Research on Emotionally Intelligent Dialogue Generation based on Automatic Dialogue System
DOI:
https://doi.org/10.5281/zenodo.10864022References:
6Keywords:
Natural Language Processing (NLP) Technology, Emotional Analysis Techniques, DMS, Generative Model, Emotional Expression Technology, Machine Learning And Deep Learning Techniques, User Modeling And Emotion Tracking, Pain Empathy In AIAbstract
Automated dialogue systems are important applications of artificial intelligence, and traditional systems struggle to understand user emotions and provide empathetic feedback. This study integrates emotional intelligence technology into automated dialogue systems and creates a dialogue generation model with emotional intelligence through deep learning and natural language processing techniques. The model can detect and understand a wide range of emotions and specific pain signals in real time, enabling the system to provide empathetic interaction. By integrating the results of the study "Can artificial intelligence detect pain and express pain empathy?", the model's ability to understand the subtle elements of pain empathy has been enhanced, setting higher standards for emotional intelligence dialogue systems. The project aims to provide theoretical understanding and practical suggestions to integrate advanced emotional intelligence capabilities into dialogue systems, thereby improving user experience and interaction quality.
References
Zhao, Y., Cheng, B., Huang, Y., & Wan, Z. (2023). Beyond words: An intelligent human-machine dialogue system with multimodal generation and emotional comprehension. International Journal of Intelligent Systems, 2023.
Huang, C., Zaiane, O. R., Trabelsi, A., & Dziri, N. (2018, June). Automatic dialogue generation with expressed emotions. In Proceedings of the 2018 Conference of the North American Chapter of the Association for Computational Linguistics: Human Language Technologies, Volume 2 (Short Papers), 49-54.
Zang, H. (2024). Precision calibration of industrial 3D scanners: An AI-enhanced approach for improved measurement accuracy. Global Academic Frontiers, 2(1), 27-37.
Ni, F., Zang, H., & Qiao, Y. (2024, January). Smartfix: Leveraging machine learning for proactive equipment maintenance in industry 4.0. In The 2nd International scientific and practical conference “Innovations in education: Prospects and challenges of today” (January 16-19, 2024). Sofia, Bulgaria. International Science Group. 2024. 389 p. (p. 313).
Zang, H., Li, S., Dong, X., Ma, D., & Dang, B. (2024). Evaluating the social impact of AI in manufacturing: A methodological framework for ethical production. Academic Journal of Sociology and Management, 2(1), 21–25. https://doi.org/10.5281/zenodo.10474511
Ma, D., Dang, B., Li, S., Zang, H., & Dong, X. (2023). Implementation of computer vision technology based on artificial intelligence for medical image analysis. International Journal of Computer Science and Information Technology, 1(1), 69-76. https://doi.org/10.62051/ijcsit.v1n1.10
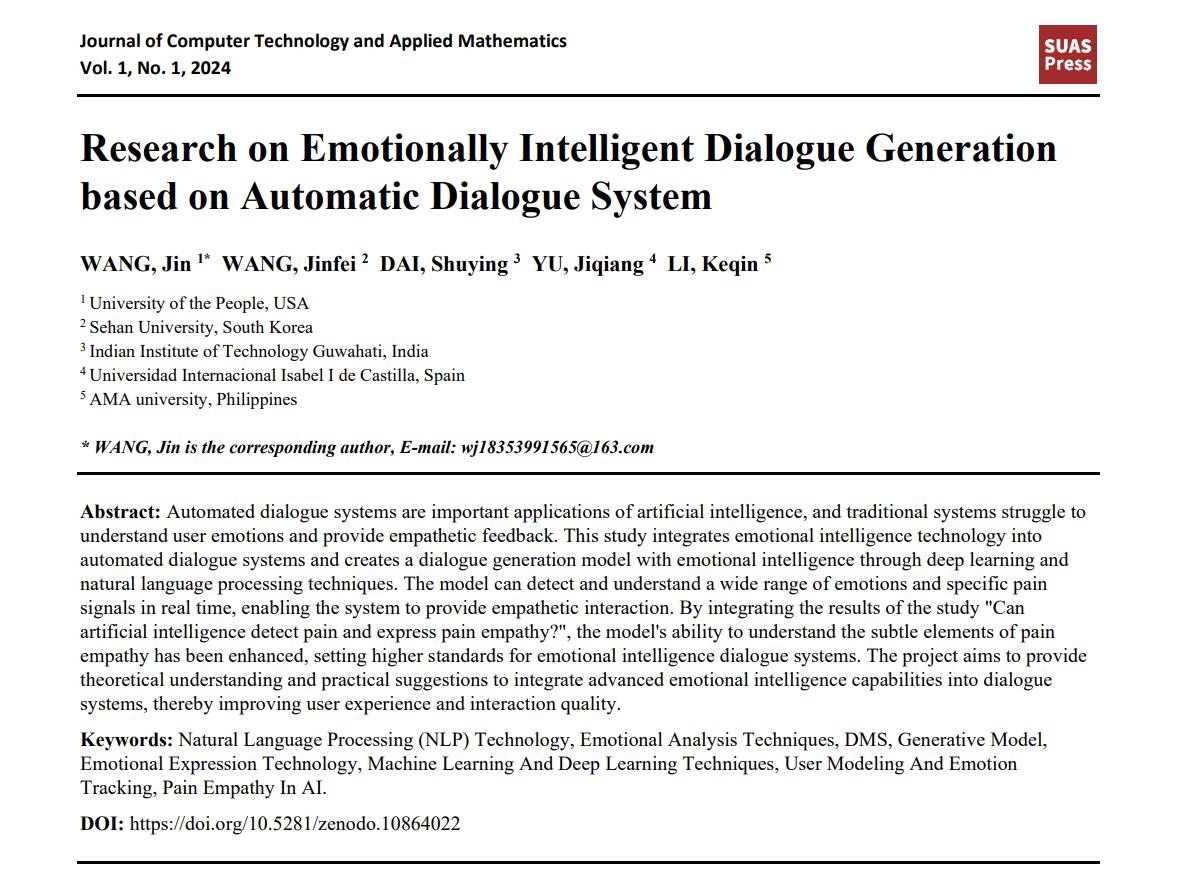
Downloads
Published
How to Cite
Issue
Section
License
Copyright (c) 2024 Copyright reserved by the author.

This work is licensed under a Creative Commons Attribution 4.0 International License.