tracking by fusing multiple features. IEEE Access, 7, 133694-133706.
Wang, C., Kang, D., Sun, H. Y., Qian, S. H., Wang, Z. X., Bao, L., & Zhang, S. H. (2024). MeGA: Hybrid Mesh-Gaussian Head Avatar for High-Fidelity Rendering and Head Editing. arXiv preprint arXiv:2404.19026.
Bačić, B., Feng, C., & Li, W. (2024). JY61 IMU SENSOR EXTERNAL VALIDITY: A FRAMEWORK FOR ADVANCED PEDOMETER ALGORITHM PERSONALISATION. ISBS Proceedings Archive, 42(1), 60.
Qu, M. (2024). High Precision Measurement Technology of Geometric Parameters Based on Binocular Stereo Vision Application and Development Prospect of The System in Metrology and Detection. Journal of Computer Technology and Applied Mathematics, 1(3), 23-29.
Zhang, Y., Wang, F., Huang, X., Li, X., Liu, S., & Zhang, H. (2024). Optimization and Application of Cloud-based Deep Learning Architecture for Multi-Source Data Prediction. arXiv preprint arXiv:2410.12642.
Cao, Y., Weng, Y., Li, M., & Yang, X. The Application of Big Data and AI in Risk Control Models: Safeguarding User Security.
Liu T, Wu Y, Ye A, Cao L, Cao Y. Two-stage sparse multi-objective evolutionary algorithm for channel selection optimization in BCIs. Frontiers in Human Neuroscience. 2024 May 22;18:1400077.
Zhang, M., Liu, Y., Zhang, B., Li, S., & Yu, H. (2024). Unilateral complete ureteral duplication with ectopic ureteral opening inserting into urethra in a female patient without incontinence: a case description and review of the literature. Quantitative Imaging in Medicine and Surgery, 14(8), 6166172-6166172.
Zhang, M., Li, S., Tian, C., Li, M., Zhang, B., & Yu, H. (2024). Changes of uterocervical angle and cervical length in early and mid-pregnancy and their value in predicting spontaneous preterm birth. Frontiers in Physiology, 15, 1304513.
Leong, H. Y., Gao, Y. F., Shuai, J., Zhang, Y., & Pamuksuz, U. (2024). Efficient Fine-Tuning of Large Language Models for Automated Medical Documentation. arXiv preprint arXiv:2409.09324.
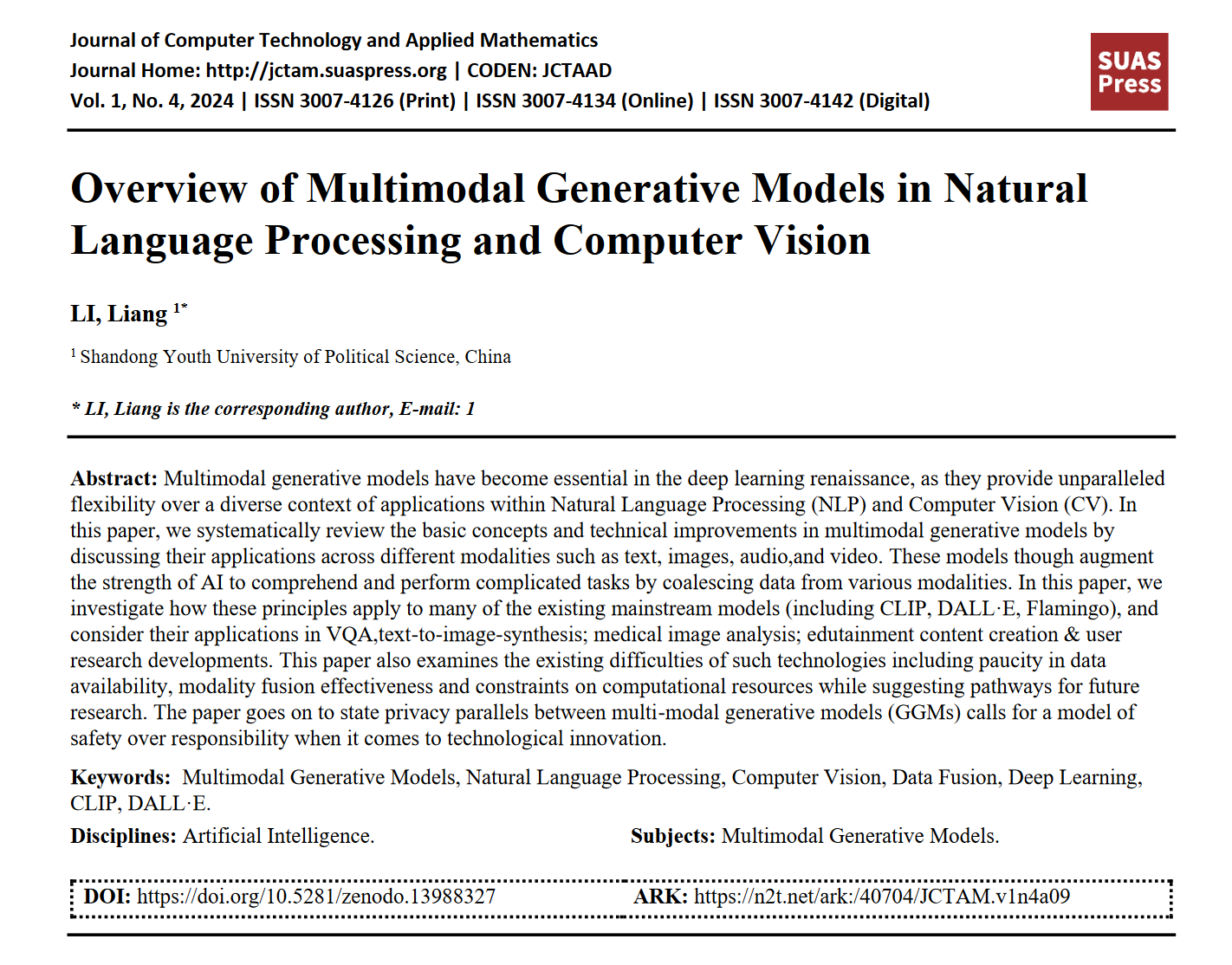
Downloads
Published
How to Cite
Issue
Section
ARK
License
Copyright (c) 2024 The author retains copyright and grants the journal the right of first publication.

This work is licensed under a Creative Commons Attribution 4.0 International License.