The Evolution of Distributed Denial of Service (DDoS) Attacks: NLP-Based Detection and Strategic Management Countermeasures in Modern Networks
DOI:
https://doi.org/10.5281/zenodo.13232838ARK:
https://n2t.net/ark:/40704/JETBM.v1n4a04References:
42Keywords:
Distributed Denial of Service (DDoS), Natural Language Processing (NLP), Cyber Attacks, Network Security, Threat Detection, incident Response, Business Continuity, Crisis Management, Botnets, Cybersecurity StrategyAbstract
Distributed Denial of Service (DDoS) attacks have evolved significantly over the years, becoming more sophisticated and challenging to mitigate. As organizations increasingly rely on modern networks for critical operations, the need for advanced detection and strategic management countermeasures has become paramount. This paper explores the evolution of DDoS attacks, focusing on the integration of Natural Language Processing (NLP) for enhanced detection and the implementation of strategic management practices to mitigate these threats. Through a comprehensive review of existing literature, case studies, and emerging technologies, the paper provides insights into the current state of DDoS defense mechanisms and proposes a framework for integrating NLP and management strategies to protect modern networks from these pervasive threats.
This study highlights how NLP techniques can analyze communication patterns within network traffic to identify early indicators of DDoS attacks, offering a proactive approach to threat detection. Additionally, the paper emphasizes the role of strategic management in ensuring a comprehensive response, from incident detection to business continuity planning. By examining the interplay between technical and managerial approaches, the paper seeks to provide a holistic solution to the growing challenge of DDoS attacks in increasingly complex network environments.
References
Bhuyan, M. H., Bhattacharyya, D. K., & Kalita, J. K. (2015). Network anomaly detection: Methods, systems, and tools. IEEE Communications Surveys & Tutorials, 16(1), 303-336. https://doi.org/10.1109/COMST.2014.2336610
Wang, H., Zhang, D., & Shin, K. G. (2007). Detecting SYN flooding attacks. IEEE INFOCOM 2002. Proceedings of the 21st Annual Joint Conference of the IEEE Computer and Communications Societies., 3, 1530-1539. https://doi.org/10.1109/INFCOM.2002.1019432
Sommer, R., & Paxson, V. (2010). Outside the closed world: On using machine learning for network intrusion detection. 2010 IEEE Symposium on Security and Privacy, 305-316. https://doi.org/10.1109/SP.2010.25
Zargar, S. T., Joshi, J., & Tipper, D. (2013). A survey of defense mechanisms against distributed denial of service (DDoS) flooding attacks. IEEE Communications Surveys & Tutorials, 15(4), 2046-2069. https://doi.org/10.1109/SURV.2013.031413.00127
Shameli-Sendi, A., Ahmad, I., & Kolahdouzan, M. R. (2015). Taxonomy of distributed denial of service mitigation approaches. Journal of Network and Computer Applications, 61, 174-197. https://doi.org/10.1016/j.jnca.2015.10.015
Feinstein, L., Schnackenberg, D., Balupari, R., & Kindred, D. (2003). Statistical approaches to DDoS attack detection and response. DARPA Information Survivability Conference and Exposition, 303-314. https://doi.org/10.1109/DISCEX.2003.1194899
Chhabra, S., & Sehgal, R. (2021). Enhancing DDoS detection using Natural Language Processing in network traffic data. International Journal of Network Security & Its Applications (IJNSA), 13(1), 15-28. https://doi.org/10.5121/ijnsa.2021.13102
Gkiotsalitis, K., & Cats, O. (2020). Public transport planning adaption under the COVID-19 pandemic crisis: Literature review of research needs and directions. Transport Reviews, 40(5), 606-622. https://doi.org/10.1080/01441647.2020.1814106
Garcia, S., Grill, M., Stiborek, J., & Zunino, A. (2014). An empirical comparison of botnet detection methods. Computers & Security, 45, 100-123. https://doi.org/10.1016/j.cose.2014.05.011
Chen, Q., Li, D., & Wang, L. (2024). Blockchain Technology for Enhancing Network Security. Journal of Industrial Engineering and Applied Science, 2(4), 22–28.
Chen, Q., Li, D., & Wang, L. (2024). The Role of Artificial Intelligence in Predicting and Preventing Cyber Attacks. Journal of Industrial Engineering and Applied Science, 2(4), 29–35.
Chen, Q., Li, D., & Wang, L. (2024). Network Security in the Internet of Things (IoT) Era. Journal of Industrial Engineering and Applied Science, 2(4), 36–41.
Li, D., Chen, Q., & Wang, L. (2024). Cloud Security: Challenges and Solutions. Journal of Industrial Engineering and Applied Science, 2(4), 42–47.
Li, D., Chen, Q., & Wang, L. (2024). Phishing Attacks: Detection and Prevention Techniques. Journal of Industrial Engineering and Applied Science, 2(4), 48–53.
Song, C., Zhao, G., & Wu, B. (2024). Applications of Low-Power Design in Semiconductor Chips. Journal of Industrial Engineering and Applied Science, 2(4), 54–59.
Zhao, G., Song, C., & Wu, B. (2024). 3D Integrated Circuit (3D IC) Technology and Its Applications. Journal of Industrial Engineering and Applied Science, 2(4), 60–65.
Wu, B., Song, C., & Zhao, G. (2024). Applications of Heterogeneous Integration Technology in Chip Design. Journal of Industrial Engineering and Applied Science, 2(4), 66–72.
Song, C., Wu, B., & Zhao, G. (2024). Optimization of Semiconductor Chip Design Using Artificial Intelligence. Journal of Industrial Engineering and Applied Science, 2(4), 73–80.
Song, C., Wu, B., & Zhao, G. (2024). Applications of Novel Semiconductor Materials in Chip Design. Journal of Industrial Engineering and Applied Science, 2(4), 81–89.
Stone-Gross, B., Holz, T., Stringhini, G., & Vigna, G. (2011). The underground economy of spam: A botmaster's perspective of coordinating large-scale spam campaigns. 6th USENIX Workshop on Large-Scale Exploits and Emergent Threats (LEET 13). https://www.usenix.org/conference/leet13/workshop-program/presentation/stone-gross
Liu, T., Cai, Q., Xu, C., Zhou, Z., Ni, F., Qiao, Y., & Yang, T. (2024). Rumor Detection with a novel graph neural network approach. arXiv Preprint arXiv:2403. 16206.
Liu, T., Cai, Q., Xu, C., Zhou, Z., Xiong, J., Qiao, Y., & Yang, T. (2024). Image Captioning in news report scenario. arXiv Preprint arXiv:2403. 16209.
Xu, C., Qiao, Y., Zhou, Z., Ni, F., & Xiong, J. (2024a). Accelerating Semi-Asynchronous Federated Learning. arXiv Preprint arXiv:2402. 10991.
Zhou, J., Liang, Z., Fang, Y., & Zhou, Z. (2024). Exploring Public Response to ChatGPT with Sentiment Analysis and Knowledge Mapping. IEEE Access.
Zhou, Z., Xu, C., Qiao, Y., Xiong, J., & Yu, J. (2024). Enhancing Equipment Health Prediction with Enhanced SMOTE-KNN. Journal of Industrial Engineering and Applied Science, 2(2), 13–20.
Zhou, Z., Xu, C., Qiao, Y., Ni, F., & Xiong, J. (2024). An Analysis of the Application of Machine Learning in Network Security. Journal of Industrial Engineering and Applied Science, 2(2), 5–12.
Zhou, Z. (2024). ADVANCES IN ARTIFICIAL INTELLIGENCE-DRIVEN COMPUTER VISION: COMPARISON AND ANALYSIS OF SEVERAL VISUALIZATION TOOLS.
Xu, C., Qiao, Y., Zhou, Z., Ni, F., & Xiong, J. (2024b). Enhancing Convergence in Federated Learning: A Contribution-Aware Asynchronous Approach. Computer Life, 12(1), 1–4.
Wang, L., Xiao, W., & Ye, S. (2019). Dynamic Multi-label Learning with Multiple New Labels. Image and Graphics: 10th International Conference, ICIG 2019, Beijing, China, August 23--25, 2019, Proceedings, Part III 10, 421–431. Springer.
Wang, L., Fang, W., & Du, Y. (2024). Load Balancing Strategies in Heterogeneous Environments. Journal of Computer Technology and Applied Mathematics, 1(2), 10–18.
Wang, L. (2024). Low-Latency, High-Throughput Load Balancing Algorithms. Journal of Computer Technology and Applied Mathematics, 1(2), 1–9.
Wang, L. (2024). Network Load Balancing Strategies and Their Implications for Business Continuity. Academic Journal of Sociology and Management, 2(4), 8–13.
Li, W. (2024). The Impact of Apple’s Digital Design on Its Success: An Analysis of Interaction and Interface Design. Academic Journal of Sociology and Management, 2(4), 14–19.
Wu, R., Zhang, T., & Xu, F. (2024). Cross-Market Arbitrage Strategies Based on Deep Learning. Academic Journal of Sociology and Management, 2(4), 20–26.
Wu, R. (2024). Leveraging Deep Learning Techniques in High-Frequency Trading: Computational Opportunities and Mathematical Challenges. Academic Journal of Sociology and Management, 2(4), 27–34.
Wang, L. (2024). The Impact of Network Load Balancing on Organizational Efficiency and Managerial Decision-Making in Digital Enterprises. Academic Journal of Sociology and Management, 2(4), 41–48.
Chen, Q., & Wang, L. (2024). Social Response and Management of Cybersecurity Incidents. Academic Journal of Sociology and Management, 2(4), 49–56.
Song, C. (2024). Optimizing Management Strategies for Enhanced Performance and Energy Efficiency in Modern Computing Systems. Academic Journal of Sociology and Management, 2(4), 57–64.
Zhou, Z., & Wu, R. (2024). Stock Price Prediction Model Based on Convolutional Neural Networks. Journal of Industrial Engineering and Applied Science, 2(4), 1–7.
Zhang, C., Zhou, Z., & Wu, R. (2024). Optimization of Automated Trading Systems with Deep Learning Strategies. Journal of Industrial Engineering and Applied Science, 2(4), 8–14.
Zhang, C., Zhou, Z., & Wu, R. (2024). Analyzing and Predicting Financial Time Series Data Using Recurrent Neural Networks. Journal of Industrial Engineering and Applied Science, 2(4), 15–21.
Zhang, C., Zhou, Z., & Wu, R. (2024). Analyzing and Predicting Financial Time Series Data Using Recurrent Neural Networks. Journal of Industrial Engineering and Applied Science, 2(4), 15–21.
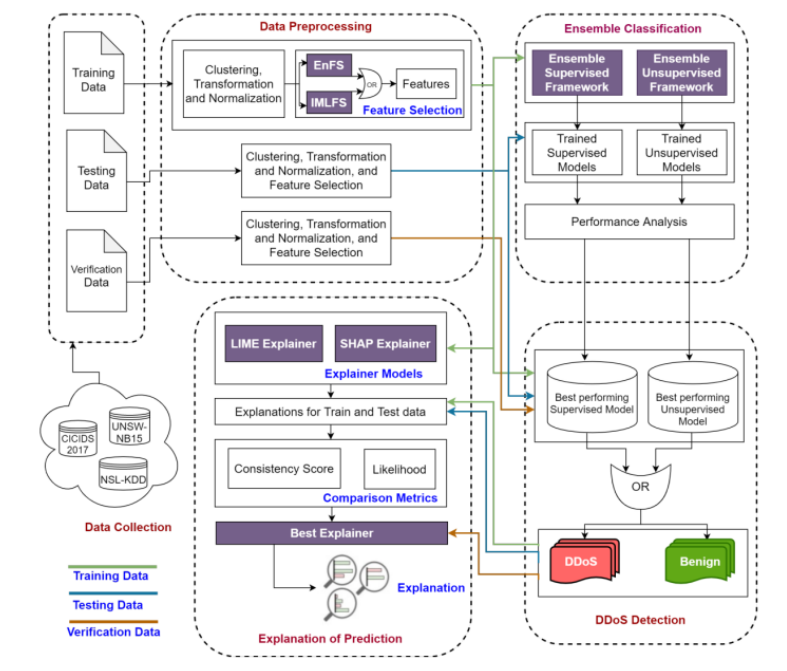
Downloads
Published
How to Cite
Issue
Section
ARK
License
Copyright (c) 2024 The author retains copyright and grants the journal the right of first publication.

This work is licensed under a Creative Commons Attribution 4.0 International License.