Big Data-Driven ESG Quantitative Investment Strategy
DOI:
https://doi.org/10.70393/6a6574626d.323837ARK:
https://n2t.net/ark:/40704/JETBM.v2n2a02Disciplines:
FinanceSubjects:
Investment BankingReferences:
15Keywords:
Big Data, ESG Investment, Quantitative Investment Strategy, Regression Analysis, Machine Learning, Data Analysis, Sustainable InvestmentAbstract
With sustainability becoming more important worldwide, investors are looking more closely at environmental, social, and governance (ESG) factors. This paper looks at how big data could help investors use ESG information effectively in quantitative investing. It discusses how the use of big data techniques can lead to more accurate and transparent ESG analyses. Using regression models, the study identifies a positive relationship between companies' ESG scores and their expected stock returns. It also illustrates how detailed big data analysis can enrich the evaluation of corporate ESG performance. Despite these advantages, the practical use of such methods still faces several significant hurdles. Data quality issues, a lack of standardized ESG metrics, and dynamic market conditions can undermine model accuracy and stability. To address these obstacles, we propose improved data cleaning procedures, the promotion of industry-wide ESG standards, and enhancements to model adaptability. Looking forward, new technologies such as artificial intelligence and blockchain are likely to help ESG investing become simpler and more efficient. Using these tools can make processing ESG data faster and clearer, giving investors stronger support when making decisions. In general, applying big data in ESG investing can help promote sustainability in financial markets and may also offer steady, long-term returns. Yet, there’s still uncertainty about how easily these technologies can actually be used in practice.
References
[1] Fu, L. (2021). The power of change — ESG "deep type" investment strategy in China. Contemporary Manager, (01), 19–20.
[2] Yang, X. (2022). Research on quantitative strategies based on investor sentiment (Master’s thesis, Central University of Finance and Economics). Central University of Finance and Economics. (Supervisors: Wei Xu & Li Ruiqiang)
[3] Xie, Y. (2022, December 22). Multi-factor driven private equity institutions focus on ESG investment. Financial Times, (006).
[4] ESG strategies: Fulfilling social responsibility and practicing long-term investment. (2021). Shanghai State-owned Assets, (07), 101–103.
[5] Tang, Y. (2022). Research on quantitative investment strategies based on public opinion data (Master’s thesis, Shandong University). Shandong University. (Supervisor: Shi Yufeng)
[6] Yu, D., Liu, L., Wu, S., Li, K., Wang, C., Xie, J., ... & Ji, R. (2024). Machine learning optimizes the efficiency of picking and packing in automated warehouse robot systems. In 2024 International Conference on Computer Engineering, Network and Digital Communication (CENDC 2024).
[7] Li, K., Liu, L., Chen, J., Yu, D., Zhou, X., Li, M., ... & Li, Z. (2024, November). Research on reinforcement learning based warehouse robot navigation algorithm in complex warehouse layout. In 2024 6th International Conference on Artificial Intelligence and Computer Applications (ICAICA) (pp. 296-301). IEEE.
[8] Li, K., Chen, J., Yu, D., Dajun, T., Qiu, X., Lian, J., ... & Han, J. (2024, October). Deep reinforcement learning-based obstacle avoidance for robot movement in warehouse environments. In 2024 IEEE 6th International Conference on Civil Aviation Safety and Information Technology (ICCASIT) (pp. 342-348). IEEE.
[9] Li, K., Wang, J., Wu, X., Peng, X., Chang, R., Deng, X., ... & Hong, B. (2024). Optimizing automated picking systems in warehouse robots using machine learning. arXiv preprint arXiv:2408.16633.
[10] Sun, J., Zhang, S., Lian, J., Fu, L., Zhou, Z., Fan, Y., & Xu, K. (2024, December). Research on Deep Learning of Convolutional Neural Network for Action Recognition of Intelligent Terminals in the Big Data Environment and its Intelligent Software Application. In 2024 IEEE 7th International Conference on Automation, Electronics and Electrical Engineering (AUTEEE) (pp. 996-1004). IEEE.
[11] Mao, Y., Tao, D., Zhang, S., Qi, T., & Li, K. (2025). Research and Design on Intelligent Recognition of Unordered Targets for Robots Based on Reinforcement Learning. arXiv preprint arXiv:2503.07340.
[12] Zuo, Q., Tao, D., Qi, T., Xie, J., Zhou, Z., Tian, Z., & Mingyu, Y. (2025). Industrial Internet Robot Collaboration System and Edge Computing Optimization. arXiv preprint arXiv:2504.02492.
[13] Zhang, T. (2025). Combining Blockchain and AI to Optimize the Intelligent Risk Control Mechanism in Decentralized Finance. Journal of Industrial Engineering and Applied Science, 3(2), 26-32.
[14] Zhou, Y., Shen, J., & Cheng, Y. (2025). Weak to strong generalization for large language models with multi-capabilities. In The Thirteenth International Conference on Learning Representations.
[15] Zhou, Y., Zhang, J., Chen, G., Shen, J., & Cheng, Y. (2024). Less is more: Vision representation compression for efficient video generation with large language models.
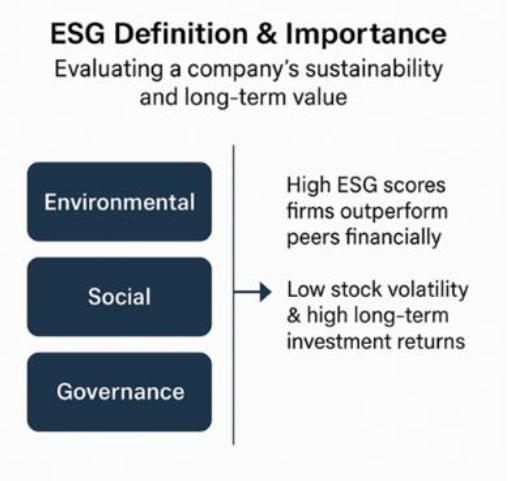
Downloads
Published
How to Cite
Issue
Section
ARK
License
Copyright (c) 2025 The author retains copyright and grants the journal the right of first publication.

This work is licensed under a Creative Commons Attribution 4.0 International License.