Enhancing Video Conferencing Experience through Speech Activity Detection and Lip Synchronization with Deep Learning Models
DOI:
https://doi.org/10.70393/6a6374616d.323637ARK:
https://n2t.net/ark:/40704/JCTAM.v2n2a03Disciplines:
Artificial Intelligence and IntelligenceSubjects:
Speech RecognitionReferences:
27Keywords:
Speech Activity Detection, Lip Synchronization, Deep Learning, Video Conferencing, Multimodal Fusion, Dynamic Time Warping, User Experience, Real-Time CommunicationAbstract
As video conferencing becomes increasingly integral to modern communication, the need for high-quality synchronization between speech and visual elements is paramount. Speech Activity Detection (VAD) and lip synchronization technologies play crucial roles in ensuring accurate, real-time communication by distinguishing speech signals from noise and aligning lip movements with audio. This paper proposes a novel multimodal fusion approach based on deep learning models that significantly improves the accuracy of speech activity detection and the real-time performance of lip synchronization. Using open datasets such as AVSpeech and LRW, this study showcases the effectiveness of the proposed models in various real-world scenarios, such as multi-party conferences, noisy environments, and cross-lingual settings. Experimental results demonstrate that the LSTM-based VAD model achieves an accuracy of 92%, outperforming traditional methods, while the lip synchronization module ensures seamless audio-visual alignment with minimal delay.
References
[1] Ephrat, A., & Sandler, M. (2018). AVSpeech: A Large-Scale Audio-Visual Dataset for Speech Recognition. Proceedings of Interspeech.
[2] Lee, W., Seong, J. J., Ozlu, B., Shim, B. S., Marakhimov, A., & Lee, S. (2021). Biosignal sensors and deep learning-based speech recognition: A review. Sensors, 21(4), 1399.Alshahrani, M. H., & Maashi, M. S. (2024). A Systematic Literature Review: Facial Expression and Lip Movement Synchronization of an Audio Track. IEEE Access.
[3] Jha, A., Voleti, V., Namboodiri, V., & Jawahar, C. V. (2019, May). Cross-language speech dependent lip-synchronization. In ICASSP 2019-2019 IEEE International Conference on Acoustics, Speech and Signal Processing (ICASSP) (pp. 7140-7144). IEEE.
[4] Naebi, A., & Feng, Z. (2023). The Performance of a Lip-Sync Imagery Model, New Combinations of Signals, a Supplemental Bond Graph Classifier, and Deep Formula Detection as an Extraction and Root Classifier for Electroencephalograms and Brain–Computer Interfaces. Applied Sciences, 13(21), 11787.
[5] Saenko, K., Livescu, K., Siracusa, M., Wilson, K., Glass, J., & Darrell, T. (2005, October). Visual speech recognition with loosely synchronized feature streams. In Tenth IEEE International Conference on Computer Vision (ICCV'05) Volume 1 (Vol. 2, pp. 1424-1431). IEEE.
[6] Lyu, S. (2024). The Application of Generative AI in Virtual Reality and Augmented Reality. Journal of Industrial Engineering and Applied Science, 2(6), 1-9.
[7] Michelsanti, D., Tan, Z. H., Zhang, S. X., Xu, Y., Yu, M., Yu, D., & Jensen, J. (2021). An overview of deep-learning-based audio-visual speech enhancement and separation. IEEE/ACM Transactions on Audio, Speech, and Language Processing, 29, 1368-1396.
[8] Lyu, S. (2024). The Technology of Face Synthesis and Editing Based on Generative Models. Journal of Computer Technology and Applied Mathematics, 1(4), 21-27.
[9] Zaki, M. M., & Shaheen, S. I. (2011). Sign language recognition using a combination of new vision based features. Pattern Recognition Letters, 32(4), 572-577.
[10] Lyu, S. (2024). Machine Vision-Based Automatic Detection for Electromechanical Equipment. Journal of Computer Technology and Applied Mathematics, 1(4), 12-20.
[11] Rao, G. A., Syamala, K., Kishore, P. V. V., & Sastry, A. S. C. S. (2018, January). Deep convolutional neural networks for sign language recognition. In 2018 conference on signal processing and communication engineering systems (SPACES) (pp. 194-197). IEEE.
[12] Lin, W. (2024). A Review of Multimodal Interaction Technologies in Virtual Meetings. Journal of Computer Technology and Applied Mathematics, 1(4), 60-68.
[13] Ahmad, R., Zubair, S., & Alquhayz, H. (2020). Speech enhancement for multimodal speaker diarization system. IEEE Access, 8, 126671-126680.
[14] Luo, M., Zhang, W., Song, T., Li, K., Zhu, H., Du, B., & Wen, H. (2021, January). Rebalancing expanding EV sharing systems with deep reinforcement learning. In Proceedings of the Twenty-Ninth International Conference on International Joint Conferences on Artificial Intelligence (pp. 1338-1344).
[15] Lin, W. (2024). A Systematic Review of Computer Vision-Based Virtual Conference Assistants and Gesture Recognition. Journal of Computer Technology and Applied Mathematics, 1(4), 28-35.
[16] Luo, M., Du, B., Zhang, W., Song, T., Li, K., Zhu, H., ... & Wen, H. (2023). Fleet rebalancing for expanding shared e-Mobility systems: A multi-agent deep reinforcement learning approach. IEEE Transactions on Intelligent Transportation Systems, 24(4), 3868-3881.
[17] Zhu, H., Luo, Y., Liu, Q., Fan, H., Song, T., Yu, C. W., & Du, B. (2019). Multistep flow prediction on car-sharing systems: A multi-graph convolutional neural network with attention mechanism. International Journal of Software Engineering and Knowledge Engineering, 29(11n12), 1727–1740.
[18] Li, K., Chen, X., Song, T., Zhang, H., Zhang, W., & Shan, Q. (2024). GPTDrawer: Enhancing Visual Synthesis through ChatGPT. arXiv preprint arXiv:2412.10429.
[19] Xu, Y., Lin, Y. S., Zhou, X., & Shan, X. (2024). Utilizing emotion recognition technology to enhance user experience in real-time. Computing and Artificial Intelligence, 2(1), 1388-1388.
[20] Lavagetto, F. (1997). Time-delay neural networks for estimating lip movements from speech analysis: A useful tool in audio-video synchronization. IEEE Transactions on Circuits and systems for Video Technology, 7(5), 786-800.
[21] Li, K., Liu, L., Chen, J., Yu, D., Zhou, X., Li, M., ... & Li, Z. (2024, November). Research on reinforcement learning based warehouse robot navigation algorithm in complex warehouse layout. In 2024 6th International Conference on Artificial Intelligence and Computer Applications (ICAICA) (pp. 296-301). IEEE.
[22] Sohn, J. W., & Lee, W. (1999). Energy-based Voice Activity Detection for Noisy Environments. IEEE Transactions on Speech and Audio Processing.
[23] Li, K., Chen, J., Yu, D., Dajun, T., Qiu, X., Lian, J., ... & Han, J. (2024, October). Deep reinforcement learning-based obstacle avoidance for robot movement in warehouse environments. In 2024 IEEE 6th International Conference on Civil Aviation Safety and Information Technology (ICCASIT) (pp. 342-348). IEEE.
[24] Sun, Y., & Ortiz, J. (2024). Machine Learning-Driven Pedestrian Recognition and Behavior Prediction for Enhancing Public Safety in Smart Cities. Journal of Artificial Intelligence and Information, 1, 51-57.
[25] Huang, X., Wu, Y., Zhang, D., Hu, J., & Long, Y. (2024, September). Improving Academic Skills Assessment with NLP and Ensemble Learning. In 2024 IEEE 7th International Conference on Information Systems and Computer Aided Education (ICISCAE) (pp. 37-41). IEEE.
[26] Yu, D., Liu, L., Wu, S., Li, K., Wang, C., Xie, J., ... & Ji, R. (2024). Machine learning optimizes the efficiency of picking and packing in automated warehouse robot systems. In 2024 International Conference on Computer Engineering, Network and Digital Communication (CENDC 2024).
[27] Ahmad, R., Zubair, S., Alquhayz, H., & Ditta, A. (2019). Multimodal speaker diarization using a pre-trained audio-visual synchronization model. Sensors, 19(23), 5163.Rabiner, L. R., & Schafer, R. (1978). Digital Processing of Speech Signals. Prentice-Hall.
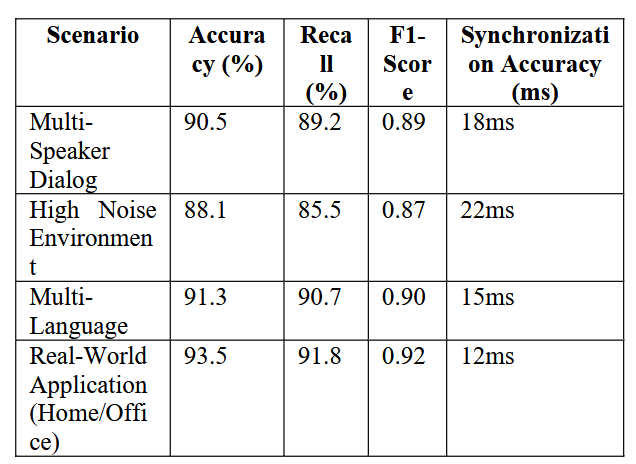
Downloads
Published
How to Cite
Issue
Section
ARK
License
Copyright (c) 2025 The author retains copyright and grants the journal the right of first publication.

This work is licensed under a Creative Commons Attribution 4.0 International License.