Scenarios Driving Economic Forecasts: Choosing Econometrics or Machine Learning
DOI:
https://doi.org/10.5281/zenodo.10927145References:
22Keywords:
Economic Forecasting, Machine Learning, Econometrics, Economic Forecasting Scenarios, Time Series Models, Advanced Regression ModelsAbstract
Economic forecasting is an in-depth understanding of the laws of economic operation. It is based on historical facts and past data to make possible inferences about future economic trends and development trends in related fields. Since the main operating rules of economic activities are different and contain randomness, economic forecast is not a deterministic forecast, but a possibility forecast. Therefore, there are various economic forecasting methods, and different forecasting methods are suitable for different application scenarios. This paper aims to explore the applicability of econometric forecasting methods and machine learning methods in different application scenarios in scenario-driven economic forecasting. For macroeconomic forecasting, the ARIMA model and VAR vector autoregressive model can process time series data and are suitable for predicting macroeconomic indicators. For industry economic forecasting, random forest regression and Adaboost regression can handle large amounts of data and complex data structures, and are suitable for predicting the development trends of specific industries. For product economic forecasting, linear regression and decision trees are capable of handling continuous-valued forecasts and are suitable for forecasting demand and price for a specific product or service. For prediction of the impact of major events, double difference DID (difference-in-difference method) can avoid the endogeneity problem of data to a large extent and is suitable for evaluating policy effects.
References
Elliott, G., & Timmermann, A. (2008). Economic forecasting. Journal of Economic Literature, 46(1), 3-56.
Mincer, J. A., & Zarnowitz, V. (1969). The evaluation of economic forecasts. In Economic forecasts and expectations: Analysis of forecasting behavior and performance (pp. 3-46). NBER.
Clements, M., & Hendry, D. (2002). An overview of economic forecasting. A Companion to Economic Forecasting. Oxford: Blackwell, 1-18.
Clemen, R. T., & Winkler, R. L. (1986). Combining economic forecasts. Journal of Business & Economic Statistics, 4(1), 39-46.
Makridakis, S., & Bakas, N. (2016). Forecasting and uncertainty: A survey. Risk and Decision Analysis, 6(1), 37-64..
Fildes, R., & Stekler, H. (2002). The state of macroeconomic forecasting. Journal of macroeconomics, 24(4), 435-468.
Diebold, F. X. (1998). The past, present, and future of macroeconomic forecasting. Journal of Economic Perspectives, 12(2), 175-192..
D'Agostino, A., Gambetti, L., & Giannone, D. (2013). Macroeconomic forecasting and structural change. Journal of applied econometrics, 28(1), 82-101.
Tsai, M. C., Cheng, C. H., Tsai, M. I., & Shiu, H. Y. (2018). Forecasting leading industry stock prices based on a hybrid time-series forecast model. PloS one, 13(12), e0209922.
Batchelor, R. A., & Dua, P. (1990). Product differentiation in the economic forecasting industry. International Journal of Forecasting, 6(3), 311-316.
Lai, Y., & Dzombak, D. A. (2020). Use of the autoregressive integrated moving average (ARIMA) model to forecast near-term regional temperature and precipitation. Weather and Forecasting, 35(3), 959-976.
Nyoni, T. (2018). Modeling and forecasting inflation in zimbabwe: a generalized autoregressive conditionally heteroskedastic (GARCH) approach..
Lütkepohl, H. (2013). Vector autoregressive models. In Handbook of research methods and applications in empirical macroeconomics (pp. 139-164). Edward Elgar Publishing..
El-Melegy, M. T. (2014). Model-wise and point-wise random sample consensus for robust regression and outlier detection. Neural networks, 59, 23-35..
Zhou, G., Liu, C., & Luo, S. (2021). Resource allocation effect of green credit policy: based on DID model. Mathematics, 9(2), 159.
Shetty, S. H., Shetty, S., Singh, C., & Rao, A. (2022). Supervised machine learning: algorithms and applications. Fundamentals and methods of machine and deep learning: algorithms, tools and applications, 1-16.
Uyanık, G. K., & Güler, N. (2013). A study on multiple linear regression analysis. Procedia-Social and Behavioral Sciences, 106, 234-240.
Scientific Platform Serving for Statistics Professional 2021. SPSSPRO. (Version 1.0.11) [Online Application Software]. Retrieved from https://www.spsspro.com.
Apté, C., & Weiss, S. (1997). Data mining with decision trees and decision rules. Future generation computer systems, 13(2-3), 197-210.
Xue, L., Liu, Y., Xiong, Y., Liu, Y., Cui, X., & Lei, G. (2021). A data-driven shale gas production forecasting method based on the multi-objective random forest regression. Journal of Petroleum Science and Engineering, 196, 107801.
Wang, F., Li, Z., He, F., Wang, R., Yu, W., & Nie, F. (2019). Feature learning viewpoint of AdaBoost and a new algorithm. IEEE Access, 7, 149890-149899.
Jun, M. J. (2021). A comparison of a gradient boosting decision tree, random forests, and artificial neural networks to model urban land use changes: The case of the Seoul metropolitan area. International Journal of Geographical Information Science, 35(11), 2149-2167.
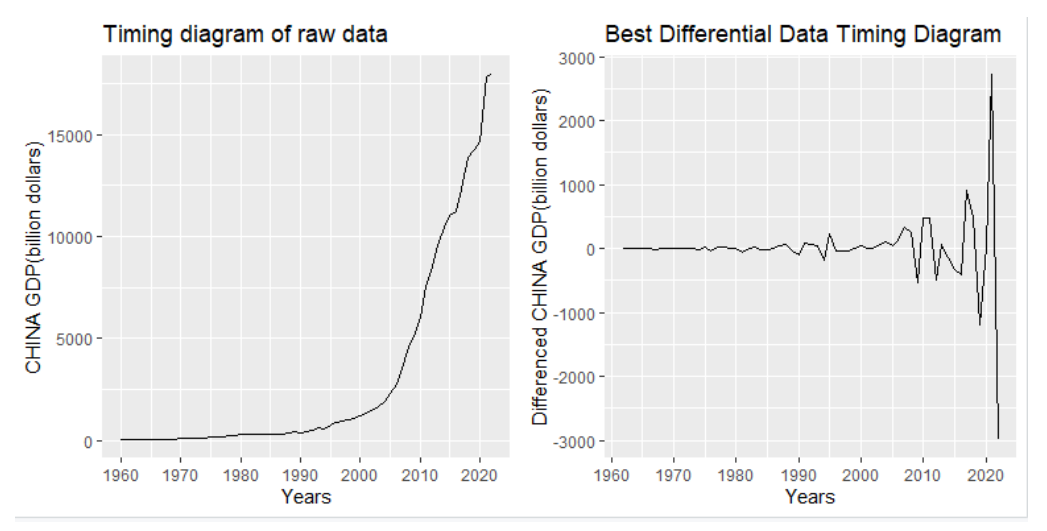
Downloads
Published
How to Cite
Issue
Section
License
Copyright (c) 2024 Copyright reserved by the author.

This work is licensed under a Creative Commons Attribution 4.0 International License.