Anomalous Payment Behavior Detection and Risk Prediction for SMEs Based on LSTM-Attention Mechanism
DOI:
https://doi.org/10.70393/616a736d.323733ARK:
https://n2t.net/ark:/40704/AJSM.v3n2a05Disciplines:
BusinessSubjects:
FinanceReferences:
8Keywords:
LSTM-Attention Mechanism, Payment Anomaly Detection, Financial Risk Prediction, SME Risk ManagementAbstract
This paper proposes a novel approach for detecting anomalous payment behaviors and predicting financial risks in Small and Medium-sized Enterprises (SMEs) using an enhanced LSTM-Attention mechanism. The model integrates bi-directional LSTM networks with a multi-head attention mechanism to capture complex temporal dependencies in payment patterns while focusing on significant transaction features. The approach addresses the challenges of imbalanced datasets and evolving payment behaviors through a comprehensive risk assessment framework and dynamic threshold adjustment mechanism. Experimental results on a dataset containing 2.85 million transactions from 7,500 SMEs demonstrate the model's superior performance, achieving 98.5% accuracy and 94.2% precision in anomaly detection. The proposed model significantly outperforms traditional approaches and contemporary deep learning methods, showing a 15-20% improvement in detection accuracy while maintaining low false positive rates. The integration of behavioral risk indicators with operational metrics enables early risk prediction with an AUC-ROC score of 0.982. The model's effectiveness is validated through extensive case studies across various industry sectors, demonstrating robust generalization capabilities and practical applicability in real-world scenarios. The research contributes to the field by introducing an adaptive risk assessment framework that combines temporal pattern analysis with contextual business information for enhanced payment risk detection.
Downloads
Metrics
References
[1] Xu, J., Chen, H., Xiao, X., Zhao, M., Liu, B. (2025). Gesture Object Detection and Recognition Based on YOLOv11.Applied and Computational Engineering,133,81-89.
[2] Chen, H., Shen, Z., Wang, Y. and Xu, J., 2024. Threat Detection Driven by Artificial Intelligence: Enhancing Cybersecurity with Machine Learning Algorithms.
[3] Liang, X., & Chen, H. (2019, July). A SDN-Based Hierarchical Authentication Mechanism for IPv6 Address. In 2019 IEEE International Conference on Intelligence and Security Informatics (ISI) (pp. 225-225). IEEE.
[4] Liang, X., & Chen, H. (2019, August). HDSO: A High-Performance Dynamic Service Orchestration Algorithm in Hybrid NFV Networks. In 2019 IEEE 21st International Conference on High Performance Computing and Communications; IEEE 17th International Conference on Smart City; IEEE 5th International Conference on Data Science and Systems (HPCC/SmartCity/DSS) (pp. 782-787). IEEE.
[5] Chen, H., & Bian, J. (2019, February). Streaming media live broadcast system based on MSE. In Journal of Physics: Conference Series (Vol. 1168, No. 3, p. 032071). IOP Publishing.
[6] Ke, Z., Zhou, S., Zhou, Y., Chang, C. H., & Zhang, R. (2025). Detection of AI Deepfake and Fraud in Online Payments Using GAN-Based Models. arXiv preprint arXiv:2501.07033.
[7] Yu, Q., Ke, Z., Xiong, G., Cheng, Y., & Guo, X. (2025). Identifying Money Laundering Risks in Digital Asset Transactions Based on AI Algorithms.
[8] Ke, Z., Xu, J., Zhang, Z., Cheng, Y., & Wu, W. (2024). A Consolidated Volatility Prediction with Back Propagation Neural Network and Genetic Algorithm. arXiv preprint arXiv:2412.07223.
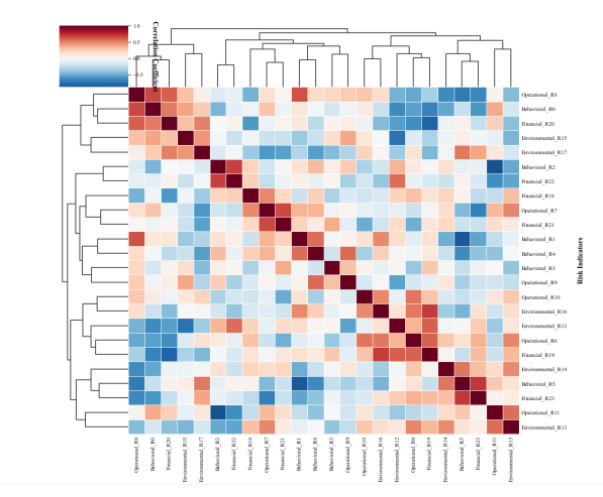
Downloads
Published
How to Cite
Issue
Section
ARK
License
Copyright (c) 2025 The author retains copyright and grants the journal the right of first publication.

This work is licensed under a Creative Commons Attribution 4.0 International License.