Predicting Enterprise Marketing Decision Making with Intelligent Data-Driven Approaches
DOI:
https://doi.org/10.5281/zenodo.11357252References:
30Keywords:
New Media Advertising, Audience Attention, Data-driven User Profiling, Marketing DecisionsAbstract
In order to improve the marketing effect of new media, traditional enterprises should increase the proportion of new media advertising and pay attention to the flow of audience's attention. On the basis of integrating data and information, advertising should conform to the changing trend of the media environment and increase the proportion of new media, so as to understand the audience's consumption habits of media use and formulate a reasonable media mix plan. Promote the integration of traditional media and new media to obtain higher publicity results at the lowest cost. This article delves into the key role of data-driven user profiling in marketing decisions. By analyzing user behavior, preferences, and needs, marketing teams are able to more accurately determine target markets, product positioning, and differentiation strategies. In addition, data-driven user profiles can guide the development of marketing and communication strategies, and enable enterprises to implement personalized marketing and customer relationship management. This personalized marketing and service experience helps to improve user loyalty and satisfaction, which in turn enhances the market competitiveness of enterprises. Overall, data-driven profiling plays an integral role in helping businesses achieve their continued growth and profitability goals.
Downloads
Metrics
References
Wang, Y., Zhu, M., Yuan, J., Wang, G., & Zhou, H. (2024). The intelligent prediction and assessment of financial information risk in the cloud computing model. arXiv preprint arXiv:2404.09322.
Lei, H., Wang, B., Shui, Z., Yang, P., & Liang, P. (2024). Automated Lane Change Behavior Prediction and Environmental Perception Based on SLAM Technology. arXiv preprint arXiv:2404.04492.
Cui, Z., Lin, L., Zong, Y., Chen, Y., & Wang, S. Precision Gene Editing Using Deep Learning: A Case Study of the CRISPR-Cas9 Editor.
Zheng, Haotian, et al. "Medication Recommendation System Based on Natural Language Processing for Patient Emotion Analysis." Academic Journal of Science and Technology 10.1 (2024): 62-68.
Li, Lianwei, et al. "Independent Grouped Information Expert Model: A Personalized Recommendation Algorithm Based on Deep Learning." (2024).
Xu, Kangming, et al. "Intelligent Classification and Personalized Recommendation of E-commerce Products Based on Machine Learning." arXiv preprint arXiv:2403.19345(2024).
Shen, Xinyu, et al. "Biology-based AI Predicts T-cell Receptor Antigen Binding Specificity." Academic Journal of Science and Technology 10.1 (2024): 23-27.
Ding, W., Zhou, H., Tan, H., Li, Z., & Fan, C. (2024). Automated Compatibility Testing Method for Distributed Software Systems in Cloud Computing.
Huang, C., Bandyopadhyay, A., Fan, W., Miller, A., & Gilbertson-White, S. (2023). Mental toll on working women during the COVID-19 pandemic: An exploratory study using Reddit data. PloS one, 18(1), e0280049.
Li, H., Wang, X., Feng, Y., Qi, Y., & Tian, J. (2024). Driving Intelligent IoT Monitoring and Control through Cloud Computing and Machine Learning. arXiv preprint arXiv:2403.18100.
Xu, J., Zhu, B., Jiang, W., Cheng, Q., & Zheng, H. (2024, April). AI-BASED RISK PREDICTION AND MONITORING IN FINANCIAL FUTURES AND SECURITIES MARKETS. In The 13th International scientific and practical conference “Information and innovative technologies in the development of society”(April 02–05, 2024) Athens, Greece. International Science Group. 2024. 321 p. (p. 222).
Wang, B., He, Y., Shui, Z., Xin, Q., & Lei, H. Predictive Optimization of DDoS Attack Mitigation in Distributed Systems using Machine Learning.
Li, X., Zong, Y., Yu, L., Li, L., & Wang, C. (2024, February). OPTIMIZING USER EXPERIENCE DESIGN AND PROJECT MANAGEMENT PRACTICES IN THE CONTEXT OF ARTIFICIAL INTELLIGENCE INNOVATION. In The 8th International scientific and practical conference “Priority areas of research in the scientific activity of teachers”(February 27–March 01, 2024) Zagreb, Croatia. International Science Group. 2024. 298 p. (p. 214).
Zhou, Hong, et al. "Application of Conversational Intelligent Reporting System Based on Artificial Intelligence and Large Language Models." Journal of Theory and Practice of Engineering Science 4.03 (2024): 176-182.
Zhou, Tong. "Improved sales forecasting using trend and seasonality decomposition with lightgbm." 2023 6th International Conference on Artificial Intelligence and Big Data (ICAIBD). IEEE, 2023.
Song, J., Li, X., Yu, L., Zong, Y., & Wang, C. INNOVATIVE RESEARCH ON INTERACTION DESIGN TALENT TRAINING IN THE ERA OF ARTIFICIAL INTELLIGENCE.
Zhu, Mengran, et al. "THE APPLICATION OF DEEP LEARNING IN FINANCIAL PAYMENT SECURITY AND THE CHALLENGE OF GENERATING ADVERSARIAL NETWORK MODELS." The 8th International scientific and practical conference “Priority areas of research in the scientific activity of teachers”(February 27–March 01, 2024) Zagreb, Croatia. International Science Group. 2024. 298 p.. 2024.
Li, X., Duan, S., Yu, L., Song, T., & Wang, C. (2024, March). REVISED SENTENCE DEVELOPING MACHINE LEARNING APPLICATIONS WITH A FOCUS ON USER EXPERIENCE, INSPIRED BY APPLE'S HUMAN. In The 12th International scientific and practical conference “Modern thoughts on the development of science: ideas, technologies and theories”(March 26–29, 2024) Amsterdam, Netherlands. International Science Group. 2024. 336 p. (p. 271).
Chen, P., Lam, K. H., Liu, Z., Mindlin, F. A., Chen, B., Gutierrez, C. B., ... & Jin, R. (2019). Structure of the full-length Clostridium difficile toxin B. Nature structural & molecular biology, 26(8), 712-719.
Tian, J., Li, H., Qi, Y., Wang, X., & Feng, Y. Intelligent Medical Detection and Diagnosis Assisted by Deep Learning.
Sun, Y. (2024). TransTARec: Time-Adaptive Translating Embedding Model for Next POI Recommendation. arXiv preprint arXiv:2404.07096.
He, Zheng, et al. "Application of K-means clustering based on artificial intelligence in gene statistics of biological information engineering."
Zhang, X., Wang, C., Chen, B., Wang, Q., Xu, W., Ye, S., ... & Zhang, R. (2019). Crystal structure of refolding fusion core of Lassa virus GP2 and design of Lassa virus fusion inhibitors. Frontiers in microbiology, 10, 1829.
Zhou, Y., Zhan, T., Wu, Y., Song, B., & Shi, C. RNA Secondary Structure Prediction Using Transformer-Based Deep Learning Models.
Sun, Y., Cui, Y., Hu, J., & Jia, W. (2018). Relation classification using coarse and fine-grained networks with SDP supervised key words selection. In Knowledge Science, Engineering and Management: 11th International Conference, KSEM 2018, Changchun, China, August 17–19, 2018, Proceedings, Part I 11 (pp. 514-522). Springer International Publishing.
Chen, B., Basak, S., Chen, P., Zhang, C., Perry, K., Tian, S., ... & Jin, R. (2022). Structure and conformational dynamics of Clostridioides difficile toxin A. Life science alliance, 5(6).
Wu, Y., Jin, Z., Shi, C., Liang, P., & Zhan, T. (2024). Research on the Application of Deep Learning-based BERT Model in Sentiment Analysis. arXiv preprint arXiv:2403.08217.
Shi, C., Liang, P., Wu, Y., Zhan, T., & Jin, Z. (2024). Maximizing User Experience with LLMOps-Driven Personalized Recommendation Systems. arXiv preprint arXiv:2404.00903.
Chen, B., Liu, Z., Perry, K., & Jin, R. (2022). Structure of the glucosyltransferase domain of TcdA in complex with RhoA provides insights into substrate recognition. Scientific reports, 12(1), 9028.
Chen, Baohua, Kay Perry, and Rongsheng Jin. "Neutralizing epitopes on Clostridioides difficile toxin A revealed by the structures of two camelid VHH antibodies." Frontiers in Immunology 13 (2022): 978858.
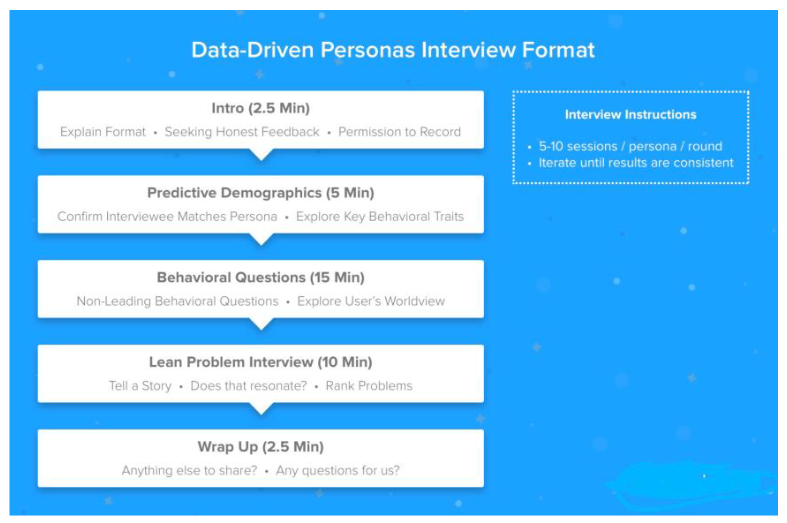
Downloads
Published
How to Cite
Issue
Section
License
Copyright (c) 2024 Journal of Industrial Engineering and Applied Science

This work is licensed under a Creative Commons Attribution 4.0 International License.