Phishing Attacks: Detection and Prevention Techniques
DOI:
https://doi.org/10.5281/zenodo.12789572ARK:
https://n2t.net/ark:/40704/JIEAS.v2n4a08References:
33Keywords:
Phishing Attacks, Detection Techniques, Prevention Strategies, Machine Learning, Heuristic-Based Detection, User Education, Email Phishing, Spear Phishing, Whaling, Smishing, Vishing, Cybersecurity, Multi-Factor Authentication, Email Authentication Protocols, Data Quality, Evasion Techniques, User ComplianceAbstract
Phishing attacks are among the most prevalent and damaging cyber threats faced by individuals and organizations today. These attacks deceive users into revealing sensitive information, leading to significant financial and reputational damage. This paper explores various techniques for detecting and preventing phishing attacks, examining their effectiveness and implementation challenges. Through comprehensive experiments and analysis, we demonstrate the efficacy of different detection methods and propose best practices for mitigating phishing threats. Our study includes a detailed evaluation of machine learning algorithms, heuristic-based approaches, and user education programs, supported by experimental data and real-world case studies.
Our research shows that while machine learning algorithms offer high detection accuracy, they require significant computational resources and continuous updates to remain effective against evolving phishing techniques. Heuristic-based approaches, on the other hand, provide quick detection with lower resource demands but may struggle with new or sophisticated attacks. User education programs are essential for long-term phishing prevention, as they empower users to recognize and avoid phishing attempts, significantly reducing the risk of successful attacks. By combining these methods, organizations can develop a robust defense strategy against phishing threats.
Downloads
Metrics
References
Abdelhamid, N., Ayesh, A., & Thabtah, F. (2014). Phishing detection: A recent intelligent machine learning comparison based on models content and features. IEEE, 8(7), 1-12.
Barracuda Networks. (2019). Spear Phishing: Top Threats and Trends.
Cybersecurity Insiders. (2019). Insider Threat Report 2019.
Das, A., Dingman, A., & Camp, L. J. (2018). Why Johnny Doesn’t Use Two Factor: A Two-Phase Usability Study of the FIDO U2F Security Key. Proceedings of the 2018 ACM International Joint Conference on Pervasive and Ubiquitous Computing.
Garera, S., Provos, N., Chew, M., & Rubin, A. D. (2007). A framework for detection and measurement of phishing attacks. WORM'07: Proceedings of the 5th ACM workshop on Recurring malcode, 1-8.
IBM. (2020). Cost of a Data Breach Report 2020.
Liu, T., Cai, Q., Xu, C., Zhou, Z., Ni, F., Qiao, Y., & Yang, T. (2024). Rumor Detection with a novel graph neural network approach. arXiv Preprint arXiv:2403. 16206.
Liu, T., Cai, Q., Xu, C., Zhou, Z., Xiong, J., Qiao, Y., & Yang, T. (2024). Image Captioning in news report scenario. arXiv Preprint arXiv:2403. 16209.
Xu, C., Qiao, Y., Zhou, Z., Ni, F., & Xiong, J. (2024a). Accelerating Semi-Asynchronous Federated Learning. arXiv Preprint arXiv:2402. 10991.
Zhou, J., Liang, Z., Fang, Y., & Zhou, Z. (2024). Exploring Public Response to ChatGPT with Sentiment Analysis and Knowledge Mapping. IEEE Access.
Zhou, Z., Xu, C., Qiao, Y., Xiong, J., & Yu, J. (2024). Enhancing Equipment Health Prediction with Enhanced SMOTE-KNN. Journal of Industrial Engineering and Applied Science, 2(2), 13–20.
Zhou, Z., Xu, C., Qiao, Y., Ni, F., & Xiong, J. (2024). An Analysis of the Application of Machine Learning in Network Security. Journal of Industrial Engineering and Applied Science, 2(2), 5–12.
Zhou, Z. (2024). ADVANCES IN ARTIFICIAL INTELLIGENCE-DRIVEN COMPUTER VISION: COMPARISON AND ANALYSIS OF SEVERAL VISUALIZATION TOOLS.
Xu, C., Qiao, Y., Zhou, Z., Ni, F., & Xiong, J. (2024b). Enhancing Convergence in Federated Learning: A Contribution-Aware Asynchronous Approach. Computer Life, 12(1), 1–4.
Wang, L., Xiao, W., & Ye, S. (2019). Dynamic Multi-label Learning with Multiple New Labels. Image and Graphics: 10th International Conference, ICIG 2019, Beijing, China, August 23--25, 2019, Proceedings, Part III 10, 421–431. Springer.
Wang, L., Fang, W., & Du, Y. (2024). Load Balancing Strategies in Heterogeneous Environments. Journal of Computer Technology and Applied Mathematics, 1(2), 10–18.
Wang, L. (2024). Low-Latency, High-Throughput Load Balancing Algorithms. Journal of Computer Technology and Applied Mathematics, 1(2), 1–9.
Wang, L. (2024). Network Load Balancing Strategies and Their Implications for Business Continuity. Academic Journal of Sociology and Management, 2(4), 8–13.
Li, W. (2024). The Impact of Apple’s Digital Design on Its Success: An Analysis of Interaction and Interface Design. Academic Journal of Sociology and Management, 2(4), 14–19.
Wu, R., Zhang, T., & Xu, F. (2024). Cross-Market Arbitrage Strategies Based on Deep Learning. Academic Journal of Sociology and Management, 2(4), 20–26.
Wu, R. (2024). Leveraging Deep Learning Techniques in High-Frequency Trading: Computational Opportunities and Mathematical Challenges. Academic Journal of Sociology and Management, 2(4), 27–34.
Wang, L. (2024). The Impact of Network Load Balancing on Organizational Efficiency and Managerial Decision-Making in Digital Enterprises. Academic Journal of Sociology and Management, 2(4), 41–48.
Chen, Q., & Wang, L. (2024). Social Response and Management of Cybersecurity Incidents. Academic Journal of Sociology and Management, 2(4), 49–56.
Song, C. (2024). Optimizing Management Strategies for Enhanced Performance and Energy Efficiency in Modern Computing Systems. Academic Journal of Sociology and Management, 2(4), 57–64.
Jansson, K., & Von Solms, R. (2013). Phishing for phishing awareness. Behaviour & Information Technology, 32(6), 584-593.
Kitterman, S. (2019). The evolution of DMARC: The email authentication standard. Messaging, Malware and Mobile Anti-Abuse Working Group (M3AAWG).
Proofpoint. (2020). The Human Factor 2020 Report.
Sahingoz, O. K., Buber, E., Demir, O., & Diri, B. (2019). Machine learning based phishing detection from URLs. Expert Systems with Applications, 117, 345-357.
Symantec. (2019). Internet Security Threat Report 2019.
Srinoy, S. (2017). Phishing website detection using URL and HTML features. International Journal of Network Security, 19(5), 760-770.
Verizon. (2020). Data Breach Investigations Report 2020.
Vinayakumar, R., Soman, K. P., & Poornachandran, P. (2019). Applying deep learning approaches for network traffic classification and intrusion detection. Springer, 62(1), 221-245.
Zhang, Y., Hong, J. I., & Cranor, L. F. (2007). Cantina: A content-based approach to detecting phishing web sites. Proceedings of the 16th international conference on World Wide Web, 639-648.
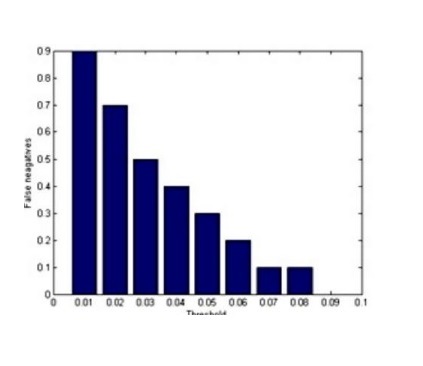
Downloads
Published
How to Cite
Issue
Section
ARK
License
Copyright (c) 2024 The author retains copyright and grants the journal the right of first publication.

This work is licensed under a Creative Commons Attribution 4.0 International License.