A Comprehensive Review of Artificial Intelligence Applications in Building Information Modeling (BIM) and Future Perspectives
DOI:
https://doi.org/10.70393/6a6374616d.323635ARK:
https://n2t.net/ark:/40704/JCTAM.v2n2a01Disciplines:
Artificial Intelligence and IntelligenceSubjects:
Machine LearningReferences:
26Keywords:
Artificial Intelligence, Building Information Modeling, Machine Learning, Deep Learning, Reinforcement Learning, Generative Adversarial Networks, Natural Language Processing, Computer Vision, Construction Technology, Smart Cities, Sustainability, IoT, Blockchain, Augmented RealityAbstract
Technologies like Artificial Intelligence (AI), the Internet of Things (IoT), cloud computing, and big data analytics are converging and leading the construction industry to a transformation towards digitization and enhancing construction efficiency. At the centre of this evolution sits Building Information Modeling (BIM), transforming from rudimentary 3D modeling tools to a holistic building lifecycle management tool. This article presents a literature review of AI applications in BIM with a focus on how machine learning, deep learning, reinforcement learning, generative adversarial networks, natural language processing, and computer vision reshape the construction industry. AI has been integrated with BIM to improve project efficiency, minimize mistakes, enhance resource allocation, and transition towards a more sustainable practice. This review also highlights current technical obstacles causing the delay of projects such as the pilot studies based on AI-enhanced BIM in the field and future research directions liaising interdisciplinary infrastructure with IoT, blockchain, augmented reality, etc. The article concludes by discussing the gradual co-evolution of adaptive artificial intelligence systems and BIM, concluding that innovation in sustainable development must always be nurtured.
References
[1] Li, K., Chen, X., Song, T., Zhang, H., Zhang, W., & Shan, Q. (2024). GPTDrawer: Enhancing Visual Synthesis through ChatGPT. arXiv preprint arXiv:2412.10429.
[2] Yashchenko, O., Makatora, D., Kubanov, R., Zinych, P., & Prusov, D. (2024). Theoretical and Methodological Bases for Implementing BIM Technologies in Construction Companies: Essence. Characteristics. Economic Efficiency.
[3] Lyu, S. (2024). The Application of Generative AI in Virtual Reality and Augmented Reality. Journal of Industrial Engineering and Applied Science, 2(6), 1-9.
[4] Waqar, A. (2024). Intelligent decision support systems in construction engineering: An artificial intelligence and machine learning approaches. Expert Systems with Applications, 249, 123503.
[5] Luo, M., Zhang, W., Song, T., Li, K., Zhu, H., Du, B., & Wen, H. (2021, January). Rebalancing expanding EV sharing systems with deep reinforcement learning. In Proceedings of the Twenty-Ninth International Conference on International Joint Conferences on Artificial Intelligence (pp. 1338-1344).
[6] Rane, N., Choudhary, S., & Rane, J. (2023). Artificial Intelligence (AI) and Internet of Things (IoT)-based sensors for monitoring and controlling in architecture, engineering, and construction: applications, challenges, and opportunities. Available at SSRN 4642197.
[7] Obiuto, N. C., Festus-Ikhuoria, I. C., Olajiga, O. K., & Adebayo, R. A. (2024). REVIEWING THE ROLE OF AI IN DRONE TECHNOLOGY AND APPLICATIONS. Computer Science & IT Research Journal, 5(4), 741-756.
[8] Zhu, H., Luo, Y., Liu, Q., Fan, H., Song, T., Yu, C. W., & Du, B. (2019). Multistep flow prediction on car-sharing systems: A multi-graph convolutional neural network with attention mechanism. International Journal of Software Engineering and Knowledge Engineering, 29(11n12), 1727–1740.
[9] Zabin, A., González, V. A., Zou, Y., & Amor, R. (2022). Applications of machine learning to BIM: A systematic literature review. Advanced Engineering Informatics, 51, 101474.
[10] Pan, Y., Shen, Y., Qin, J., & Zhang, L. (2024). Deep reinforcement learning for multi-objective optimization in BIM-based green building design. Automation in Construction, 166, 105598.
[11] Asghari, V., Wang, Y., Biglari, A. J., Hsu, S. C., & Tang, P. (2022). Reinforcement learning in construction engineering and management: A review. Journal of Construction Engineering and Management, 148(11), 03122009.
[12] Lin, W. (2024). A Systematic Review of Computer Vision-Based Virtual Conference Assistants and Gesture Recognition. Journal of Computer Technology and Applied Mathematics, 1(4), 28-35.
[13] Rane, N., Choudhary, S., & Rane, J. (2023). Integrating Building Information Modelling (BIM) with ChatGPT, Bard, and similar generative artificial intelligence in the architecture, engineering, and construction industry: applications, a novel framework, challenges, and future scope. Bard, and similar generative artificial intelligence in the architecture, engineering, and construction industry: applications, a novel framework, challenges, and future scope (November 22, 2023).
[14] Rane, N., Choudhary, S., & Rane, J. (2023). Leading-edge technologies for architectural design: a comprehensive review. Available at SSRN 4637891.
[15] Locatelli, M., Seghezzi, E., Pellegrini, L., Tagliabue, L. C., & Di Giuda, G. M. (2021). Exploring natural language processing in construction and integration with building information modeling: A scientometric analysis. Buildings, 11(12), 583.
[16] Lyu, S. (2024). Machine Vision-Based Automatic Detection for Electromechanical Equipment. Journal of Computer Technology and Applied Mathematics, 1(4), 12-20
[17] Jiang, S., Zhang, J., Shi, J., & Wu, Y. (2024). Adaptive information retrieval for enhanced building safety management leveraging BIM. Engineering, Construction and Architectural Management.
[18] Huang, M. Q., Ninić, J., & Zhang, Q. (2021). BIM, machine learning and computer vision techniques in underground construction: Current status and future perspectives. Tunnelling and Underground Space Technology, 108, 103677.
[19] Luo, M., Du, B., Zhang, W., Song, T., Li, K., Zhu, H., ... & Wen, H. (2023). Fleet rebalancing for expanding shared e-Mobility systems: A multi-agent deep reinforcement learning approach. IEEE Transactions on Intelligent Transportation Systems, 24(4), 3868-3881.
[20] Golafshani, E., Chiniforush, A. A., Zandifaez, P., & Ngo, T. (2024). An artificial intelligence framework for predicting operational energy consumption in office buildings. Energy and Buildings, 114409.
[21] Li, G., Zhao, X., Fan, C., Fang, X., Li, F., & Wu, Y. (2021). Assessment of long short-term memory and its modifications for enhanced short-term building energy predictions. Journal of building engineering, 43, 103182.
[22] Kaklauskas, A., Zavadskas, E. K., Lepkova, N., Raslanas, S., Dauksys, K., Vetloviene, I., & Ubarte, I. (2021). Sustainable construction investment, real estate development, and COVID-19: a review of literature in the field. Sustainability, 13(13), 7420.
[23] Banihashemi, S., Golizadeh, H., & Rahimian, F. P. (2022). Data-driven BIM for energy efficient building design. Routledge.
[24] Lyu, S. (2024). The Technology of Face Synthesis and Editing Based on Generative Models. Journal of Computer Technology and Applied Mathematics, 1(4), 21-27.
[25] Lin, W. (2024). A Review of Multimodal Interaction Technologies in Virtual Meetings. Journal of Computer Technology and Applied Mathematics, 1(4), 60-68.
[26] Sun, Y., Pai, N., Ramesh, V.V., Aldeer, M. and Ortiz, J., 2023. GeXSe (Generative Explanatory Sensor System): An Interpretable Deep Generative Model for Human Activity Recognition in Smart Spaces. arXiv preprint arXiv:2306.15857.
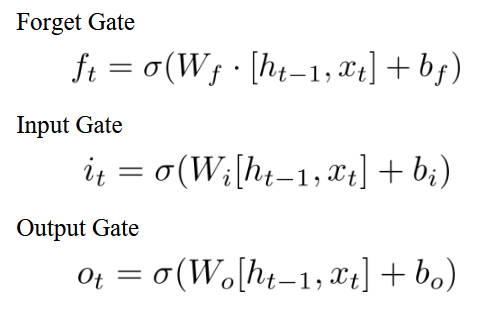
Downloads
Published
How to Cite
Issue
Section
ARK
License
Copyright (c) 2025 The author retains copyright and grants the journal the right of first publication.

This work is licensed under a Creative Commons Attribution 4.0 International License.