Hybrid Deep Learning for AI-Based Financial Time Series Prediction
DOI:
https://doi.org/10.5281/zenodo.10938270References:
25Keywords:
Financial Time Series Forecasting, Random Forest Model, Machine Learning, Deep LearningAbstract
As the influencing factors of the development of things are not clear or the data collection is difficult, many forecasting problems have evolved into univariate time series forecasting problems, that is, mining its own inherent laws from the past time series data, so as to predict its future development trend. The purpose of this study is to explore the feasibility of using random forest classifier to predict the long-term trend of stocks. By analyzing data sets such as Apple, Samsung, and General Electric, we built a random forest model and found that its prediction accuracy was 85 to 95 percent. With the increase of the number of decision trees, the prediction results of the model tend to be stable, indicating that increasing the number of decision trees can improve the prediction accuracy. In addition, we point out that the method is also suitable for short-term trend forecasting, and suggest training with more fine-grained transaction data to improve forecasting accuracy. Finally, we look forward to the potential of the field of artificial intelligence in time series forecasting, emphasizing that the application of technologies such as deep learning will further improve forecasting accuracy and provide more reliable decision support for financial investors.
References
Li, Zhenglin, et al. "Feature Manipulation for DDPM based Change Detection." arXiv preprint arXiv:2403.15943 (2024).
Xu, J., Wu, B., Huang, J., Gong, Y., Zhang, Y., & Liu, B. (2024). Practical Applications of Advanced Cloud Services and Generative AI Systems in Medical Image Analysis. arXiv preprint arXiv:2403.17549.
Zhu, Mengran, et al. "Ensemble Methodology: Innovations in Credit Default Prediction Using LightGBM, XGBoost, and LocalEnsemble." arXiv preprint arXiv:2402.17979 (2024).
Zhang, Y., Liu, B., Gong, Y., Huang, J., Xu, J., & Wan, W. (2024). Application of Machine Learning Optimization in Cloud Computing Resource Scheduling and Management. arXiv preprint arXiv:2402.17216.
Gong, Y., Huang, J., Liu, B., Xu, J., Wu, B., & Zhang, Y. (2024). Dynamic Resource Allocation for Virtual Machine Migration Optimization using Machine Learning. arXiv preprint arXiv:2403.13619.
Liu, B. (2023). Based on intelligent advertising recommendation and abnormal advertising monitoring system in the field of machine learning. International Journal of Computer Science and Information Technology, 1(1), 17-23.
Wu, Binbin, et al. "Enterprise Digital Intelligent Remote Control System Based on Industrial Internet of Things." (2024).
Pan, Y., Wu, B., Zheng, H., Zong, Y., & Wang, C. (2024, March). THE APPLICATION OF SOCIAL MEDIA SENTIMENT ANALYSIS BASED ON NATURAL LANGUAGE PROCESSING TO CHARITY. In The 11th International scientific and practical conference “Advanced technologies for the implementation of educational initiatives”(March 19–22, 2024) Boston, USA. International Science Group. 2024. 254 p. (p. 216).
K. Xu, X. Wang, Z. Hu and Z. Zhang, "3D Face Recognition Based on Twin Neural Network Combining Deep Map and Texture," 2019 IEEE 19th International Conference on Communication Technology (ICCT), Xi'an, China, 2019, pp. 1665-1668, doi: 10.1109/ICCT46805.2019.8947113.
Shi, Peng, Yulin Cui, Kangming Xu, Mingmei Zhang, and Lianhong Ding. 2019. "Data Consistency Theory and Case Study for Scientific Big Data" Information 10, no. 4: 137. https://doi.org/10.3390/info10040137.
Zhenghua Hu, Xianmei Wang, Kangming Xu, and Pu Dong. 2020. Real-time Target Tracking Based on PCANet-CSK Algorithm. In Proceedings of the 2019 3rd International Conference on Computer Science and Artificial Intelligence (CSAI '19). Association for Computing Machinery, New York, NY, USA, 343–346. https://doi.org/10.1145/3374587.3374607.
Medication Recommendation System Based on Natural Language Processing for Patient Emotion Analysis. (2024). Academic Journal of Science and Technology, 10(1), 62-68. https://doi.org/10.54097/v160aa61
Li, X., Zheng, H., Chen, J., Zong, Y., & Yu, L. (2024). User Interaction Interface Design and Innovation Based on Artificial Intelligence Technology. Journal of Theory and Practice of Engineering Science, 4(03), 1-8.
Xu, K., Zhou, H., Zheng, H., Zhu, M., & Xin, Q. (2024). Intelligent Classification and Personalized Recommendation of E-commerce Products Based on Machine Learning. arXiv preprint arXiv:2403.19345.
Zhou, H., Xu, K., Bao, Q., Lou, Y., & Qian, W. (2024). Application of Conversational Intelligent Reporting System Based on Artificial Intelligence and Large Language Models. Journal of Theory and Practice of Engineering Science, 4(03), 176-182.
Li, L., Xu, K., Zhou, H., & Wang, Y. (2024). Independent Grouped Information Expert Model: A Personalized Recommendation Algorithm Based on Deep Learning.
Che, C., Lin, Q., Zhao, X., Huang, J., & Yu, L. (2023, September). Enhancing Multimodal Understanding with CLIP-Based Image-to-Text Transformation. In Proceedings of the 2023 6th International Conference on Big Data Technologies (pp. 414-418).
Huang, Zengyi, et al. "Research on Generative Artificial Intelligence for Virtual Financial Robo-Advisor." Academic Journal of Science and Technology 10.1 (2024): 74-80.
Huang, Zengyi, et al. "Application of Machine Learning-Based K-Means Clustering for Financial Fraud Detection." Academic Journal of Science and Technology 10.1 (2024): 33-39.
Wu, Y., Jin, Z., Shi, C., Liang, P., & Zhan, T. (2024). Research on the Application of Deep Learning-based BERT Model in Sentiment Analysis. arXiv preprint arXiv:2403.08217.
Shi, C., Liang, P., Wu, Y., Zhan, T., & Jin, Z. (2024). Maximizing User Experience with LLMOps-Driven Personalized Recommendation Systems. arXiv preprint arXiv:2404.00903.
Huang, Zengyi, et al. "Application of Machine Learning-Based K-Means Clustering for Financial Fraud Detection." Academic Journal of Science and Technology 10.1 (2024): 33-39.
Xu, Z., Gong, Y., Zhou, Y., Bao, Q., & Qian, W. (2024). Enhancing Kubernetes Automated Scheduling with Deep Learning and Reinforcement Techniques for Large-Scale Cloud Computing Optimization. arXiv preprint arXiv:2403.07905.
Xu, X., Xu, Z., Ling, Z., Jin, Z., & Du, S. (2024). Comprehensive Implementation of TextCNN for Enhanced Collaboration between Natural Language Processing and System Recommendation. arXiv preprint arXiv:2403.09718.
Song, B., Xu, Y., & Wu, Y. (2024). ViTCN: Vision Transformer Contrastive Network For Reasoning. arXiv preprint arXiv:2403.09962.
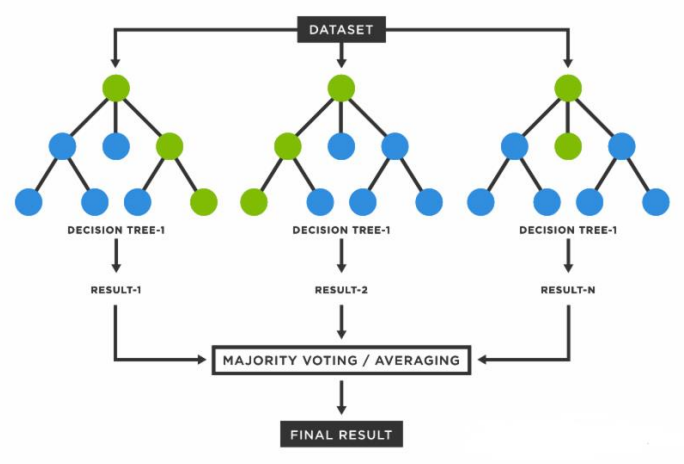
Downloads
Published
How to Cite
Issue
Section
License
Copyright (c) 2024 Copyright reserved by the author.

This work is licensed under a Creative Commons Attribution 4.0 International License.