Machine Learning Based Prediction of Water Demand in Megacities: A Case Study of Beijing
DOI:
https://doi.org/10.5281/zenodo.10791242Keywords:
sustainability, nature-based solution, sustainable development goalsAbstract
With the acceleration of urbanization, smart cities are increasingly entering the public consciousness. Beijing, a city facing severe water scarcity, has seen some relief in its water supply pressure through the South-to-North Water Diversion Project. However, as economic development progresses and the population continues to expand, the demand for water in Beijing is still on the rise. Conducting a scientific and rational prediction of water demand is a prerequisite and foundation for planning and constructing future water supply projects. This paper embarks on a study of water demand prediction in Beijing, China, initially identifying 13 explanatory variables related to economics, society, water usage, and resources. Utilizing data from Beijing from 2004 to 2020, a predictive model encompassing both statistical and machine learning models for water demand was established. The findings indicate that among all the models considered, the Random Forest model performed the best, with R2 scores of 97.9% and 97.8%, respectively. A comparative analysis of the model's predictive performance further demonstrates the superiority of machine learning models over statistical models. The results of this study offer valuable insights for the planning and construction of future water supply projects in Beijing. They can serve as a reference for the formulation of water supply management policies in other cities.
Downloads
Metrics
References
Kim, J., Lee, H., Lee, M., Han, H., Kim, D., & Kim, H. S. (2022). Development of a Deep Learning-Based Prediction Model for Water Consumption at the Household Level. Water, 14(9), 1512.
Fu, H. (2023). A Comprehensive Review of Nature-Based Solutions: Current status and future research. AIMS Environmental Science, 10(5).
Sidhu, R. K., Kumar, R., & Rana, P. S. (2020). Machine learning based crop water demand forecasting using minimum climatological data. Multimedia Tools and Applications, 79, 13109-13124.
Zang, H., & Dong, X. (2024). Optimizing Soil Health Management in Smart Agriculture: Deep Learning Algorithms for Nutrient Analysis and Fertilizer Recommendation with Precision Agriculture Systems. Journal of Industrial Engineering and Applied Science, 2(1), 1-7.
Rezaali, M., Quilty, J., & Karimi, A. (2021). Probabilistic urban water demand forecasting using wavelet-based machine learning models. Journal of Hydrology, 600, 126358.
Ni, F., Zang, H., & Qiao, Y. (2024, January). Smartfix: Leveraging machine learning for proactive equipment maintenance in industry 4.0. In The 2nd International scientific and practical conference “Innovations in education: prospects and challenges of today”(January 16-19, 2024) Sofia, Bulgaria. International Science Group. 2024. 389 p. (p. 313).
Guo, G., Liu, S., Wu, Y., Li, J., Zhou, R., & Zhu, X. (2018). Short-term water demand forecast based on deep learning method. Journal of Water Resources Planning and Management, 144(12), 04018076.
Yan, X., Xiao, M., Wang, W., Li, Y., & Zhang, F. (2024). A Self-Guided Deep Learning Technique for MRI Image Noise Reduction. Journal of Theory and Practice of Engineering Science, 4(01), 109-117.
Ahmad, T., & Chen, H. (2018). Utility companies strategy for short-term energy demand forecasting using machine learning based models. Sustainable cities and society, 39, 401-417.
Chen, S., Li, K., Fu, H., Wu, Y. C., & Huang, Y. (2023). Sea Ice Extent Prediction with Machine Learning Methods and Subregional Analysis in the Arctic. Atmosphere, 14(6), 1023.
Weimin, W. A. N. G., Yufeng, L. I., Xu, Y. A. N., Mingxuan, X. I. A. O., & Min, G. A. O. (2024). Enhancing Liver Segmentation: A Deep Learning Approach with EAS Feature Extraction and Multi-Scale Fusion. International Journal of Innovative Research in Computer Science & Technology, 12(1), 26-34.
Nunes Carvalho, T. M., de Souza Filho, F. D. A., & Porto, V. C. (2021). Urban water demand modeling using machine learning techniques: Case study of Fortaleza, Brazil. Journal of Water Resources Planning and Management, 147(1), 05020026.
Hsieh, Y. T., Anjum, K., & Pompili, D. (2022, October). Ultra-low Power Analog Recurrent Neural Network Design Approximation for Wireless Health Monitoring. In 2022 IEEE 19th International Conference on Mobile Ad Hoc and Smart Systems (MASS) (pp. 211-219). IEEE.
Ibrahim, T., Omar, Y., & Maghraby, F. A. (2020, March). Water demand forecasting using machine learning and time series algorithms. In 2020 International Conference on Emerging Smart Computing and Informatics (ESCI) (pp. 325-329). IEEE.
Smolak, K., Kasieczka, B., Fialkiewicz, W., Rohm, W., Siła-Nowicka, K., & Kopańczyk, K. (2020). Applying human mobility and water consumption data for short-term water demand forecasting using classical and machine learning models. Urban Water Journal, 17(1), 32-42.
Wang, Q., & Wang, S. (2020). Machine learning-based water level prediction in Lake Erie. Water, 12(10), 2654.
Hsieh, Y. T., Qi, Z., & Pompili, D. (2022, November). ML-based Joint Doppler Estimation and Compensation in Underwater Acoustic Communications. In Proceedings of the 16th International Conference on Underwater Networks & Systems (pp. 1-8).
Xu, Z., Lv, Z., Li, J., & Shi, A. (2022). A novel approach for predicting water demand with complex patterns based on ensemble learning. Water Resources Management, 36(11), 4293-4312.
Tianqi, Y. (2022). Integrated models for rocking of offshore wind turbine structures. American Journal of Interdisciplinary Research in Engineering and Sciences, 9(1), 13-24.
Truong, V. H., Ly, Q. V., Le, V. C., Vu, T. B., Tran, T. T., & Goethals, P. (2021). Machine learning-based method for forecasting water levels in irrigation and drainage systems. Environmental Technology & Innovation, 23, 101762.
Potočnik, P., Škerl, P., & Govekar, E. (2021). Machine-learning-based multi-step heat demand forecasting in a district heating system. Energy and Buildings, 233, 110673.
Candelieri, A., Giordani, I., Archetti, F., Barkalov, K., Meyerov, I., Polovinkin, A., ... & Zolotykh, N. (2019). Tuning hyperparameters of a SVM-based water demand forecasting system through parallel global optimization. Computers & Operations Research, 106, 202-209.
Guo, Y., Wang, J., Chen, H., Li, G., Liu, J., Xu, C., ... & Huang, Y. (2018). Machine learning-based thermal response time ahead energy demand prediction for building heating systems. Applied energy, 221, 16-27.
Garzón, A., Kapelan, Z., Langeveld, J., & Taormina, R. (2022). Machine Learning‐Based Surrogate Modeling for Urban Water Networks: Review and Future Research Directions. Water Resources Research, 58(5), e2021WR031808.
Kavya, M., Mathew, A., Shekar, P. R., & Sarwesh, P. (2023). Short term water demand forecast modelling using artificial intelligence for smart water management. Sustainable Cities and Society, 95, 104610.
Deng, T., Chau, K. W., & Duan, H. F. (2021). Machine learning based marine water quality prediction for coastal hydro-environment management. Journal of Environmental Management, 284, 112051.
Hanoon, S. K., Abdullah, A. F., Shafri, H. Z., & Wayayok, A. (2022). A Novel Approach Based on Machine Learning and Public Engagement to Predict Water-Scarcity Risk in Urban Areas. ISPRS International Journal of Geo-Information, 11(12), 606.
Wang, L., & El-Gohary, N. M. (2019, June). Machine Learning-Based Prediction of Building Water Consumption for Improving Building Water Efficiency. In ASCE International Conference on Computing in Civil Engineering 2019 (pp. 139-145). Reston, VA: American Society of Civil Engineers.
Li, X., Wang, X., Chen, X., Lu, Y., Fu, H., & Wu, Y. C. (2024). Unlabeled data selection for active learning in image classification. Scientific Reports, 14(1), 424.
Wang, L., & El-Gohary, N. M. (2019, June). Machine Learning-Based Prediction of Building Water Consumption for Improving Building Water Efficiency. In ASCE International Conference on Computing in Civil Engineering 2019 (pp. 139-145). Reston, VA: American Society of Civil Engineers.
Nasaruddin, N., Zakaria, S. F., Ahmad, A., Ul-Saufie, A. Z., & Mohamaed Noor, N. (2021). Water demand prediction using machine learning: a review.
Candelieri, A., Soldi, D., & Archetti, F. (2015). Layered machine learning for short-term water demand forecasting. Environmental Engineering & Management Journal (EEMJ), 14(9).
Khan, P. W., Byun, Y. C., Lee, S. J., & Park, N. (2020). Machine learning based hybrid system for imputation and efficient energy demand forecasting. Energies, 13(11), 2681.
Kamoona, A., Song, H., Keshavarzian, K., Levy, K., Jalili, M., Wilkinson, R., ... & Meegahapola, L. (2023). Machine learning based energy demand prediction. Energy Reports, 9, 171-176.
Seo, Y., Kwon, S., & Choi, Y. (2018). Short-term water demand forecasting model combining variational mode decomposition and extreme learning machine. Hydrology, 5(4), 54.
Yi, S., Kondolf, G. M., Sandoval-Solis, S., & Dale, L. (2022). Application of machine learning-based energy use forecasting for inter-basin water transfer project. Water Resources Management, 36(14), 5675-5694.
Mokhtar, A., Al-Ansari, N., El-Ssawy, W., Graf, R., Aghelpour, P., He, H., ... & Abuarab, M. (2023). Prediction of Irrigation Water Requirements for Green Beans-Based Machine Learning Algorithm Models in Arid Region. Water resources management, 37(4), 1557-1580.
Liao, Z., Zang, N., Wang, X., Li, C., & Liu, Q. (2021). Machine learning-based prediction of chlorophyll-a variations in receiving reservoir of world’s largest water transfer project—a case study in the miyun reservoir, North China. Water, 13(17), 2406.
Ji, G., Wang, J., Ge, Y., & Liu, H. (2014, May). Urban water demand forecasting by LS-SVM with tuning based on elitist teaching-learning-based optimization. In The 26th Chinese Control and Decision Conference (2014 CCDC) (pp. 3997-4002). IEEE.
Ye, G., Wan, J., Deng, Z., Wang, Y., Zhu, B., Yan, Z., & Ji, S. (2024). Machine learning-based prediction of biological oxygen demand and unit electricity consumption in different-scale wastewater treatment plants. Journal of Environmental Chemical Engineering, 12(2), 111849.
Ye, G., Wan, J., Deng, Z., Wang, Y., Zhu, B., Yan, Z., & Ji, S. (2024). Machine learning-based prediction of biological oxygen demand and unit electricity consumption in different-scale wastewater treatment plants. Journal of Environmental Chemical Engineering, 12(2), 111849.
Liu, W., Yu, H., Yang, L., Yin, Z., Zhu, M., & Wen, X. (2021). Deep learning-based predictive framework for groundwater level forecast in arid irrigated areas. Water, 13(18), 2558.
Liu, W., Yu, H., Yang, L., Yin, Z., Zhu, M., & Wen, X. (2021). Deep learning-based predictive framework for groundwater level forecast in arid irrigated areas. Water, 13(18), 2558.
Houchati, M., Beitelmal, A. H., & Khraisheh, M. (2022). Predictive modeling for rooftop solar energy throughput: a machine learning-based optimization for building energy demand scheduling. Journal of Energy Resources Technology, 144(1), 011302.
Groppo, G. D. S., Costa, M. A., & Libânio, M. (2023). Predicting time-series for water demand in the big data environment using statistical methods, machine learning and the novel analog methodology dynamic time scan forecasting. Water Supply, 23(2), 624-644.
Murthy, A., Green, C., Stoleru, R., Bhunia, S., Swanson, C., & Chaspari, T. (2019, November). Machine learning-based irrigation control optimization. In Proceedings of the 6th ACM International Conference on Systems for Energy-Efficient Buildings, Cities, and Transportation (pp. 213-222).
Aggarwal, S., & Sehgal, S. (2021, August). Prediction of Water Consumption for New York city using Machine Learning. In 2021 8th International Conference on Signal Processing and Integrated Networks (SPIN) (pp. 486-490). IEEE.
Li, W., Finsa, M. M., Laskey, K. B., Houser, P., & Douglas-Bate, R. (2023). Groundwater level prediction with machine learning to support sustainable irrigation in water scarcity regions. Water, 15(19), 3473.
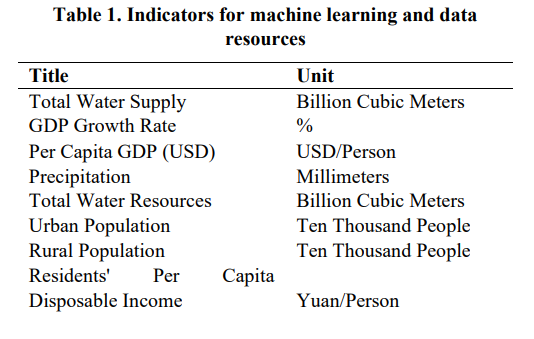
Downloads
Published
How to Cite
Issue
Section
License
Copyright (c) 2024 Journal of Industrial Engineering and Applied Science

This work is licensed under a Creative Commons Attribution 4.0 International License.