Machine learning and Feature Selection: Applications in Business Management
DOI:
https://doi.org/10.70393/6a6574626d.323438ARK:
https://n2t.net/ark:/40704/JETBM.v1n6a05Disciplines:
BusinessSubjects:
Business StrategyReferences:
18Keywords:
Machine Learning, Feature Selection, Business ManagementAbstract
In recent years, we have a higher demand for machine learning models in the field of economics and business managment. We have a higher demand for quality of the features used for training. In this process, feature selection plays a key role in identifying the most meaningful features from a dataset while we perfrom various business tasks. Feature selection is not just a technical exercise; it also has profound implications for the transparency and explainability of machine learning models. This study aims to be a valuable resource for both academic and industry experts, offering insights that connect theoretical knowledge with practical implementation. And this paper also highlights the potential applications and significance of feature selection across industries like business, finance, and other real-world scenarios. And it aims to explore deep in the feature selection, showing that its impact on model performance and its role in various domains.
References
Che, C., & Tian, J. (2024). Game Theory: Concepts, Applications, and Insights from Operations Research. Journal of Computer Technology and Applied Mathematics, 1(4), 53-59.
Lee, I., & Shin, Y. J. (2020). Machine learning for enterprises: Applications, algorithm selection, and challenges. Business Horizons, 63(2), 157-170.
Che, C., & Tian, J. (2024). Maximum flow and minimum cost flow theory to solve the evacuation planning. Advances in Engineering Innovation, 12, 60-64.
Cheng, X. (2024). A Comprehensive Study of Feature Selection Techniques in Machine Learning Models.
Taha, A., Cosgrave, B., & Mckeever, S. (2022). Using feature selection with machine learning for generation of insurance insights. Applied Sciences, 12(6), 3209.
Chen, L. H., & Hsiao, H. D. (2008). Feature selection to diagnose a business crisis by using a real GA-based support vector machine: An empirical study. Expert systems with applications, 35(3), 1145-1155.
Cheng, X. (2024). Machine Learning-Driven Fraud Detection: Management, Compliance, and Integration. Academic Journal of Sociology and Management, 2(6), 8-13.
Di Mauro, M., Galatro, G., Fortino, G., & Liotta, A. (2021). Supervised feature selection techniques in network intrusion detection: A critical review. Engineering Applications of Artificial Intelligence, 101, 104216
Che, C., & Tian, J. (2024). Methods comparison for neural network-based structural damage recognition and classification. Advances in Operation Research and Production Management, 3, 20-26.
Zhao, Z., Anand, R., & Wang, M. (2019, October). Maximum relevance and minimum redundancy feature selection methods for a marketing machine learning platform. In 2019 IEEE international conference on data science and advanced analytics (DSAA) (pp. 442-452). IEEE.
Che, C., & Tian, J. (2024). Understanding the Interrelation Between Temperature and Meteorological Factors: A Case Study of Szeged Using Machine Learning Techniques. Journal of Computer Technology and Applied Mathematics, 1(4), 47-52.
Tian, J., & Che, C. (2024). Automated Machine Learning: A Survey of Tools and Techniques. Journal of Industrial Engineering and Applied Science, 2(6), 71-76.
Li, Y., Li, T., & Liu, H. (2017). Recent advances in feature selection and its applications. Knowledge and Information Systems, 53, 551-577.
Che, C., & Tian, J. (2024). Leveraging AI in Traffic Engineering to Enhance Bicycle Mobility in Urban Areas. Journal of Industrial Engineering and Applied Science, 2(6), 10-15.
Cheng, X. (2024). Investigations into the Evolution of Generative AI. Journal of Computer Technology and Applied Mathematics, 1(4), 117-122.
Bose, I., & Mahapatra, R. K. (2001). Business data mining—a machine learning perspective. Information & management, 39(3), 211-225.
Cheng, X., & Che, C. (2024). Optimizing Urban Road Networks for Resilience Using Genetic Algorithms. Academic Journal of Sociology and Management, 2(6), 1-7.
Cheng, X., & Che, C. (2024). Interpretable Machine Learning: Explainability in Algorithm Design. Journal of Industrial Engineering and Applied Science, 2(6), 65-70.
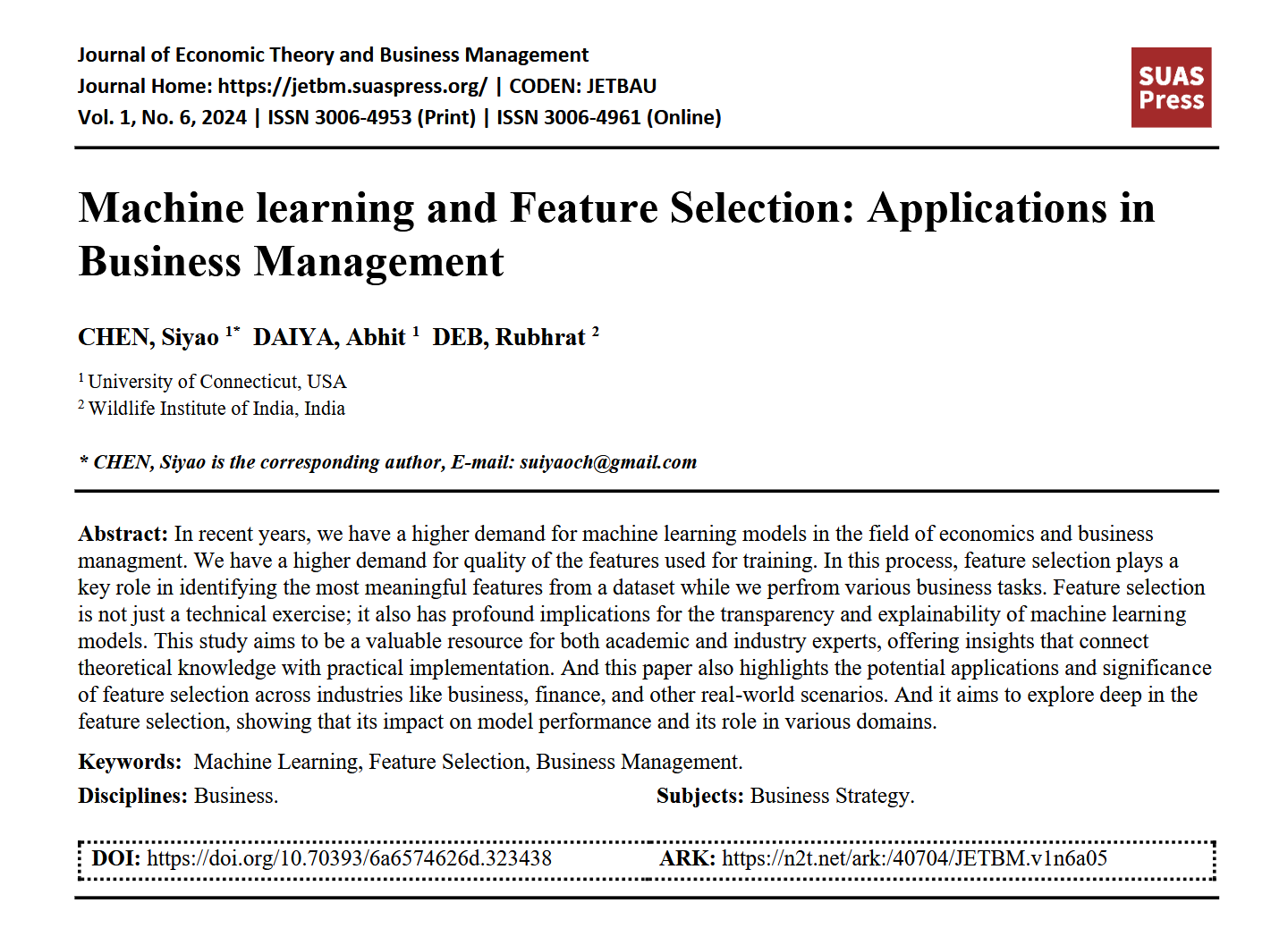
Downloads
Published
How to Cite
Issue
Section
ARK
License
Copyright (c) 2024 The author retains copyright and grants the journal the right of first publication.

This work is licensed under a Creative Commons Attribution 4.0 International License.