The Application of Generative AI in Virtual Reality and Augmented Reality
DOI:
https://doi.org/10.70393/6a69656173.323339ARK:
https://n2t.net/ark:/40704/JIEAS.v2n6a01Disciplines:
Computer ScienceSubjects:
Generative AIReferences:
47Keywords:
Virtual Reality (VR), Augmented Reality (AR), Immersion, InteractionAbstract
This study explores the historical development of Virtual Reality (VR) and Augmented Reality (AR) technologies and their applications in various fields, such as education, tourism, and consumer experiences. Through a review of relevant literature, the paper analyzes how VR and AR enhance user engagement and satisfaction by providing immersive and interactive experiences. In education, VR and AR are used to create vivid learning environments, promoting students' understanding and interest; in the tourism industry, these technologies enhance visitors' exploration and experience of destinations; in business, AR applications improve consumer shopping experiences and brand loyalty.
Despite the widespread application of these technologies facing challenges such as high costs and user adaptability issues, research shows their potential remains significant. Future research should focus on optimizing user experience, lowering technological barriers, and expanding application scenarios. In conclusion, the development of VR and AR technologies will drive innovation and transformation across industries, offering richer experiences in daily life.
Downloads
Metrics
References
Wang, C., Kang, D., Sun, H. Y., Qian, S. H., Wang, Z. X., Bao, L., & Zhang, S. H. (2024). MeGA: Hybrid Mesh-Gaussian Head Avatar for High-Fidelity Rendering and Head Editing. arXiv preprint arXiv:2404.19026.
Wang, D. (Ed.). (2016). Information Science and Electronic Engineering: Proceedings of the 3rd International Conference of Electronic Engineering and Information Science (ICEEIS 2016), January 4-5, 2016, Harbin, China. CRC Press.
Huang,S., Yang, H., Yao, Y., Lin, X.& Tu, Y. (2024). Deep adaptive interest network: personalized recommendation with context-aware learning. arXiv preprint arXiv:2409.02425.
Z. Wang et al., ―Cdc-yolofusion: Leveraging cross-scale dynamic convolution fusion for visible-infrared object detection,‖ IEEE Transactions on Intelligent Vehicles, pp. 1–14, 2024.
Yu, P., Xu, X., & Wang, J. (2024). Applications of Large Language Models in Multimodal Learning. Journal of Computer Technology and Applied Mathematics, 1(4), 108-116.
Yang, J., Liu, J., Yao, Z., & Ma, C. (2024). Measuring digitalization capabilities using machine learning. Research in International Business and Finance, 70, 102380. doi:10.1016/j.ribaf.2024.102380
Ma, B., Ma, B., Gao, M., Wang, Z., Ban, X., Huang, H., & Wu, W. (2021). Deep learning‐based automatic inpainting for material microscopic images. Journal of Microscopy, 281(3), 177-189.
Zhang, H., Guo, J., Li, K., Zhang, Y., & Zhao, Y. (2024). Offline Signature Verification Based on Feature Disentangling Aided Variational Autoencoder. arXiv preprint arXiv:2409.19754.
Li, S., Lin, J., Shi, H., Zhang, J., Wang, S., Yao, Y., ... & Yang, K. (2024). DTCLMapper: Dual Temporal Consistent Learning for Vectorized HD Map Construction. arXiv preprint arXiv:2405.05518.
Cao, J., Xu, R., Lin, X., Qin, F., Peng, Y., & Shao, Y. (2023). Adaptive receptive field U-shaped temporal convolutional network for vulgar action segmentation. Neural Computing and Applications, 35(13), 9593–9606. https://doi.org/10.1007/s00500-022-05938-1
Shijie Liu, Kang Yan, Feiwei Qin, Changmiao Wang, Ruiquan Ge, Kai Zhang, Jie Huang, Yong Peng, and Jin Cao. Infrared image super-resolution via lightweight information split network. In International Conference on Intelligent Computing, pages 293–304. Springer, 2024.
Jiang, H., Qin, F., Cao, J., Peng, Y., & Shao, Y. (2021). Recurrent neural network from adder’s perspective: Carry-lookahead RNN. Neural Networks, 144, 297–306. https://doi.org/10.1016/j.neunet.2021.07.011
Yang, Y., Jin, Y., Tian, Q., Yang, Y., Qin, W., & Ke, X. (2024). Enhancing Gastrointestinal Diagnostics with YOLO-Based Deep Learning Techniques. Preprints. https://doi.org/10.20944/preprints202408.1202.v1
Y. Jin, “Graphcnnpred: A stock market indices prediction using a graph based deep learning system,” arXiv preprint arXiv:2407.03760, 2024.
Yu, P., Cui, V. Y., & Guan, J. (2021, March). Text classification by using natural language processing. In Journal of Physics: Conference Series (Vol. 1802, No. 4, p. 042010). IOP Publishing.
Feuerriegel, S., Hartmann, J., Janiesch, C., & Zschech, P. (2024). Generative ai. Business & Information Systems Engineering, 66(1), 111-126.
Brynjolfsson, E., Li, D., & Raymond, L. R. (2023). Generative AI at work (No. w31161). National Bureau of Economic Research.
Epstein, Z., Hertzmann, A., Investigators of Human Creativity, Akten, M., Farid, H., Fjeld, J., ... & Smith, A. (2023). Art and the science of generative AI. Science, 380(6650), 1110-1111.
Bretos, M. A., Ibáñez-Sánchez, S., & Orús, C. (2024). Applying virtual reality and augmented reality to the tourism experience: a comparative literature review. Spanish Journal of Marketing-ESIC, 28(3), 287-309.
Raji, M. A., Olodo, H. B., Oke, T. T., Addy, W. A., Ofodile, O. C., & Oyewole, A. T. (2024). Business strategies in virtual reality: a review of market opportunities and consumer experience. International Journal of Management & Entrepreneurship Research, 6(3), 722-736.
Mergen, M., Graf, N., & Meyerheim, M. (2024). Reviewing the current state of virtual reality integration in medical education-a scoping review. BMC Medical Education, 24(1), 788.
Lampropoulos, G., & Kinshuk. (2024). Virtual reality and gamification in education: a systematic review. Educational technology research and development, 1-95.
Berkman, M. I. (2024). History of virtual reality. In Encyclopedia of computer graphics and games (pp. 873-881). Cham: Springer International Publishing.
Omran, W., Ramos, R. F., & Casais, B. (2024). Virtual reality and augmented reality applications and their effect on tourist engagement: a hybrid review. Journal of Hospitality and Tourism Technology, 15(4), 497-518.
Hidayat, R., & Wardat, Y. (2024). A systematic review of augmented reality in science, technology, engineering and mathematics education. Education and Information Technologies, 29(8), 9257-9282.
Koumpouros, Y. (2024). Revealing the true potential and prospects of augmented reality in education. Smart Learning Environments, 11(1), 2.
Wahid, A., Huda, M., Rohim, M. A., Ali, A. H., Kaspin, K. G., Fiqiyah, M., & Jima’ain, M. T. A. (2024, February). Augmented reality model in supporting instruction process: a critical review. In International Congress on Information and Communication Technology (pp. 69-83). Singapore: Springer Nature Singapore.
Schultz, C. D., & Kumar, H. (2024). ARvolution: Decoding consumer motivation and value dimensions in augmented reality. Journal of Retailing and Consumer Services, 78, 103701.
Rauschnabel, P. A., Felix, R., Heller, J., & Hinsch, C. (2024). The 4C framework: Towards a holistic understanding of consumer engagement with augmented reality. Computers in Human Behavior, 154, 108105.
Wahyuanto, E., Heriyanto, H., & Hastuti, S. (2024). Study of the Use of Augmented Reality Technology in Improving the Learning Experience in the Classroom. West Science Social and Humanities Studies, 2(05), 700-705.
Tao Y. SQBA: sequential query-based blackbox attack, Fifth International Conference on Artificial Intelligence and Computer Science (AICS 2023). SPIE, 2023, 12803: 721-729.
Tao Y. Meta Learning Enabled Adversarial Defense, 2023 IEEE International Conference on Sensors, Electronics and Computer Engineering (ICSECE). IEEE, 2023: 1326-1330.
Yiyi Tao, Yiling Jia, Nan Wang, and Hongning Wang. 2019. The FacT: Taming Latent Factor Models for Explainability with Factorization Trees. In Proceedings of the 42nd International ACM SIGIR Conference on Research and Development in Information Retrieval (SIGIR'19). Association for Computing Machinery, New York, NY, USA, 295–304.
Yiyi Tao, Zhuoyue Wang, Hang Zhang, Lun Wang. 2024. NEVLP: Noise-Robust Framework for Efficient Vision-Language Pre-training. arXiv:2409.09582.
Luo, M., Zhang, W., Song, T., Li, K., Zhu, H., Du, B., & Wen, H. (2021, January). Rebalancing expanding EV sharing systems with deep reinforcement learning. In Proceedings of the Twenty-Ninth International Conference on International Joint Conferences on Artificial Intelligence (pp. 1338-1344).
Luo, M., Du, B., Zhang, W., Song, T., Li, K., Zhu, H., ... & Wen, H. (2023). Fleet rebalancing for expanding shared e-Mobility systems: A multi-agent deep reinforcement learning approach. IEEE Transactions on Intelligent Transportation Systems, 24(4), 3868-3881.
Zhu, H., Luo, Y., Liu, Q., Fan, H., Song, T., Yu, C. W., & Du, B. (2019). Multistep flow prediction on car-sharing systems: A multi-graph convolutional neural network with attention mechanism. International Journal of Software Engineering and Knowledge Engineering, 29(11n12), 1727–1740.
Sun, Y., & Ortiz, J. (2024). Machine Learning-Driven Pedestrian Recognition and Behavior Prediction for Enhancing Public Safety in Smart Cities. Journal of Artificial Intelligence and Information, 1, 51-57.
Yaonian Zhong, Enhancing the Heat Dissipation Efficiency of Computing Units Within Autonomous Driving Systems and Electric Vehicles, J. World Journal of Innovation and Modern Technology, 2024, 7 (5): 100-104.
Lin, W. (2024). A Review of Multimodal Interaction Technologies in Virtual Meetings. Journal of Computer Technology and Applied Mathematics, 1(4), 60-68.
Lin, W. (2024). A Systematic Review of Computer Vision-Based Virtual Conference Assistants and Gesture Recognition. Journal of Computer Technology and Applied Mathematics, 1(4), 28-35.
Luo, M., Zhang, W., Song, T., Li, K., Zhu, H., Du, B., & Wen, H. (2021, January). Rebalancing expanding EV sharing systems with deep reinforcement learning. In Proceedings of the Twenty-Ninth International Conference on International Joint Conferences on Artificial Intelligence (pp. 1338-1344).
Luo, M., Du, B., Zhang, W., Song, T., Li, K., Zhu, H., ... & Wen, H. (2023). Fleet rebalancing for expanding shared e-Mobility systems: A multi-agent deep reinforcement learning approach. IEEE Transactions on Intelligent Transportation Systems, 24(4), 3868-3881.
Zhu, H., Luo, Y., Liu, Q., Fan, H., Song, T., Yu, C. W., & Du, B. (2019). Multistep flow prediction on car-sharing systems: A multi-graph convolutional neural network with attention mechanism. International Journal of Software Engineering and Knowledge Engineering, 29(11n12), 1727–1740.
Sun, Y., & Ortiz, J. (2024). Machine Learning-Driven Pedestrian Recognition and Behavior Prediction for Enhancing Public Safety in Smart Cities. Journal of Artificial Intelligence and Information, 1, 51-57.
Yaonian Zhong, Enhancing the Heat Dissipation Efficiency of Computing Units Within Autonomous Driving Systems and Electric Vehicles, J. World Journal of Innovation and Modern Technology, 2024, 7 (5): 100-104.
Leong, H. Y., Gao, Y. F., Shuai, J., Zhang, Y., & Pamuksuz, U. (2024). Efficient Fine-Tuning of Large Language Models for Automated Medical Documentation. arXiv preprint arXiv:2409.09324.
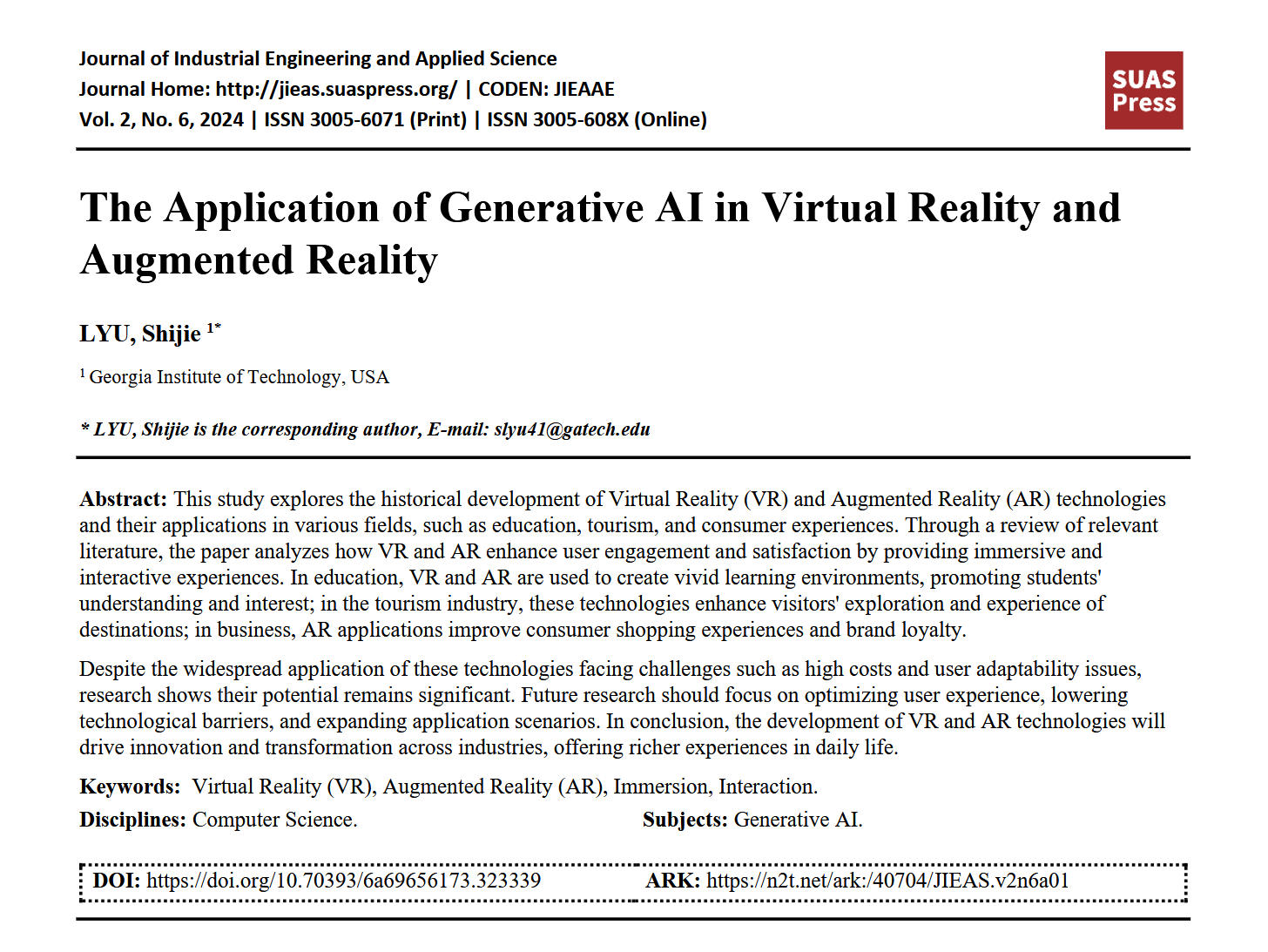
Downloads
Published
How to Cite
Issue
Section
ARK
License
Copyright (c) 2024 The author retains copyright and grants the journal the right of first publication.

This work is licensed under a Creative Commons Attribution 4.0 International License.