Leveraging AI in Traffic Engineering to Enhance Bicycle Mobility in Urban Areas
DOI:
https://doi.org/10.70393/6a69656173.323039ARK:
https://n2t.net/ark:/40704/JIEAS.v2n6a02Disciplines:
Artificial IntelligenceSubjects:
Traffic EngineeringReferences:
12Keywords:
AI-Driven Traffic Engineering, Urban Bicycle Mobility, Intelligent Transport SystemsAbstract
More With the rise of AI-driven technologies, urban cycling is becoming more accessible and appealing to many due to benefits such as health improvement and cost efficiency. Governments worldwide are promoting cycling as a sustainable transportation option to address environmental challenges. Ensuring seamless bicycle mobility in cities is essential to incentivize cycling. AI-powered Traffic Engineering can significantly enhance the flow of bicycle traffic in urban areas by optimizing infrastructure and safety. This article explores the benefits of cycling and the rationale for investing in AI-integrated cycling infrastructure. It provides examples of smart solutions such as AI-based vehicle-cycle segregation (including London's Cycle Superhighways), protected intersections enhanced by machine learning algorithms, and Intelligent Transport Systems (ITS) that incorporate AI for dynamic traffic management. Their implementation and impact on cyclists and overall traffic flow are analyzed, demonstrating that these advanced systems reduce accidents, boost road efficiency, and make cycling more enjoyable. Quantitative data on these improvements is also presented. In conclusion, AI-enabled Traffic Engineering solutions play a vital role in enhancing bicycle mobility and safety in urban environments.
Downloads
Metrics
References
Che, C., Hu, H., Zhao, X., Li, S., & Lin, Q. (2023). Advancing Cancer Document Classification with R andom Forest. Academic Journal of Science and Technology, 8(1), 278-280.
Huang, Z., Zheng, H., Li, C., & Che, C. (2024). Application of machine learning-based k-means clustering for financial fraud detection. Academic Journal of Science and Technology, 10(1), 33-39.
Che, C., Zheng, H., Huang, Z., Jiang, W., & Liu, B. (2024). Intelligent robotic control system based on computer vision technology. Applied and Computational Engineering, 64, 142-147.
Yu, L., Li, C., Gao, L., Liu, B., & Che, C. (2024, March). Stochastic analysis of touch-tone frequency recognition in two-way radio systems for dialed telephone number identification. In 2024 7th International Conference on Advanced Algorithms and Control Engineering (ICAACE) (pp. 1565-1572). IEEE.
Ghadban, M., Baayoun, A., Lakkis, I., Najem, S., Saliba, N., & Shihadeh, A. (2020). A novel method to improve temperature forecast in data-scarce urban environments with application to the Urban Heat Island in Beirut. Urban Climate, 33, 100648. https://doi.org/10.1016/j.uclim.2020.100648
Mateo, F., Carrasco, J., Sellami, A., Millán-Giraldo, M., Domínguez, M., & Soria-Olivas, E. (2013). Machine learning methods to forecast temperature in buildings. Expert Systems with Applications, 40(4), 1061-1068.
Che, C., Li, C., & Huang, Z. (2024). The Integration of Generative Artificial Intelligence and Computer Vision in Industrial Robotic Arms. International Journal of Computer Science and Information Technology, 2(3), 1-9.
Lin, Q., Che, C., Hu, H., Zhao, X., & Li, S. (2023). A Comprehensive Study on Early Alzheimer’s Disease Detection through Advanced Machine Learning Techniques on MRI Data. Academic Journal of Science and Technology, 8(1), 281-285.
Che, C., Huang, Z., Li, C., Zheng, H., & Tian, X. (2024). Integrating generative AI into financial market prediction for improved decision making. Applied and Computational Engineering, 64, 155-161.
Liu, H., Wang, C., Zhan, X., Zheng, H., & Che, C. (2024). Enhancing 3D Object Detection by Using Neural Network with Self-adaptive Thresholding. arXiv preprint arXiv:2405.07479.
Huang, Z., Che, C., Zheng, H., & Li, C. (2024). Research on Generative Artificial Intelligence for Virtual Financial Robo-Advisor. Academic Journal of Science and Technology, 10(1), 74-80.
Che, C., Lin, Q., Zhao, X., Huang, J., & Yu, L. (2023, September). Enhancing Multimodal Understanding with CLIP-Based Image-to-Text Transformation. In Proceedings of the 2023 6th International Conference on Big Data Technologies (pp. 414-418).
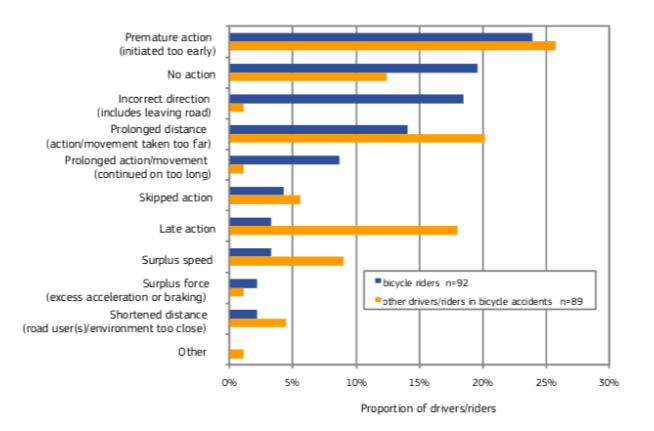
Downloads
Published
How to Cite
Issue
Section
ARK
License
Copyright (c) 2024 The author retains copyright and grants the journal the right of first publication.

This work is licensed under a Creative Commons Attribution 4.0 International License.