Combining Blockchain and AI to Optimize the Intelligent Risk Control Mechanism in Decentralized Finance
DOI:
https://doi.org/10.70393/6a69656173.323739ARK:
https://n2t.net/ark:/40704/JIEAS.v3n2a04Disciplines:
Artificial Intelligence TechnologySubjects:
CybersecurityReferences:
11Keywords:
Blockchain, Artificial Intelligence, Decentralized Finance (DeFi), Risk Management, Intelligent Risk Control, Smart ContractsAbstract
This study explores the optimized application of combining blockchain (Blockchain) and artificial intelligence (AI) in the intelligent risk control of decentralized finance (DeFi). Although the decentralization and transparency of DeFi have driven financial innovation, they have also introduced risks related to market manipulation, smart contract vulnerabilities, and liquidity. Traditional centralized risk control approaches struggle to adapt. This research proposes a blockchain+AI-based intelligent risk control framework. Blockchain’s tamper-resistance enhances transaction security, while AI’s intelligent learning capabilities improve risk identification. Experimental results show that this model outperforms traditional solutions in detection accuracy (94.1%), false alarm rate (2.1%), and detection latency (180ms), and it remains robust under high market volatility. The findings suggest that combining blockchain and AI can effectively strengthen DeFi risk control, enhance system transparency and security, and provide theoretical and practical directions for future intelligent and automated risk management.
Downloads
Metrics
References
[1] Yang, X., Wang, X., Li, Q., Zhou, H., & Wang, C. (2023). A decentralized cloud data integrity auditing scheme based on blockchain and fog computing. Journal of Electronics & Information Technology, 45(10), 3759–3766.
[2] Chen, X. (2021). Research on decentralized cloud storage technology based on blockchain (Master's thesis). Nanjing University of Posts and Telecommunications.
[3] Brenner, G., Baza, M., Rasheed, A., Lalouani, W., Badr, M., & Alshahrani, H. (2023). DPark: Decentralized smart private-parking system using blockchains. Journal of Grid Computing, 21(3).
[4] Li, H. (2021). A decentralized trading model based on blockchain dual identity structure and its applications (Doctoral dissertation). National University of Defense Technology.
[5] Chen, Z., Zhang, L., Zhang, S., & Kan, H. (2021). Access control identity scheme based on blockchain and decentralized attribute cryptography. Scientia Sinica Informationis, 51(8), 1345–1359.
[6] Mao, Y., Tao, D., Zhang, S., Qi, T., & Li, K. (2025). Research and Design on Intelligent Recognition of Unordered Targets for Robots Based on Reinforcement Learning. arXiv preprint arXiv:2503.07340.
[7] Sun, J., Zhang, S., Lian, J., Fu, L., Zhou, Z., Fan, Y., & Xu, K. (2024, December). Research on Deep Learning of Convolutional Neural Network for Action Recognition of Intelligent Terminals in the Big Data Environment and its Intelligent Software Application. In 2024 IEEE 7th International Conference on Automation, Electronics and Electrical Engineering (AUTEEE) (pp. 996-1004). IEEE.
[8] Yu, D., Liu, L., Wu, S., Li, K., Wang, C., Xie, J., ... & Ji, R. (2024). Machine learning optimizes the efficiency of picking and packing in automated warehouse robot systems. In 2024 International Conference on Computer Engineering, Network and Digital Communication (CENDC 2024).
[9] Li, K., Liu, L., Chen, J., Yu, D., Zhou, X., Li, M., ... & Li, Z. (2024, November). Research on reinforcement learning based warehouse robot navigation algorithm in complex warehouse layout. In 2024 6th International Conference on Artificial Intelligence and Computer Applications (ICAICA) (pp. 296-301). IEEE.
[10] Li, K., Wang, J., Wu, X., Peng, X., Chang, R., Deng, X., ... & Hong, B. (2024). Optimizing automated picking systems in warehouse robots using machine learning. arXiv preprint arXiv:2408.16633.
[11] Li, K., Chen, J., Yu, D., Dajun, T., Qiu, X., Lian, J., ... & Han, J. (2024, October). Deep reinforcement learning-based obstacle avoidance for robot movement in warehouse environments. In 2024 IEEE 6th International Conference on Civil Aviation Safety and Information Technology (ICCASIT) (pp. 342-348). IEEE.
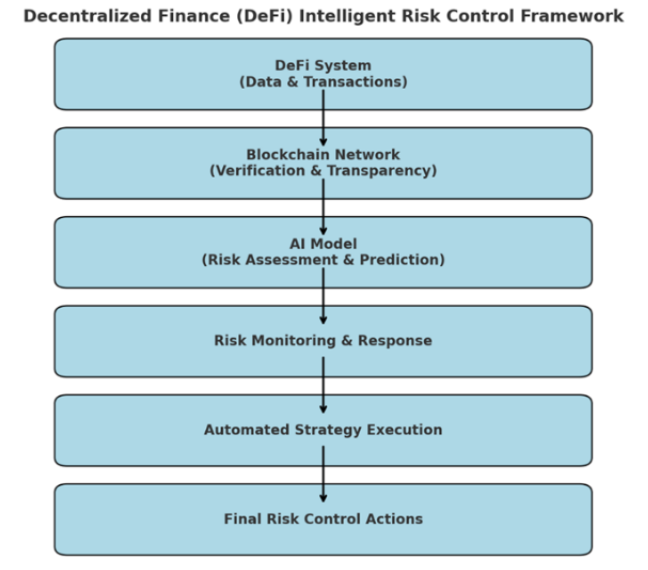
Downloads
Published
How to Cite
Issue
Section
ARK
License
Copyright (c) 2025 The author retains copyright and grants the journal the right of first publication.

This work is licensed under a Creative Commons Attribution 4.0 International License.