Applications of Low-Power Design in Semiconductor Chips
DOI:
https://doi.org/10.5281/zenodo.12794397ARK:
https://n2t.net/ark:/40704/JIEAS.v2n4a09References:
23Keywords:
Low-Power Design, Semiconductor Chips, Dynamic Voltage and Frequency Scaling (DVFS), Multi-Threshold CMOS (MTCMOS), Power Gating, Energy Efficiency, Power Consumption, Battery Life, Thermal Management, High-Performance Computing, Leakage Power, Sustainable Electronics, Advanced CMOS Technology, Performance Optimization, Low-Power TechniquesAbstract
As technology continues to evolve, the demand for high-performance yet low-power semiconductor chips has intensified. This paper explores the applications of low-power design in semiconductor chips, examining various methodologies, techniques, and their effectiveness. Through comprehensive analysis and experimental data, we highlight the significance of low-power design in modern electronics, its impact on performance, and future trends. The paper covers multiple low-power design strategies, including dynamic voltage and frequency scaling (DVFS), multi-threshold CMOS (MTCMOS), and power gating, supported by case studies and experimental results.
Our findings demonstrate that DVFS significantly reduces power consumption by dynamically adjusting voltage and frequency based on workload requirements, thus maintaining performance during low-demand periods. MTCMOS utilizes transistors with different threshold voltages to balance power and performance, effectively reducing leakage power in non-critical paths. Power gating, which involves switching off power to inactive parts of a chip, proved highly effective in reducing static power consumption. These techniques, when combined, offer a comprehensive approach to low-power semiconductor design, ensuring energy efficiency without compromising performance.
Downloads
Metrics
References
Borkar, S., & Chien, A. A. (2011). The future of microprocessors. Communications of the ACM, 54(5), 67-77.
Kaul, H., Anders, M., Hsu, S., Agarwal, A., Krishnamurthy, R., & Borkar, S. (2012). Near-threshold voltage (NTV) design: Opportunities and challenges. In Proceedings of the 49th Annual Design Automation Conference (pp. 1149-1154).
Kim, W., Kim, D., & Min, H. (2008). Adaptive voltage scaling in dual voltage designs. IEEE Transactions on Computer-Aided Design of Integrated Circuits and Systems, 27(8), 1530-1543.
Pedram, M. (1996). Power minimization in IC design: principles and applications. ACM Transactions on Design Automation of Electronic Systems (TODAES), 1(1), 3-56.
Takayanagi, T., Kuroda, T., & Sakurai, T. (1998). A 60-mW MPEG4 video codec using clustered voltage scaling with variable supply-voltage scheme. IEEE Journal of Solid-State Circuits, 33(11), 1772-1780.
Liu, T., Cai, Q., Xu, C., Zhou, Z., Ni, F., Qiao, Y., & Yang, T. (2024). Rumor Detection with a novel graph neural network approach. arXiv Preprint arXiv:2403. 16206.
Liu, T., Cai, Q., Xu, C., Zhou, Z., Xiong, J., Qiao, Y., & Yang, T. (2024). Image Captioning in news report scenario. arXiv Preprint arXiv:2403. 16209.
Xu, C., Qiao, Y., Zhou, Z., Ni, F., & Xiong, J. (2024a). Accelerating Semi-Asynchronous Federated Learning. arXiv Preprint arXiv:2402. 10991.
Zhou, J., Liang, Z., Fang, Y., & Zhou, Z. (2024). Exploring Public Response to ChatGPT with Sentiment Analysis and Knowledge Mapping. IEEE Access.
Zhou, Z., Xu, C., Qiao, Y., Xiong, J., & Yu, J. (2024). Enhancing Equipment Health Prediction with Enhanced SMOTE-KNN. Journal of Industrial Engineering and Applied Science, 2(2), 13–20.
Zhou, Z., Xu, C., Qiao, Y., Ni, F., & Xiong, J. (2024). An Analysis of the Application of Machine Learning in Network Security. Journal of Industrial Engineering and Applied Science, 2(2), 5–12.
Zhou, Z. (2024). ADVANCES IN ARTIFICIAL INTELLIGENCE-DRIVEN COMPUTER VISION: COMPARISON AND ANALYSIS OF SEVERAL VISUALIZATION TOOLS.
Xu, C., Qiao, Y., Zhou, Z., Ni, F., & Xiong, J. (2024b). Enhancing Convergence in Federated Learning: A Contribution-Aware Asynchronous Approach. Computer Life, 12(1), 1–4.
Wang, L., Xiao, W., & Ye, S. (2019). Dynamic Multi-label Learning with Multiple New Labels. Image and Graphics: 10th International Conference, ICIG 2019, Beijing, China, August 23--25, 2019, Proceedings, Part III 10, 421–431. Springer.
Wang, L., Fang, W., & Du, Y. (2024). Load Balancing Strategies in Heterogeneous Environments. Journal of Computer Technology and Applied Mathematics, 1(2), 10–18.
Wang, L. (2024). Low-Latency, High-Throughput Load Balancing Algorithms. Journal of Computer Technology and Applied Mathematics, 1(2), 1–9.
Wang, L. (2024). Network Load Balancing Strategies and Their Implications for Business Continuity. Academic Journal of Sociology and Management, 2(4), 8–13.
Li, W. (2024). The Impact of Apple’s Digital Design on Its Success: An Analysis of Interaction and Interface Design. Academic Journal of Sociology and Management, 2(4), 14–19.
Wu, R., Zhang, T., & Xu, F. (2024). Cross-Market Arbitrage Strategies Based on Deep Learning. Academic Journal of Sociology and Management, 2(4), 20–26.
Wu, R. (2024). Leveraging Deep Learning Techniques in High-Frequency Trading: Computational Opportunities and Mathematical Challenges. Academic Journal of Sociology and Management, 2(4), 27–34.
Wang, L. (2024). The Impact of Network Load Balancing on Organizational Efficiency and Managerial Decision-Making in Digital Enterprises. Academic Journal of Sociology and Management, 2(4), 41–48.
Chen, Q., & Wang, L. (2024). Social Response and Management of Cybersecurity Incidents. Academic Journal of Sociology and Management, 2(4), 49–56.
Song, C. (2024). Optimizing Management Strategies for Enhanced Performance and Energy Efficiency in Modern Computing Systems. Academic Journal of Sociology and Management, 2(4), 57–64.
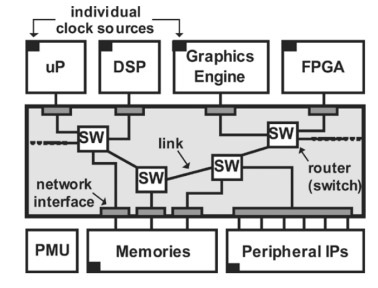
Downloads
Published
How to Cite
Issue
Section
ARK
License
Copyright (c) 2024 The author retains copyright and grants the journal the right of first publication.

This work is licensed under a Creative Commons Attribution 4.0 International License.