Interpretable Machine Learning: Explainability in Algorithm Design
DOI:
https://doi.org/10.70393/6a69656173.323337ARK:
https://n2t.net/ark:/40704/JIEAS.v2n6a07Disciplines:
Computer ScienceSubjects:
Machine LearningReferences:
22Keywords:
Machine Learning, AutoML, Computational EfficiencyAbstract
In recent years, there is a high demand for transparency and accountability in machine learning models, especially in domains such as healthcare, finance and etc. In this paper, we delve into deep how to make machine learning models more interpretable, with focus on the importance of the explainability of the algorithm design. The main objective of this paper is to fill this gap and provide a comprehensive survey and analytical study towards AutoML. To that end, we first introduce the AutoML technology and review its various tools and techniques.
Downloads
Metrics
References
Huang, Z., Zheng, H., Li, C., & Che, C. (2024). Application of machine learning-based k-means clustering for financial fraud detection. Academic Journal of Science and Technology, 10(1), 33-39.
Huang, Z., Che, C., Zheng, H., & Li, C. (2024). Research on Generative Artificial Intelligence for Virtual Financial Robo-Advisor. Academic Journal of Science and Technology, 10(1), 74-80.
Waring, J., Lindvall, C., & Umeton, R. (2020). Automated machine learning: Review of the state-of-the-art and opportunities for healthcare. Artificial intelligence in medicine, 104, 101822.
Che, C., Lin, Q., Zhao, X., Huang, J., & Yu, L. (2023, September). Enhancing Multimodal Understanding with CLIP-Based Image-to-Text Transformation. In Proceedings of the 2023 6th International Conference on Big Data Technologies (pp. 414-418).
Cheng, X. (2024). Machine Learning-Driven Fraud Detection: Management, Compliance, and Integration. Academic Journal of Sociology and Management, 2(6), 8-13.
Hutter, F., Kotthoff, L., & Vanschoren, J. (2019). Automated machine learning: methods, systems, challenges (p. 219). Springer Nature.
Lin, Q., Che, C., Hu, H., Zhao, X., & Li, S. (2023). A Comprehensive Study on Early Alzheimer’s Disease Detection through Advanced Machine Learning Techniques on MRI Data. Academic Journal of Science and Technology, 8(1), 281-285.
Vaccaro, L., Sansonetti, G., & Micarelli, A. (2021). An empirical review of automated machine learning. Computers, 10(1), 11.
Che, C., Huang, Z., Li, C., Zheng, H., & Tian, X. (2024). Integrating generative AI into financial market prediction for improved decision making. Applied and Computational Engineering, 64, 155-161.
Che, C., Li, C., & Huang, Z. (2024). The Integration of Generative Artificial Intelligence and Computer Vision in Industrial Robotic Arms. International Journal of Computer Science and Information Technology, 2(3), 1-9.
Che, C., & Tian, J. (2024). Game Theory: Concepts, Applications, and Insights from Operations Research. Journal of Computer Technology and Applied Mathematics, 1(4), 53-59.
Feurer, M., Klein, A., Eggensperger, K., Springenberg, J., Blum, M., & Hutter, F. (2015). Efficient and robust automated machine learning. Advances in neural information processing systems, 28.
Che, C., & Tian, J. (2024). Analyzing patterns in Airbnb listing prices and their classification in London through geospatial distribution analysis. Advances in Engineering Innovation, 12, 53-59.
Che, C., & Tian, J. (2024). Maximum flow and minimum cost flow theory to solve the evacuation planning. Advances in Engineering Innovation, 12, 60-64.
Che, C., & Tian, J. (2024). Understanding the Interrelation Between Temperature and Meteorological Factors: A Case Study of Szeged Using Machine Learning Techniques. Journal of Computer Technology and Applied Mathematics, 1(4), 47-52.
Cheng, X., Liu, K., Hu, X., Liu, T., Che, C., & Zhu, C. (2024). Comparative Analysis of Machine Learning Models for Music Recommendation. Theoretical and Natural Science, 53, 249-254.
Che, C., & Tian, J. (2024). Methods comparison for neural network-based structural damage recognition and classification. Advances in Operation Research and Production Management, 3, 20-26.
Cheng, X., & Che, C. (2024). Optimizing Urban Road Networks for Resilience Using Genetic Algorithms. Academic Journal of Sociology and Management, 2(6), 1-7.
Cheng, X. (2024). Investigations into the Evolution of Generative AI. Journal of Computer Technology and Applied Mathematics, 1(4), 117-122.
Tuggener, L., Amirian, M., Rombach, K., Lörwald, S., Varlet, A., Westermann, C., & Stadelmann, T. (2019, June). Automated machine learning in practice: state of the art and recent results. In 2019 6th Swiss Conference on Data Science (SDS) (pp. 31-36). IEEE.
Che, C., Hu, H., Zhao, X., Li, S., & Lin, Q. (2023). Advancing Cancer Document Classification with R andom Forest. Academic Journal of Science and Technology, 8(1), 278-280.
Chauhan, K., Jani, S., Thakkar, D., Dave, R., Bhatia, J., Tanwar, S., & Obaidat, M. S. (2020, March). Automated machine learning: The new wave of machine learning. In 2020 2nd International Conference on Innovative Mechanisms for Industry Applications (ICIMIA) (pp. 205-212). IEEE.
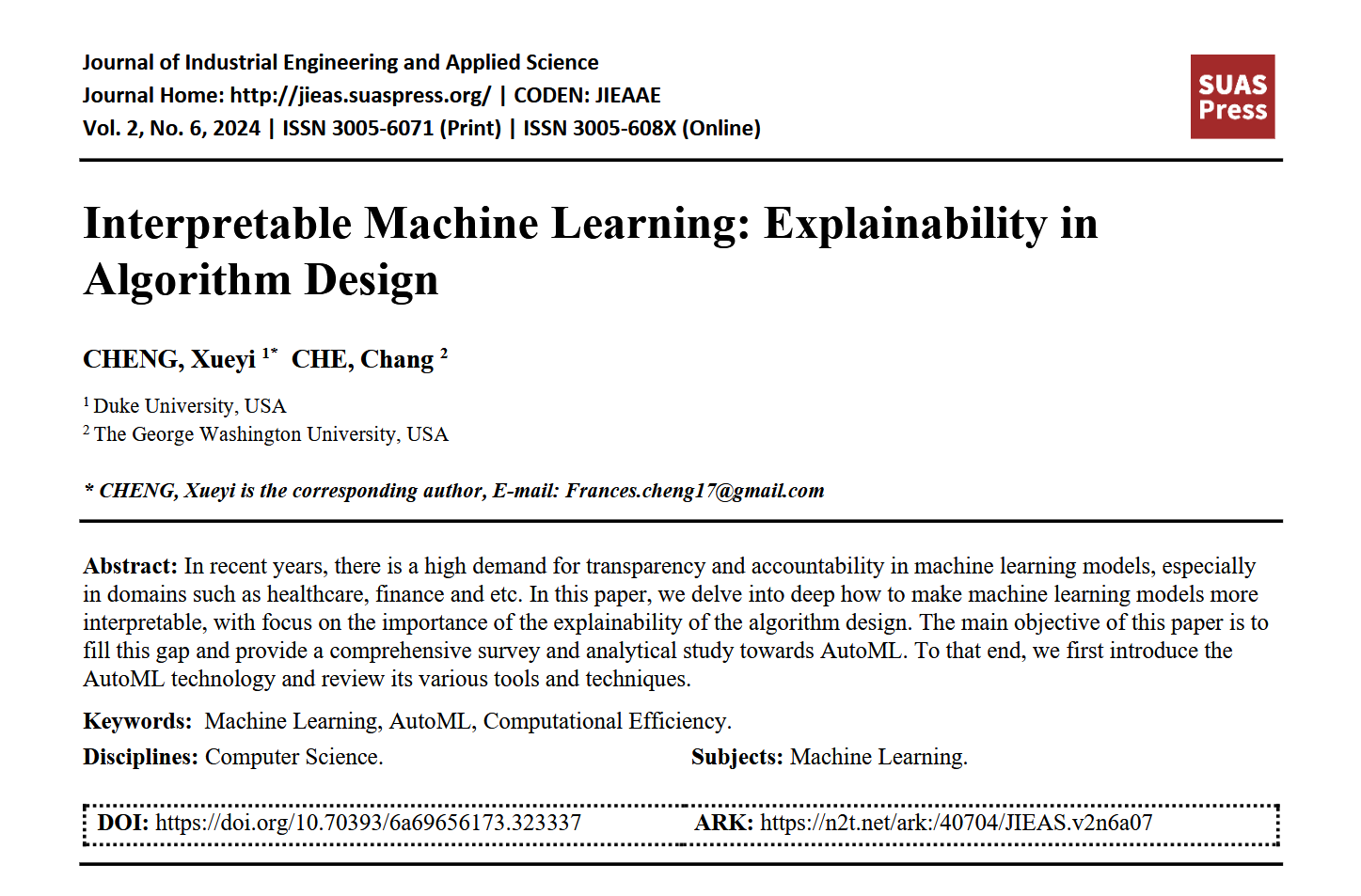
Downloads
Published
How to Cite
Issue
Section
ARK
License
Copyright (c) 2024 The author retains copyright and grants the journal the right of first publication.

This work is licensed under a Creative Commons Attribution 4.0 International License.