Research on The Prediction and Optimization of Blast Furnace Molten Iron Temperature Based on LSTM Long and Short-Term Neural Memory Network
DOI:
https://doi.org/10.5281/zenodo.13147689ARK:
https://n2t.net/ark:/40704/JIEAS.v2n4a21References:
12Keywords:
Iron and Steel industry, Green, OLS Regression Model, LSTM Long Short-term Memory Neural Network, PSO Particle Swarm Optimization Algorithm, Blast Furnace Molten Iron Temperature Prediction, Fuel RatioAbstract
The modern steel industry pursues green, low-consumption and high-quality development, and the prediction of fuel ratio and molten iron temperature is the key. However, the traditional prediction methods are limited, and the information age requires technological updates. In this paper, OLS regression, LSTM and PSO algorithms are used to establish and optimize the blast furnace molten iron temperature prediction model. First, analyze the literature and preprocess the data. Secondly, reduce dimension processing and select key variables. Then, construct the LSTM model to predict the temperature and evaluate the effect. Next, optimize the model with PSO and evaluate again. Finally, summarize the model, the prediction accuracy is high, especially the optimized model is significantly improve the prediction performance, but still need to be combined with the actual production of real-time detection and adjustment.
Downloads
Metrics
References
Bian, L. (2014). Water temperature intelligent online monitoring device in the application of ironmaking blast furnace. Electronic World, (16), 44.
Bi, C. (2022). Research on iron and steel interface molten iron temperature prediction based on data-driven model. Inner Mongolia University of Science and Technology, (03), 78.
Li, J. (2014). Modeling and forecasting of blast furnace molten iron temperature based on data mining. Inner Mongolia University of Science and Technology, (06), 73.
Zhang, Y., Li, J., & Cui, G. (2013). Modeling study of wavelet neural network in blast furnace molten iron temperature prediction. Computer and Applied Chemistry, 30(10), 1173-1176.
Cui, G., Li, J., Zhang, Y., Lu, J., & Ma, X. (2014). Multivariate time series modeling and prediction of blast furnace molten iron temperature. Journal of Iron and Steel Research, 26(04), 33-37.
Pan, D., Jiang, Z., Xu, C., & Gui, W. (2023). Research progress on the detection method of blast furnace molten iron temperature. Journal of Instrumentation, 44(12), 280-296.
Zhao, Y. (2015). Blast furnace molten iron temperature prediction based on data preprocessing and intelligent optimization. Inner Mongolia University of Science and Technology, (08), 66.
Guo, X. (2019). Analysis of the impact of oxygen enrichment on blast furnace ironmaking. Tianjin Metallurgy, (01), 18-20.
Rolling Steel House Self-media. (2024, June 1). Blast Furnace Ironmaking Process. Retrieved from https://zhuanlan.zhihu.com/p/677074317?utm_id=0
Zhang, W., & Bi, C. (2015). Discussion on the relationship between coke quality and blast furnace fuel index in Meigang. Proceedings of the Third Symposium on Ironmaking Benchmarking, Energy Saving and Cost Reduction and Related Technologies in 2015, 165-169.
Empty. (2024, June 5). Retrieved from https://blog.csdn.net/weixin_42746469/article/details/94394760
A team of Wang students. (2024, June 5). Retrieved from https://blog.csdn.net/Wang_Dou_Dou_/article/details/119417078
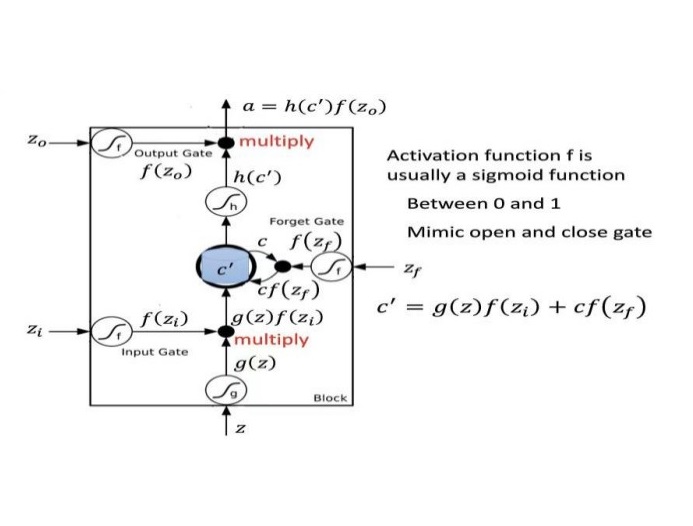
Downloads
Published
How to Cite
Issue
Section
ARK
License
Copyright (c) 2024 The author retains copyright and grants the journal the right of first publication.

This work is licensed under a Creative Commons Attribution 4.0 International License.