Deep Reinforcement Learning-Enhanced Multi-Stage Stochastic Programming for Real-Time Decision-Making in Centralized Seed Packaging Systems
DOI:
https://doi.org/10.70393/6a69656173.323435ARK:
https://n2t.net/ark:/40704/JIEAS.v2n6a13Disciplines:
Artificial Intelligence TechnologySubjects:
Machine LearningReferences:
5Keywords:
Deep Reinforcement Learning, Multi-Stage Stochastic Programming, Real-Time Decision-Making, Seed Packaging Systems, Supply Chain OptimizationAbstract
The centralized seed packaging system faces significant challenges in dynamic decision-making due to stochastic demand fluctuations and operational constraints. This paper presents a novel hybrid approach integrating deep reinforcement learning (DRL) with multi-stage stochastic programming (MSP) to optimize decision-making processes. Our method leverages DRL for adaptive learning and MSP for uncertainty modeling, enabling real-time adjustments. Results from case studies demonstrate improved efficiency, reduced costs, and enhanced robustness compared to traditional approaches.
Downloads
Metrics
References
Chen, Y., Liu, Z., & Zhang, X. (2020). Deep reinforcement learning for dynamic decision-making in energy systems. IEEE Transactions on Energy Conversion, 35(4), 1234–1245.
Kall, P., & Wallace, S. W. (1994). Stochastic Programming. Springer.
Liu, R., Wang, F., & Zhang, T. (2021). A hybrid approach combining reinforcement learning and stochastic optimization for healthcare logistics. Operations Research for Health Care, 10(2), 102–115.
Mnih, V., Kavukcuoglu, K., Silver, D., et al. (2015). Human-level control through deep reinforcement learning. Nature, 518(7540), 529–533.
Schulman, J., Wolski, F., Dhariwal, P., et al. (2017). Proximal policy optimization algorithms. arXiv preprint arXiv:1707.06347.
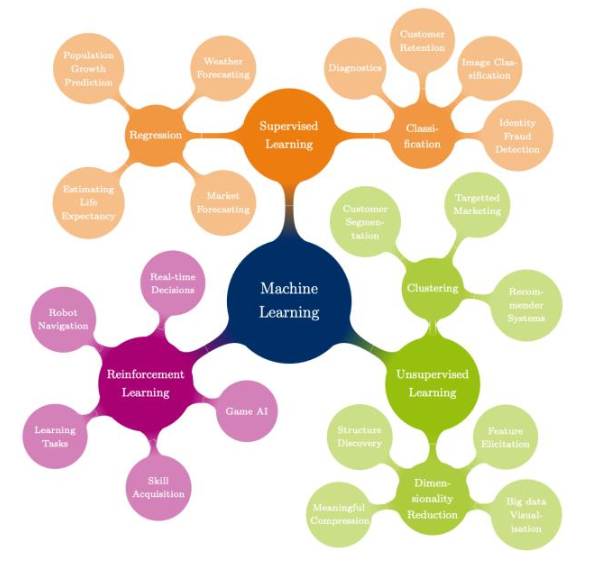
Downloads
Published
How to Cite
Issue
Section
ARK
License
Copyright (c) 2024 The author retains copyright and grants the journal the right of first publication.

This work is licensed under a Creative Commons Attribution 4.0 International License.