FSMM: Food System Monitoring Model for Re-optimization
DOI:
https://doi.org/10.70393/6a69656173.323433ARK:
https://n2t.net/ark:/40704/JIEAS.v2n6a11Disciplines:
Applied MathematicsSubjects:
Mathematical ModelingReferences:
28Keywords:
Boosting Regression, Linear Regression, FSMM, Entropy Weight, Data Mining, Supervised Learning, Energy UseAbstract
The unstability of global food system have aroused growing attention worldwide. Althrough there is sufficient food produced to feed every person, many people in the world are suffering from hunger. Moreover, the current food system is harmful to the environment. As our global population continues to rise, the ability to produce more food while sustaining, and even improving, the health of our environment counts.
The FSMM(Food System Monitoring Model) provides score rank using entropy weight and subjective weight to evaluate the current state of the food system for most countries in the world. It contains 9 individual features to measure multiple aspects, including efficiency, profitability, sustainability and equity.
Considering the future trend of the food system, we apply Gray Forecast Model to forecast the next 5 years of the development of the food system to figure out the time for each country to change into equity and sustainability.
To find out the relation between external factors and food system, The FSMM uses Boosting Regression Model to find the correlation between the scores and 10 indicators which represent the influences of energy use, government, agriculture, social factors and population ratio. The FSMM fits the data from 178 countries from 2000 to 2019. After simulating and adjusting the parameters to decrease the loss of the model, we gets the importance rate of each indicator.
We find the indicators whose importance rate change significantly when the food system is optimized for equity and sustainability. And we use these indicatos as dependent variable and apply Linear Regression to fit by using the yields of the wheat, meat and vegetables as independent variable. By doing this, We find out the direct impact of re-optimization on the food system.
We finally get the importance rate and the coefficient of two regression model and analyze the specific influence of various factors on the food system and compare the differences between the influence on developing and developed countries.
After the overall analysis of the influence on developed and developing countries, we pick some specific developing and developed countries to analyze and discuss the scalability and adaptability of the model.
Downloads
Metrics
References
Han Cao et al. “Mitigating Knowledge Conflicts in Language Model-Driven Question Answer- ing”. In: arXiv preprint arXiv:2411.11344 (2024).
Han-Cheng Dan, Bingjie Lu, and Mengyu Li. “Evaluation of asphalt pavement texture using multiview stereo reconstruction based on deep learning”. In: Construction and Building Materials 412 (2024), p. 134837.
Han-Cheng Dan et al. “Image-driven prediction system: Automatic extraction of aggregate gradation of pavement core samples integrating deep learning and interactive image processing framework”. In: Construction and Building Materials 453 (2024), p. 139056.
Han-Cheng Dan et al. “Multiple distresses detection for Asphalt Pavement using improved you Only Look Once Algorithm based on convolutional neural network”. In: International Journal of Pavement Engineering 25.1 (2024), p. 2308169.
Polly J Ericksen. “Conceptualizing food systems for global environmental change research”. In: Global environmental change 18.1 (2008), pp. 234–245.
Xiu Fang et al. “A Domain-Aware Crowdsourcing System with Copier Removal”. In: Interna- tional Conference on Internet of Things, Communication and Intelligent Technology. Springer. 2022, pp. 761–773.
Xiu Fang et al. “Selecting workers wisely for crowdsourcing when copiers and domain experts co-exist”. In: Future Internet 14.2 (2022), p. 37.
T Garnett and C Godfray. Navigating a course through competing food system priorities. Food Climate Research Network and the Oxford Martin Programme on the Future of Food, University of Oxford, UK. 2012.
Yuting Hu et al. “Improving text-image matching with adversarial learning and circle loss for multi-modal steganography”. In: International Workshop on Digital Watermarking. Springer. 2020, pp. 41–52.
Zhuohuan Hu et al. “Research on Financial Multi-Asset Portfolio Risk Prediction Model Based on Convolutional Neural Networks and Image Processing”. In: Applied Science and Engineering Journal for Advanced Research 3.6 (Nov. 2024), pp. 39–50. DOI: 10.5281/ zenodo.14214385. URL: https://asejar.singhpublication.com/index.php/ojs/ article/view/115.
Ashtad Javanmardi et al. “Enhancing construction project workflow reliability through observe– plan–do–check–react cycle: A bridge project case study”. In: Buildings 13.9 (2023), p. 2379. 2
Muxin Jia, Ang Liu, and Taro Narahara. “The Integration of Dual Evaluation and Minimum Spanning Tree Clustering to Support Decision-Making in Territorial Spatial Planning”. In: Sustainability 16.10 (2024). ISSN: 2071-1050. DOI: 10.3390/su16103928. URL: https://www.mdpi.com/2071-1050/16/10/3928.
Xiangtian Li et al. “Artistic Neural Style Transfer Algorithms with Activation Smoothing”. In: arXiv preprint arXiv:2411.08014 (2024).
Zhuo Li et al. “Recursive Balanced k-Subset Sum Partition for Rule-constrained Resource Allocation”. In: Proceedings of the 29th ACM International Conference on Information & Knowledge Management. 2020, pp. 2121–2124.
Dong Liu and Meng Jiang. “Distance Recomputator and Topology Reconstructor for Graph Neural Networks”. In: (2024). arXiv: 2406.17281 [cs.LG]. URL: https://arxiv.org/ abs/2406.17281.
Dong Liu, Meng Jiang, and Kaiser Pister. “LLMEasyQuant – An Easy to Use Toolkit for LLM Quantization”. In: (2024). arXiv: 2406.19657 [cs.LG]. URL: https://arxiv.org/abs/ 2406.19657.
Dong Liu et al. “GraphSnapShot: Graph Machine Learning Acceleration with Fast Storage and Retrieval”. In: (2024). arXiv: 2406.17918 [cs.LG]. URL: https://arxiv.org/abs/ 2406.17918.
Fang Liu et al. “Application of an ANN and LSTM-based Ensemble Model for Stock Market Prediction”. In: arXiv preprint arXiv:2410.20253 (2024).
Menglin Liu and Ang Liu. “Homeownership and Social Policy Preferences in China Mediating Roles of Employment Sector and Socioeconomic Perceptions”. In: Available at SSRN 4860606 ().
Enpu Ma et al. “Spatio-temporal evolution of global food security pattern and its influencing factors in 2000-2014”. In: Acta Geographica Sinica 75.2 (2020), pp. 332–347. DOI: 10.11821/dlxb202002009. URL: https://doi.org/10.11821/dlxb202002009.
Priyadarshi R Shukla et al. Climate Change and Land: an IPCC special report on climate change, desertification, land degradation, sustainable land management, food security, and greenhouse gas fluxes in terrestrial ecosystems. 2019.
Bingyang Wang, Ying Chen, and Zichao Li. “A novel Bayesian Pay-As-You-Drive insurance model with risk prediction and causal mapping”. In: Decision Analytics Journal (2024), p. 100522. ISSN: 2772-6622. DOI: https : / / doi . org / 10 . 1016 / j . dajour . 2024 . 100522.
Jia Wang et al. “IRDA: Incremental reinforcement learning for dynamic resource allocation”. In: IEEE Transactions on Big Data 8.3 (2020), pp. 770–783.
Yijie Weng and Jianhao Wu. “Fortifying the global data fortress: a multidimensional ex- amination of cyber security indexes and data protection measures across 193 nations”. In: International Journal of Frontiers in Engineering Technology 6.2 (2024). ISSN: 2706-655X. DOI: 10.25236/ijfet.2024.060206.
Yijie Weng and Jianhao Wu. “Leveraging Artificial Intelligence to Enhance Data Security and Combat Cyber Attacks”. In: Journal of Artificial Intelligence General science (JAIGS) ISSN: 3006-4023 5.1 (2024), pp. 392–399. DOI: 10.60087/jaigs.v5i1.211.
Yijie Weng et al. “Comprehensive Overview of Artificial Intelligence Applications in Modern Industries”. In: arXiv preprint arXiv:2409.13059 (2024). DOI: 10 . 48550 / arXiv . 2409 . 13059.
Ka Ho Wong et al. “BigARM: A big-data-driven airport resource management engine and application tools”. In: Database Systems for Advanced Applications: 25th International Con- ference, DASFAA 2020, Jeju, South Korea, September 24–27, 2020, Proceedings, Part III 25. Springer. 2020, pp. 741–744.
Wenjun Wu. “Alphanetv4: Alpha Mining Model”. In: arXiv preprint arXiv:2411.04409 (2024).
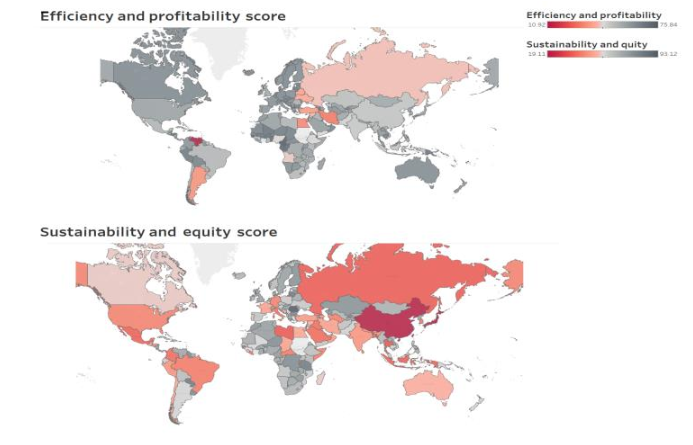
Downloads
Published
How to Cite
Issue
Section
ARK
License
Copyright (c) 2024 The author retains copyright and grants the journal the right of first publication.

This work is licensed under a Creative Commons Attribution 4.0 International License.