A Comprehensive Review of Reinforcement Learning in Intelligent Allocation and Optimization of Educational Resources
DOI:
https://doi.org/10.70393/6a6374616d.323436ARK:
https://n2t.net/ark:/40704/JETBM.v1n6a03Disciplines:
Artificial Intelligence and IntelligenceSubjects:
Machine LearningReferences:
20Keywords:
Reinforcement Learning, Educational Resource Optimization, Equity in Education, Multi-Agent Systems, Personalized Learning, Resource Scheduling, Hybrid AI Approaches, Educational Data QualityAbstract
Educational resource imbalances pose considerable barriers to accomplishing equitable opportunities to learn worldwide. Traditional approaches to resource allocation frequently fail to adapt to the ever-changing and intricate needs of educational systems, exacerbating disparities. This paper explores applying reinforcement learning (RL) in optimizing how resources in education are distributed and used, offering a promising solution to these hurdles. The analysis delves into fundamental RL concepts and algorithms, like deep reinforcement learning and multi-agent reinforcement learning, and investigates their applications in customized learning, scheduling resources, and promoting fairness. It highlights major difficulties such as information quality, scalability, fairness, and transparency, along with possibilities for innovation through blended methodologies and instant decision making. By combining existing research and distinguishing critical gaps, this study provides practical insights for advancing RL applications in education, paving the way for more inclusive and effective systems for managing resources.
References
Grigsby, J., Fan, L., & Zhu, Y. (2023). Amago: Scalable in-context reinforcement learning for adaptive agents. arXiv preprint arXiv:2310.09971.
Hafiz, A. M., Hassaballah, M., Alqahtani, A., Alsubai, S., & Hameed, M. A. (2023). Reinforcement Learning with an Ensemble of Binary Action Deep Q-Networks. Computer Systems Science & Engineering, 46(3).
Tefera, M. K., Zhang, S., & Jin, Z. (2023). Deep reinforcement learning-assisted optimization for resource allocation in downlink OFDMA cooperative systems. Entropy, 25(3), 413.
Sun, Q., Qiu, Z., Ye, H., & Wan, Z. (2019, February). Multinational Corporation Location Plan under Multiple Factors. In Journal of Physics: Conference Series (Vol. 1168, No. 3, p. 032012). IOP Publishing.
De Giacomo, G., Favorito, M., Leotta, F., Mecella, M., & Silo, L. (2023). Digital twin composition in smart manufacturing via Markov decision processes. Computers in Industry, 149, 103916.
He, Z. (2024). An Empirical Analysis of the Factors Influencing Commodity Housing Prices in China Based on Econometrics. In INTERNET FINANCE AND DIGITAL ECONOMY: Advances in Digital Economy and Data Analysis Technology The 2nd International Conference on Internet Finance and Digital Economy, Kuala Lumpur, Malaysia, 19–21 August 2022 (pp. 99-112).
Sun, Y., Salami Pargoo, N., Jin, P., & Ortiz, J. (2024, October). Optimizing Autonomous Driving for Safety: A Human-Centric Approach with LLM-Enhanced RLHF. In Companion of the 2024 on ACM International Joint Conference on Pervasive and Ubiquitous Computing (pp. 76-80).
Silver, N., Kaplan, M., LaVaque-Manty, D., & Meizlish, D. (Eds.). (2023). Using reflection and metacognition to improve student learning: Across the disciplines, across the academy. Taylor & Francis.
Jeyaraman, J., Krishnamoorthy, G., Kumar Konidena, B., & Sistla, S. M. K. (2024). Machine learning for demand forecasting in manufacturing. International Journal for Multidisciplinary Research, 6(1), 1-115.
Mitra, R., McGough, S. F., Chakraborti, T., Holmes, C., Copping, R., Hagenbuch, N., ... & MacArthur, B. D. (2023). Learning from data with structured missingness. Nature Machine Intelligence, 5(1), 13-23.
Lu, J. (2024). Optimizing e-commerce with multi-objective recommendations using ensemble learning.
Lu, J., Long, Y., Li, X., Shen, Y., & Wang, X. (2024). Hybrid Model Integration of LightGBM, DeepFM, and DIN for Enhanced Purchase Prediction on the Elo Dataset. Preprints. https://doi.org/10.20944/preprints202411.1053.v1
Zhang, Y., Wang, F., Huang, X., Li, X., Liu, S., & Zhang, H. (2024). Optimization and Application of Cloud-based Deep Learning Architecture for Multi-Source Data Prediction. arXiv preprint arXiv:2410.12642.
Cao, H., Zhang, Z., Li, X., Wu, C., Zhang, H., & Zhang, W. (2024). Mitigating Knowledge Conflicts in Language Model-Driven Question Answering. arXiv preprint arXiv:2411.11344.
Huang, X., Wu, Y., Zhang, D., Hu, J., & Long, Y. (2024). Improving Academic Skills Assessment with NLP and Ensemble Learning. arXiv preprint arXiv:2409.19013.
Kang, Y., Song, Y., & Huang, S. (2024). Tie memories to e-souvenirs: Personalized souvenirs with augmented reality for interactive learning in the museum.
Gu, Y., Liu, B., Zhang, T., Sha, X., & Chen, S. (2024). Architectural Synergies in Bi-Modal and Bi-Contrastive Learning. IEEE Access.
Su, Y., Sun, Y., Zhang, M., & Wang, J. (2024). Vexless: A Serverless Vector Data Management System Using Cloud Functions. Proceedings of the ACM on Management of Data, 2(3), 1-26.
Lin, W. (2024). A Review of Multimodal Interaction Technologies in Virtual Meetings. Journal of Computer Technology and Applied Mathematics, 1(4), 60-68.
Lin, W. (2024). A Systematic Review of Computer Vision-Based Virtual Conference Assistants and Gesture Recognition. Journal of Computer Technology and Applied Mathematics, 1(4), 28-35.
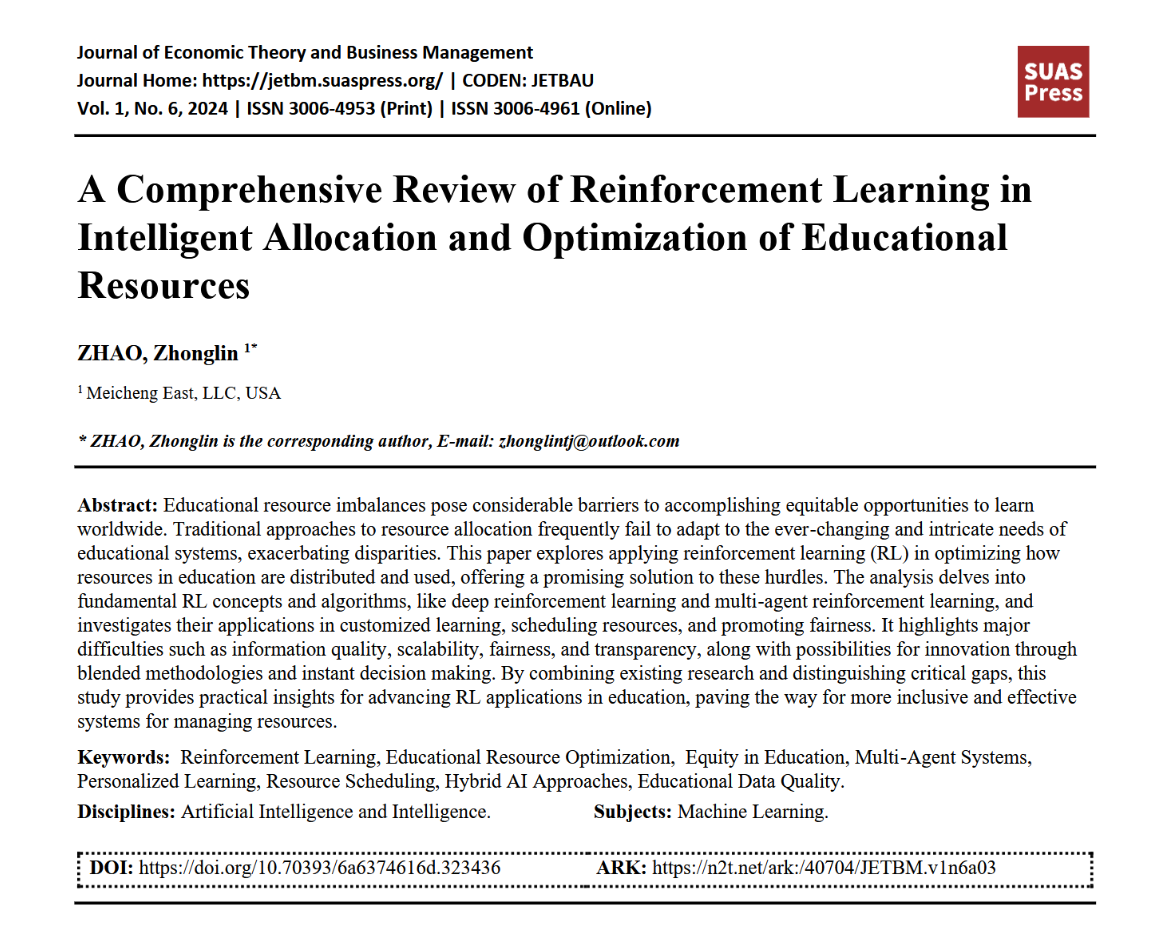
Downloads
Published
How to Cite
Issue
Section
ARK
License
Copyright (c) 2024 The author retains copyright and grants the journal the right of first publication.

This work is licensed under a Creative Commons Attribution 4.0 International License.