Application of Deep Reinforcement Learning for Cryptocurrency Market Trend Forecasting and Risk Management
DOI:
https://doi.org/10.5281/zenodo.13854906ARK:
https://n2t.net/ark:/40704/JIEAS.v2n5a08References:
18Keywords:
Deep Reinforcement Learning (DRL), Long Short-term Memory Network (LSTM), The Cryptocurrency Market, Risk Management Time Series ForecastingAbstract
With the gradual development and integration of artificial intelligence into various industries, there is also a great range of integration in the financial industry. Therefore, this article focuses on the trend prediction model and financial risk management problems of deep reinforcement learning (DRL), one of the largest branches of artificial intelligence, in the cryptocurrency market. In addition, in the experimental part of this paper, the artificial intelligence machine learning Long short-term memory network (LSTM) model is used to make effective time series prediction and analysis on the relevant data of the cryptocurrency market, so as to make a large-scale analysis to improve the accuracy of market trend prediction and the effectiveness of risk management. In addition, in this experiment, technology-related indicators, emotional states of financial market customers and other content related to large language models are combined. While optimizing investment strategy by using deep reinforcement learning algorithm, machine learning prediction model is also used to capture the time dependence of financial market. The experimental results also show that the predicted results are consistent with the actual value. Therefore, the model has high practical application value in predicting the time series price trend of cryptocurrency in the financial market and indicates that the integrated DRL model framework can further optimize and manage the price and trading strategy of the financial market. Future research should focus on improving the LSTM model and incorporating more features to improve prediction accuracy and adapt to market changes.
Downloads
Metrics
References
Jiang, Z., & Liang, J. (2017, September). Cryptocurrency portfolio management with deep reinforcement learning. In 2017 Intelligent systems conference (IntelliSys) (pp. 905-913). IEEE.
Lucarelli, G., & Borrotti, M. (2019). A deep reinforcement learning approach for automated cryptocurrency trading. In Artificial Intelligence Applications and Innovations: 15th IFIP WG 12.5 International Conference, AIAI 2019, Hersonissos, Crete, Greece, May 24–26, 2019, Proceedings 15 (pp. 247-258). Springer International Publishing.
Xu, Y., Liu, Y., Xu, H., & Tan, H. (2024). AI-Driven UX/UI Design: Empirical Research and Applications in FinTech. International Journal of Innovative Research in Computer Science & Technology, 12(4), 99-109.
Liu, Y., Xu, Y., & Song, R. (2024). Transforming User Experience (UX) through Artificial Intelligence (AI) in interactive media design. Engineering Science & Technology Journal, 5(7), 2273-2283.
Li, H., Wang, S. X., Shang, F., Niu, K., & Song, R. (2024). Applications of Large Language Models in Cloud Computing: An Empirical Study Using Real-world Data. International Journal of Innovative Research in Computer Science & Technology, 12(4), 59-69.
Ping, G., Wang, S. X., Zhao, F., Wang, Z., & Zhang, X. (2024). Blockchain Based Reverse Logistics Data Tracking: An Innovative Approach to Enhance E-Waste Recycling Efficiency.
Schnaubelt, M. (2022). Deep reinforcement learning for the optimal placement of cryptocurrency limit orders. European Journal of Operational Research, 296(3), 993-1006.
Lei, H., Wang, B., Shui, Z., Yang, P., & Liang, P. (2024). Automated Lane Change Behavior Prediction and Environmental Perception Based on SLAM Technology. arXiv preprint arXiv:2404.04492.
Wang, B., Zheng, H., Qian, K., Zhan, X., & Wang, J. (2024). Edge computing and AI-driven intelligent traffic monitoring and optimization. Applied and Computational Engineering, 77, 225-230.
Xu, H., Niu, K., Lu, T., & Li, S. (2024). Leveraging artificial intelligence for enhanced risk management in financial services: Current applications and future prospects. Engineering Science & Technology Journal, 5(8), 2402-2426.
Shi, Y., Shang, F., Xu, Z., & Zhou, S. (2024). Emotion-Driven Deep Learning Recommendation Systems: Mining Preferences from User Reviews and Predicting Scores. Journal of Artificial Intelligence and Development, 3(1), 40-46.
Wang, Shikai, Kangming Xu, and Zhipeng Ling. "Deep Learning-Based Chip Power Prediction and Optimization: An Intelligent EDA Approach." International Journal of Innovative Research in Computer Science & Technology 12.4 (2024): 77-87.
Ping, G., Zhu, M., Ling, Z., & Niu, K. (2024). Research on Optimizing Logistics Transportation Routes Using AI Large Models. Applied Science and Engineering Journal for Advanced Research, 3(4), 14-27.
Shang, F., Shi, J., Shi, Y., & Zhou, S. (2024). Enhancing E-Commerce Recommendation Systems with Deep Learning-based Sentiment Analysis of User Reviews. International Journal of Engineering and Management Research, 14(4), 19-34.
Kochliaridis, V., Kouloumpris, E., & Vlahavas, I. (2023). Combining deep reinforcement learning with technical analysis and trend monitoring on cryptocurrency markets. Neural Computing and Applications, 35(29), 21445-21462.
Betancourt, Carlos, and Wen-Hui Chen. "Reinforcement learning with self-attention networks for cryptocurrency trading." Applied Sciences 11.16 (2021): 7377.
Yang, M., Huang, D., Zhang, H., & Zheng, W. (2024). AI-Enabled Precision Medicine: Optimizing Treatment Strategies Through Genomic Data Analysis. Journal of Computer Technology and Applied Mathematics, 1(3), 73-84.
Wen, X., Shen, Q., Zheng, W., & Zhang, H. (2024). AI-Driven Solar Energy Generation and Smart Grid Integration A Holistic Approach to Enhancing Renewable Energy Efficiency. International Journal of Innovative Research in Engineering and Management, 11(4), 55-55.
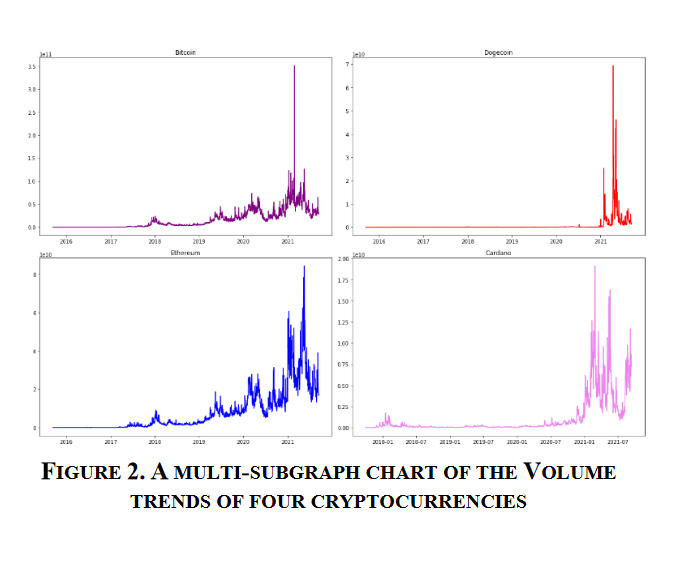
Downloads
Published
How to Cite
Issue
Section
ARK
License
Copyright (c) 2024 The author retains copyright and grants the journal the right of first publication.

This work is licensed under a Creative Commons Attribution 4.0 International License.